Anomaly-guided weakly supervised lesion segmentation on retinal OCT images
Medical Image Analysis(2024)
摘要
The availability of big data can transform the studies in biomedical research to generate greater scientific insights if expert labeling is available to facilitate supervised learning. However, data annotation can be labor-intensive and cost-prohibitive if pixel-level precision is required. Weakly supervised semantic segmentation (WSSS) with image-level labeling has emerged as a promising solution in medical imaging. However, most existing WSSS methods in the medical domain are designed for single-class segmentation per image, overlooking the complexities arising from the co-existence of multiple classes in a single image. Additionally, the multi-class WSSS methods from the natural image domain cannot produce comparable accuracy for medical images, given the challenge of substantial variation in lesion scales and occurrences. To address this issue, we propose a novel anomaly-guided mechanism (AGM) for multi-class segmentation in a single image on retinal optical coherence tomography (OCT) using only image-level labels. AGM leverages the anomaly detection and self-attention approach to integrate weak abnormal signals with global contextual information into the training process. Furthermore, we include an iterative refinement stage to guide the model to focus more on the potential lesions while suppressing less relevant regions. We validate the performance of our model with two public datasets and one challenging private dataset. Experimental results show that our approach achieves a new state-of-the-art performance in WSSS for lesion segmentation on OCT images.
更多查看译文
关键词
Weakly supervised segmentation,Multi-label classification,Retinal OCT lesion segmentation,Anomaly detection,Self-attention
AI 理解论文
溯源树
样例
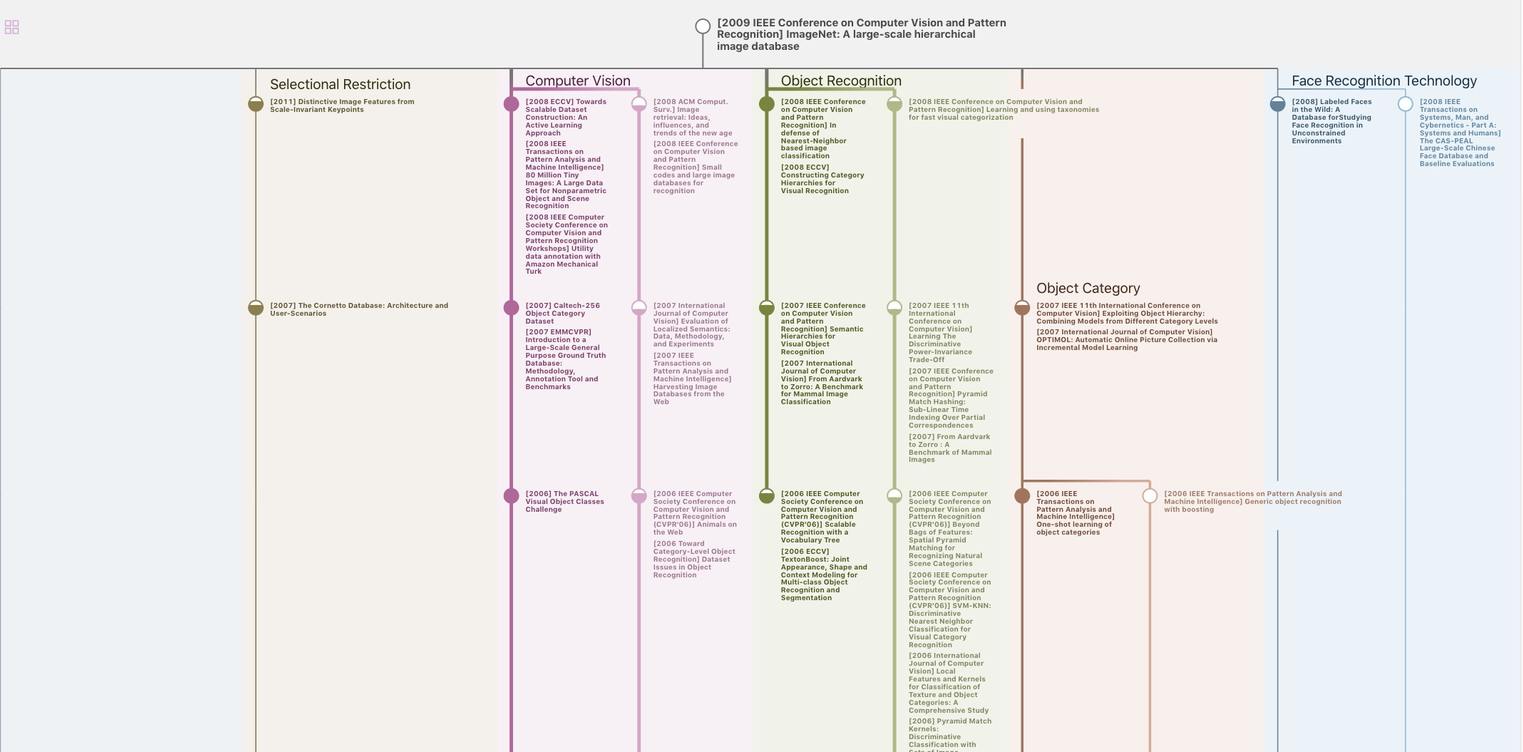
生成溯源树,研究论文发展脉络
Chat Paper
正在生成论文摘要