Machine Learning Improves Prediction of Postoperative Outcomes After Gastrointestinal Surgery: A Systematic Review and Meta-analysis
Journal of Gastrointestinal Surgery(2024)
摘要
Background
Machine learning (ML) approaches have become increasingly popular in predicting surgical outcomes. However, it is unknown whether they are superior to traditional statistical methods such as logistic regression (LR). The aim of this study was to perform a systematic review and meta-analysis to compare the performance of ML versus LR models in predicting postoperative outcomes for patients undergoing gastrointestinal surgery.
Methods
A systematic search of Embase, MEDLINE, Cochrane, Web of Science, and Google Scholar was performed through December 2022. The primary outcome was the discriminatory performance of ML versus LR models as measured by the AUC. A meta-analysis was then performed using a random effects model.
Results
A total of 62 LR models and 143ML models were included across 38 studies. On average, the best performing ML models had a significantly higher AUC than the LR models (ΔAUC 0.07, 95%CI 0.04-0.09; p<0.001). Similarly, on average, the best performing ML models had a significantly higher logit (AUC) than the LR models (Δlogit (AUC) 0.41, 95%CI 0.23-0.58; p<0.001). Nearly half (44%) of studies were found to have a low risk of bias. Upon a subset analysis of only low-risk studies, the difference in logit (AUC) remained significant (ML versus LR, Δlogit (AUC) 0.40, 95%CI 0.14-0.66; p=0.009).
Conclusion
We found a significant improvement in the discriminatory ability when using ML over LR algorithms in predicting postoperative outcomes for patients undergoing gastrointestinal surgery. Subsequent efforts should establish standardized protocols for both developing and reporting studies utilizing ML models and explore the practical implementation of these models.
更多查看译文
关键词
Machine Learning,Logistic Regression,Systematic Review,Meta-analysis,Gastrointestinal Surgery
AI 理解论文
溯源树
样例
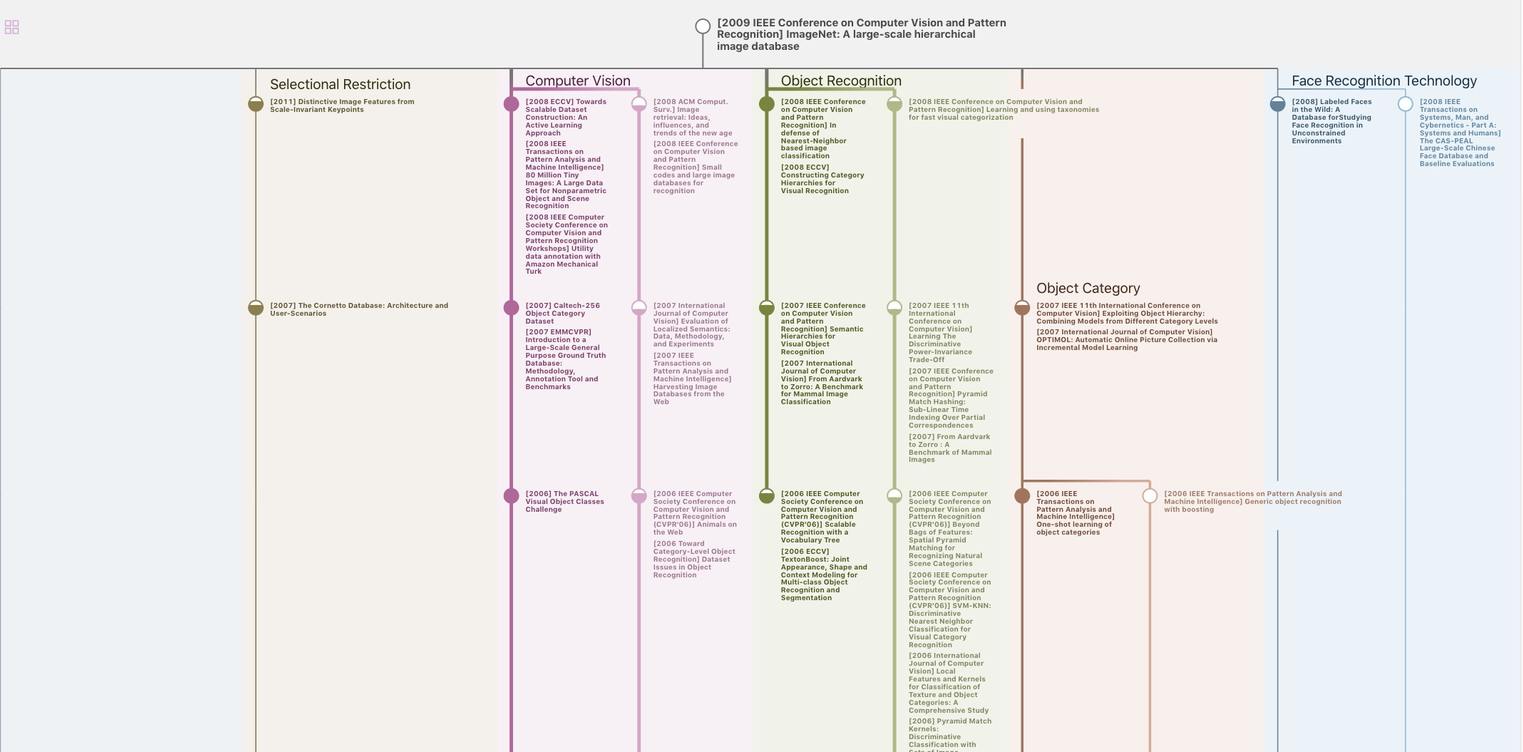
生成溯源树,研究论文发展脉络
Chat Paper
正在生成论文摘要