Integrating satellite remote sensing and Machine Learning techniques for ecological quality assessment in Italian coastal waters
crossref(2024)
摘要
Assessing and monitoring water ecological quality in coastal areas is crucial for safeguarding marine ecosystems, and consequently has a central role in European Union environmental policies. In this work we present the use of in situ monitoring and satellite Earth Observation (EO) techniques as an innovative approach to assess water quality using machine learning. This study shows the use of Sentinel-3 Ocean and Land Color Instrument (OLCI) imagery from Copernicus Marine Service to evaluate the ecological quality of Italian coastal waters, focusing on the Trophic Index (TRIX, Vollenweider et al., 1998), used as a key indicator within the Marine Strategy Directive (2008/56/EC) to characterize the trophic status of coastal waters. Specifially, we investigated the possibility of integrating remote sensing and machine learning techniques to estimate TRIX levels in Italian coastal waters, using an in situ dataset for TRIX estimation obtained from the Marine Strategy Directive Monitoring database (ISPRA). Given that TRIX computation is traditionally based on in situ data, the exploitation of satellite data presents an opportunity to obtain it on a larger spatial and temporal scale. As the TRIX index relies significantly on chlorophyll a, that often exhibits correlations with other components (Massi et al., 2019), we initially explored the relationships between TRIX variables, especially chlorophyll a concentration, and water spectral reflectance using in situ multispectral observations. Encouraged by the results, the effectiveness of OLCI full-resolution data was tested for evaluating the trophic status of Tuscany coastal waters. Our findings initially showed distinctive geographic patterns of in situ TRIX values in the Ligurian, Tyrrhenian, and Ionian coastal regions of Italy, revealing regions with eutrophic conditions near estuaries and others exhibiting oligotrophic characteristics. A Random Forest Regression algorithm was applied to this database, constituted by in situ data and OLCI data, optimizing calibration parameters to predict TRIX levels. This method, via the Feature Importance analysis, underscored the significance of specific spectral bands related to chlorophyll spectral response. The statistical analysis validates the model's performance, indicating in the initial test described above a moderate level of error (MAE of 0.51) and satisfactory explanatory power (R2 of 0.37) (Lapucci et al., 2023). These outcomes show the potentiality of a synergistic use of remote sensing and machine learning in environmental monitoring and management. Current investigations are aimed at refining methodologies and broaden datasets to further test and enhance TRIX monitoring capabilities from a spatial perspective. References: Vollenweider, R.A.; Giovanardi, F.; Montanari, G.; Rinaldi, A. Characterization of the trophic conditions of marine coastal waters with special reference to the NW Adriatic Sea. Environmetrics 1998, 9, 329–357. Massi, L.; Maselli, F.; Rossano, C.; Gambineri, S.; Chatzinikolaou, E.; Dailianis, T.; Arvanitidis, C.; Nuccio, C.; Scapini, F.; Lazzara,L. Reflectance spectra classification for the rapid assessment of water ecological quality in Mediterranean ports. Oceanologia 2019,61, 445–459. Lapucci, C.; Antonini, A.; Böhm, E.; Organelli, E.; Massi, L.; Ortolani, A.; Brandini, C.; Maselli, F. Use of Sentinel-3 OLCI Images and Machine Learning to Assess the Ecological Quality of Italian Coastal Waters. Sensors 2023, 23, 9258.
更多查看译文
AI 理解论文
溯源树
样例
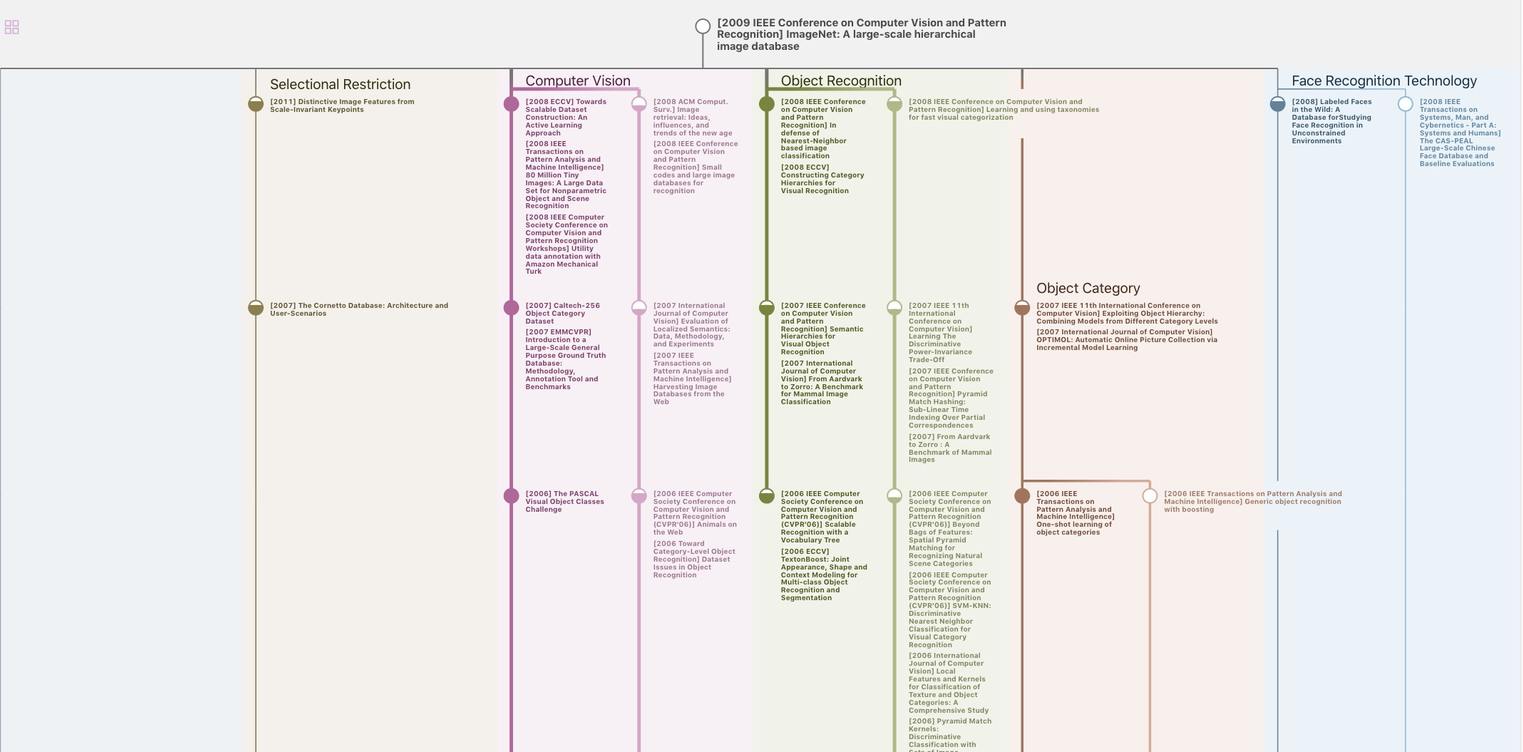
生成溯源树,研究论文发展脉络
Chat Paper
正在生成论文摘要