Evaluating multi-task learning strategies for tropical cyclones itnensity forecasting from satellite images
crossref(2024)
摘要
Skillfully forecasting the evolution of tropical cyclones (TC) is crucial forthe human populations in areas at risk, and an essential indicator of a storm’spotential impact is the Maximum Sustained Wind Speed, often referred to asthe cyclone’s intensity. Predicting the future intensity of ongoing storms istraditionally done using statistical-dynamical methods such as (D)SHIPS andLGEM, or as a byproduct of fully dynamical models such as the HWRF model.Previous works have shown that deep learning models based on convolutionalneural networks can achieve comparable performances using infrared and/orpassive microwave satellite imagery as input. Recently, multi-task models havehighlighted that jointly learning the future intensity and other indicators suchas the TC size with shared network weights can improve the performance in thecontext of intensity estimation. This ongoing work aims to evaluate which tasksand architectures can lead to the best improvement for intensity forecasting.
更多查看译文
AI 理解论文
溯源树
样例
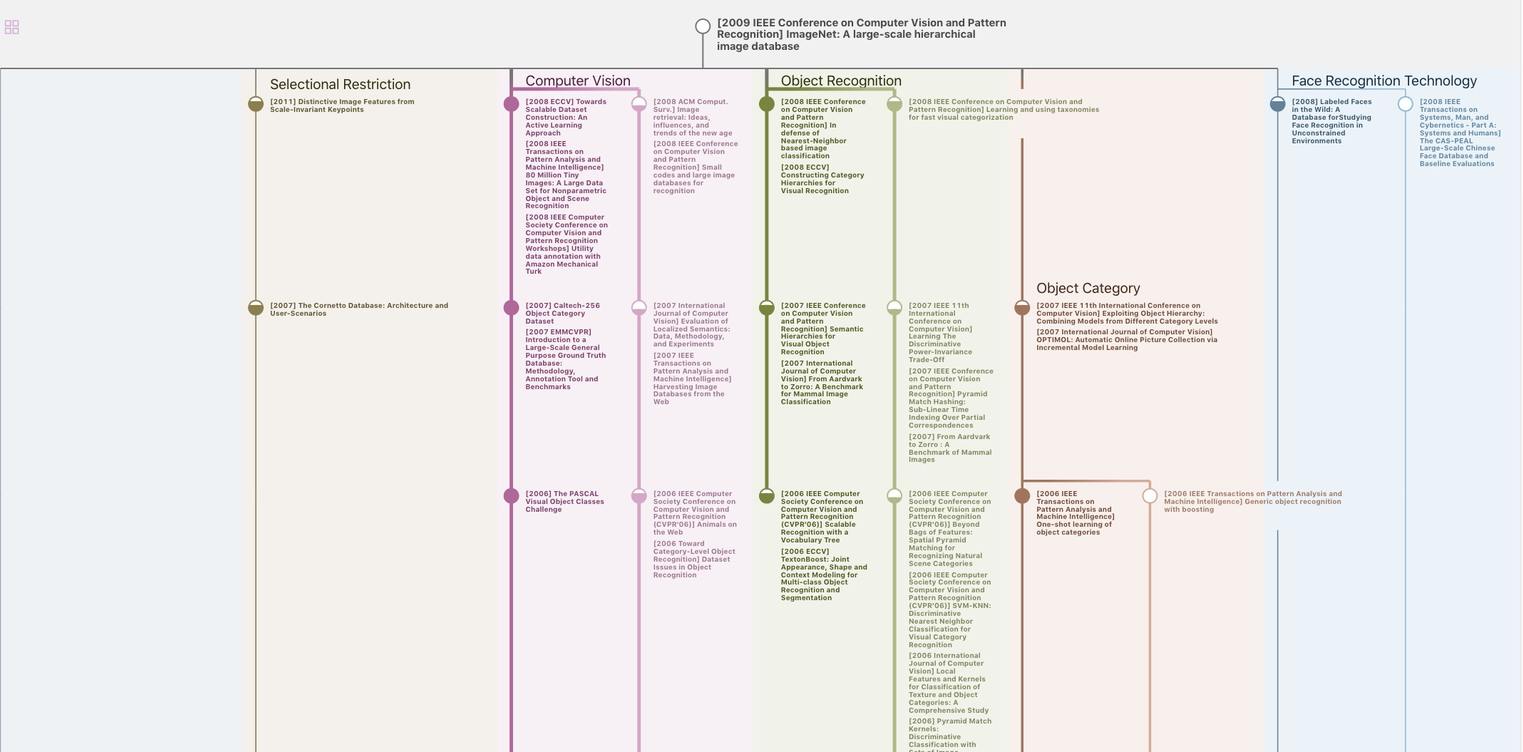
生成溯源树,研究论文发展脉络
Chat Paper
正在生成论文摘要