Long-Term Trends in Senegalese Dryland Tree Density
crossref(2024)
摘要
The historical extent and distribution of trees is central to our understanding of the current and future dynamics within the terrestrial biosphere. Typically, trees outside of forests, that characterise drylands, are often left out of coarse-grained analyses as they cannot be reasonably detected. However, 29% of tree cover within drylands is found outside of areas previously classified as forest. The rapid advancement of remote sensing technology over the last 50 years has seen a paralleled increase in both computational capacity and algorithmic sophistication. However, it is challenging to utilise older data sources with long-term image coverage and a coarser pixel resolution than the phenomenon being observed. There has been little research exploring the potential for modern data science methods, such as deep learning, for extracting more information from older data sources. Here, we propose a method for creating long-term tree density maps at the 15-meter scale, based on sub-meter resolution tree information. By utilising high resolution imagery to automatically detect and count individual trees, we have produced a deep learning-based method to predict tree density from coarser satellite imagery for Senegal over the past 25 years. Validation of our predictions will be conducted against on-site measurements from national forest inventory sites, facilitating an evaluation of high-resolution trends in dryland woody vegetation across time. The intricate and nonlinear nature inherent in neural networks renders them adept at managing noise and discontinuities within data sources. Here, we show that common artifacts produced by gap filling which often result from cloud cover and sensor error, can be minimised with the use of a deep learning framework. Such an analysis unlocks the possibility to extract less noisy and more continuous information from poorer image sources.
更多查看译文
AI 理解论文
溯源树
样例
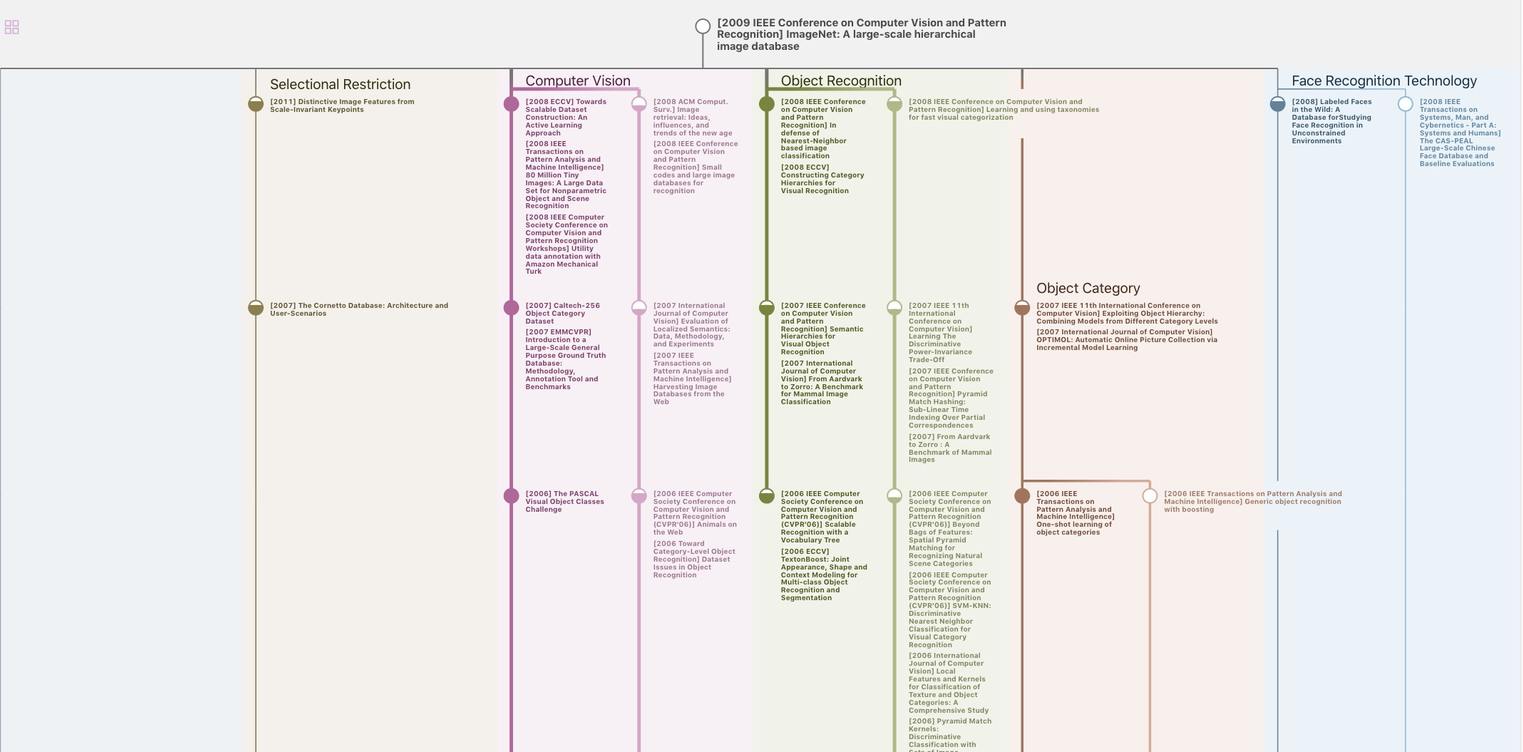
生成溯源树,研究论文发展脉络
Chat Paper
正在生成论文摘要