Latent Semantic Consensus for Deterministic Geometric Model Fitting.
IEEE transactions on pattern analysis and machine intelligence(2024)
摘要
Estimating reliable geometric model parameters from the data with severe outliers is a fundamental and important task in computer vision. This paper attempts to sample high-quality subsets and select model instances to estimate parameters in the multi-structural data. To address this, we propose an effective method called Latent Semantic Consensus (LSC). The principle of LSC is to preserve the latent semantic consensus in both data points and model hypotheses. Specifically, LSC formulates the model fitting problem into two latent semantic spaces based on data points and model hypotheses, respectively. Then, LSC explores the distributions of points in the two latent semantic spaces, to remove outliers, generate high-quality model hypotheses, and effectively estimate model instances. Finally, LSC is able to provide consistent and reliable solutions within only a few milliseconds for general multi-structural model fitting, due to its deterministic fitting nature and efficiency. Compared with several state-of-the-art model fitting methods, our LSC achieves significant superiority for the performance of both accuracy and speed on synthetic data and real images. The code will be available at https://github.com/guobaoxiao/LSC.
更多查看译文
关键词
Deterministic fitting,latent semantic,model fitting,multiple-structure data
AI 理解论文
溯源树
样例
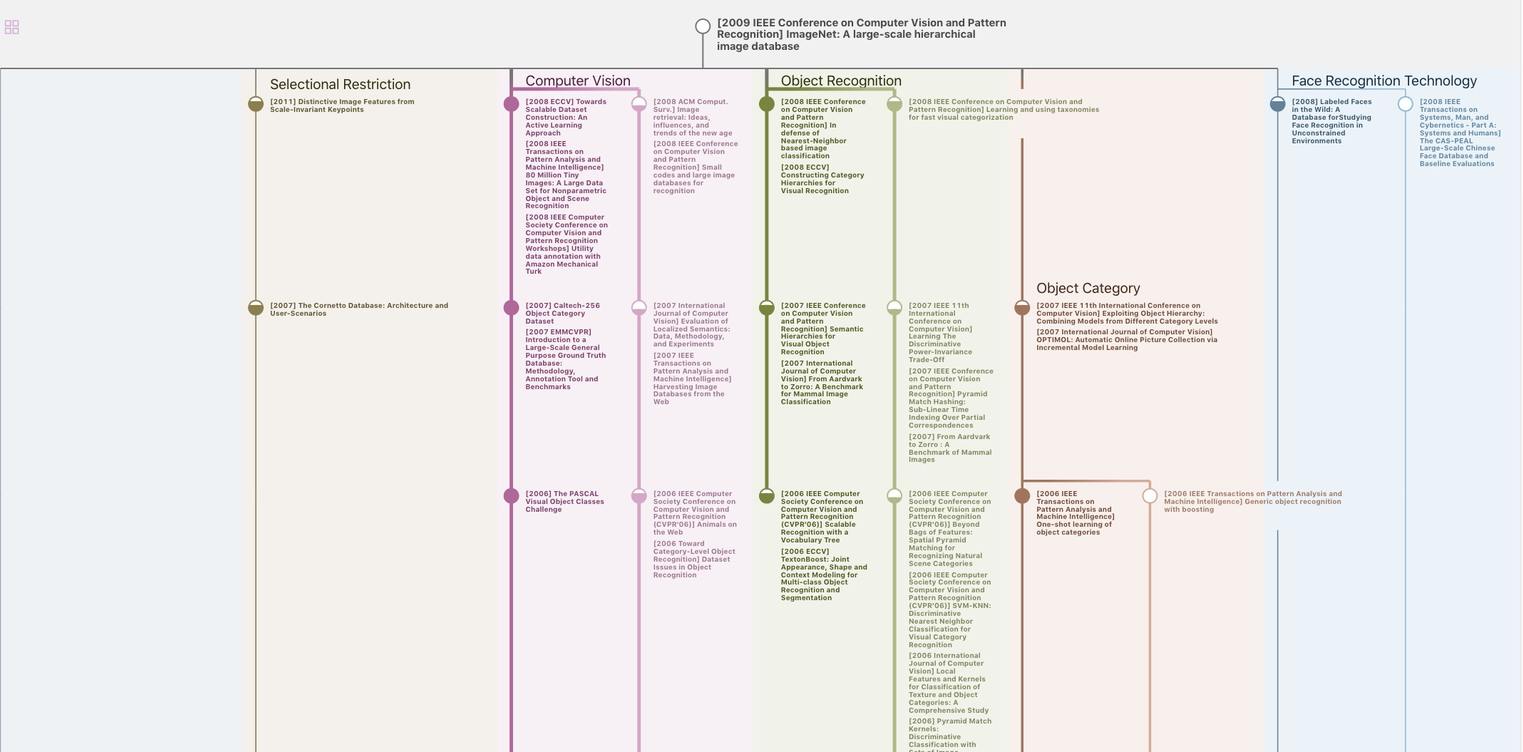
生成溯源树,研究论文发展脉络
Chat Paper
正在生成论文摘要