Decomposing Vision-based LLM Predictions for Auto-Evaluation with GPT-4
arxiv(2024)
摘要
The volume of CT exams being done in the world has been rising every year,
which has led to radiologist burn-out. Large Language Models (LLMs) have the
potential to reduce their burden, but their adoption in the clinic depends on
radiologist trust, and easy evaluation of generated content. Presently, many
automated methods are available to evaluate the reports generated for chest
radiographs, but such an approach is not available for CT presently. In this
paper, we propose a novel evaluation framework to judge the capabilities of
vision-language LLMs in generating accurate summaries of CT-based
abnormalities. CT slices containing an abnormality (e.g., lesion) were input to
a vision-based LLM (GPT-4V, LLaVA-Med, and RadFM), and it generated a free-text
summary of the predicted characteristics of the abnormality. Next, a GPT-4
model decomposed the summary into specific aspects (body part, location, type,
and attributes), automatically evaluated the characteristics against the
ground-truth, and generated a score for each aspect based on its clinical
relevance and factual accuracy. These scores were then contrasted against those
obtained from a clinician, and a high correlation ( 85
observed. Although GPT-4V outperformed other models in our evaluation, it still
requires overall improvement. Our evaluation method offers valuable insights
into the specific areas that need the most enhancement, guiding future
development in this field.
更多查看译文
AI 理解论文
溯源树
样例
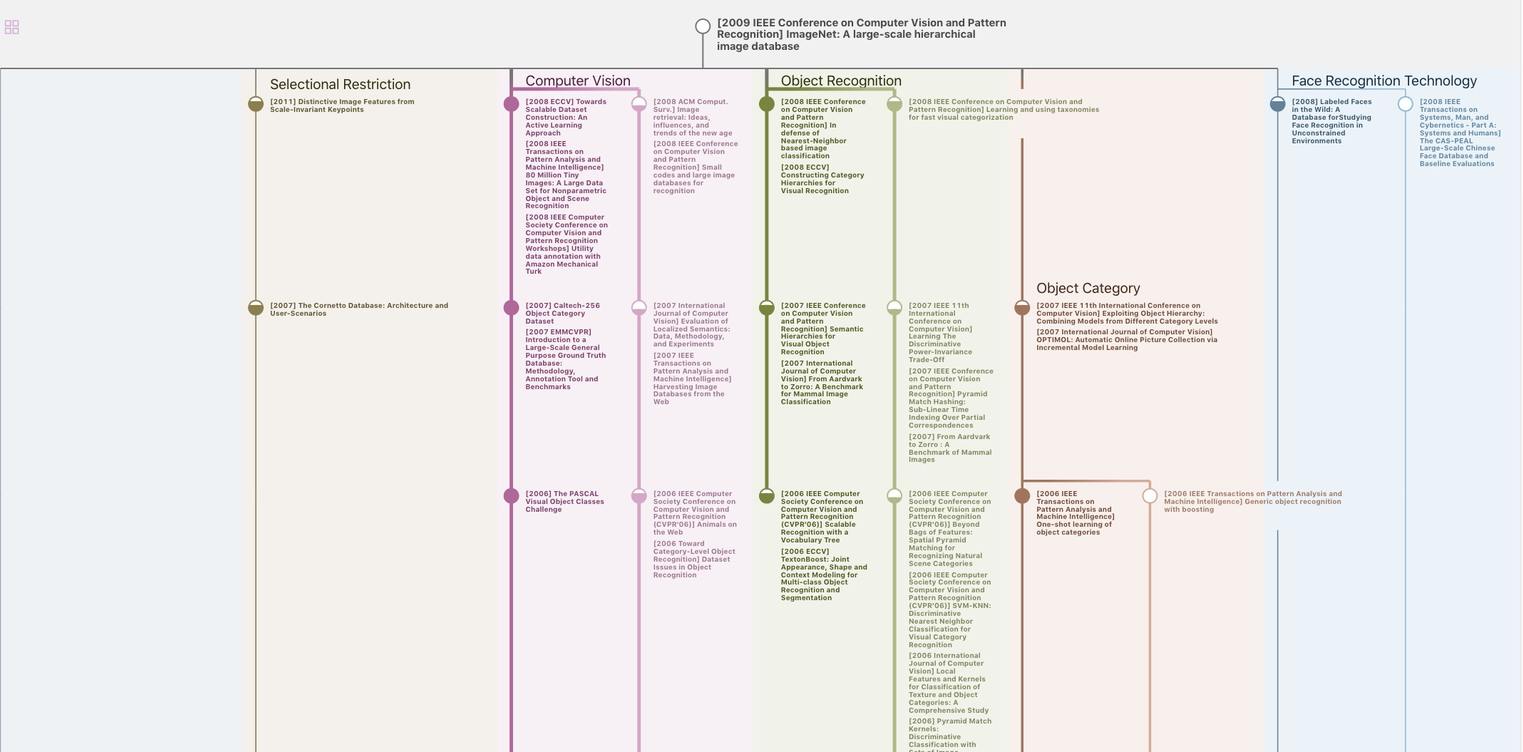
生成溯源树,研究论文发展脉络
Chat Paper
正在生成论文摘要