Comparison of Domain Selection Methods for Multi-Source Manifold Feature Transfer Learning in Electroencephalogram Classification
APPLIED SCIENCES-BASEL(2024)
摘要
Transfer learning (TL) utilizes knowledge from the source domain (SD) to enhance the classification rate in the target domain (TD). It has been widely used to address the challenge of sessional and inter-subject variations in electroencephalogram (EEG)-based brain-computer interfaces (BCIs). However, utilizing knowledge from a combination of both related and non-related sources can significantly deteriorate the classification performance across individual target domains, resulting in a negative transfer (NT). Hence, NT becomes one of the most significant challenges for transfer learning algorithms. Notably, domain selection techniques have been developed to address the challenge of NT emerging from the transfer of knowledge from non-related sources. However, existing domain selection approaches iterate through domains and remove a single low-beneficial domain at a time, which can massively affect the classification performance in each iteration since SDs respond differently to other sources. In this paper, we compare domain selection techniques for a multi-source manifold feature transfer learning (MMFT) framework to address the challenge of NT and then evaluate the effect of beneficial and non-beneficial sources on TL performance. To evaluate the effect of low-beneficial and high beneficial sources on TL performance, some commonly used domain selection methods are compared, namely, domain transferability estimation (DTE), rank of domain (ROD), label similarity analysis, and enhanced multi-class MMFT (EMC-MMFT), using the same multi-class cross-session and cross-subject classification problems. The experimental results demonstrate the superiority of the EMC-MMFT algorithm in terms of minimizing the effect of NT. The highest classification accuracy (CA) of 100% is achieved when optimal combinations of high beneficial sources are selected for two-class SSMVEP problems, while the highest CAs of 98% and 87% are achieved for three- and four-class SSMVEP problems, respectively. The highest CA of 98% is achieved for two-class MI classification problems, while the highest CAs of 90% and 71.5% are obtained for three- and four-class MI problems, respectively.
更多查看译文
关键词
brain-computer interface (BCI),electroencephalogram (EEG),transfer learning (TL),negative transfer (NT),multi-source manifold feature transfer learning (MMFT),enhanced multi-class MMFT (EMC-MMFT)
AI 理解论文
溯源树
样例
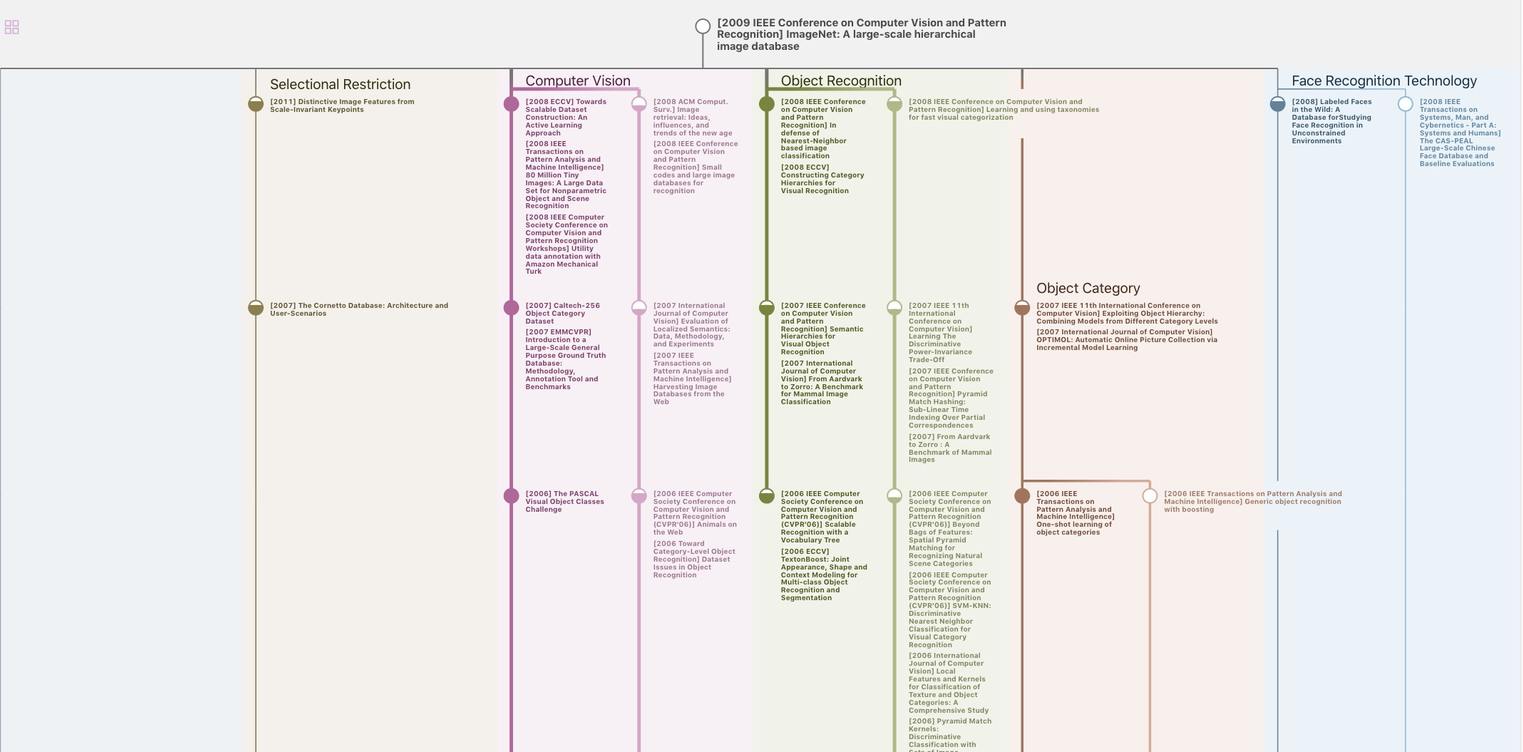
生成溯源树,研究论文发展脉络
Chat Paper
正在生成论文摘要