Mapping 3-D classroom seats based on partial object point cloud completion
CARTOGRAPHY AND GEOGRAPHIC INFORMATION SCIENCE(2024)
摘要
3-D classroom seating information is of great significance in a host of planning contexts including indoor navigation and facility management. Mapping classroom 3-D seats based on a single-view LiDAR scan is challenging because of occlusions and viewpoint limitations. In essence, one is left with incomplete point clouds, making object recognition difficult. The aim of this paper is to acquire complete point clouds for objects in a room based on a single-view LiDAR scan, focusing in particular on chairs in indoor spaces such as classrooms, lecture halls, theaters, concert venues, and sports arenas. A spatial optimization enabled framework is proposed for 3-D classroom seat mapping from a single-view LiDAR scan. A two-step clustering method is proposed to segment each individual chair object in the LiDAR scan. A chair model is extracted and used to complete other chair point clouds via registration. Notably, a parameter estimation method is devised based on spatial priors and integrated in an iterative closest point optimization approach to refine registration. Applications to multiple classroom scans demonstrate the effectiveness and accuracy of the proposed methodology. The developed segmentation-registration framework harnesses spatial knowledge to complete a single-view LiDAR scan with many identical objects. The resultant object point cloud is critical for navigation, route guidance, facility management, pandemic response and planning, indoor mapping and venue utilization, among others, which require precise and complete spatial information. Identified 3-D chairs extracted from LiDAR scans provide essential information for stakeholders seating configuration, property management, and indoor navigation.A new and challenging problem of completing partial objects from a single-view LiDAR scan is formulated.The developed framework provides a fast and high-precision solution to process single-view LiDAR scans for extracting and restoring incomplete objects by combining spatial knowledge, machine learning, and spatial optimization.Empirical results demonstrate the capability to acquire complete chair point clouds from multiple classroom scans to support planning and policy efforts in mitigating disease spread.
更多查看译文
关键词
LiDAR,point cloud completion,point cloud registration,spatial optimization,machine learning,clustering,indoor mapping
AI 理解论文
溯源树
样例
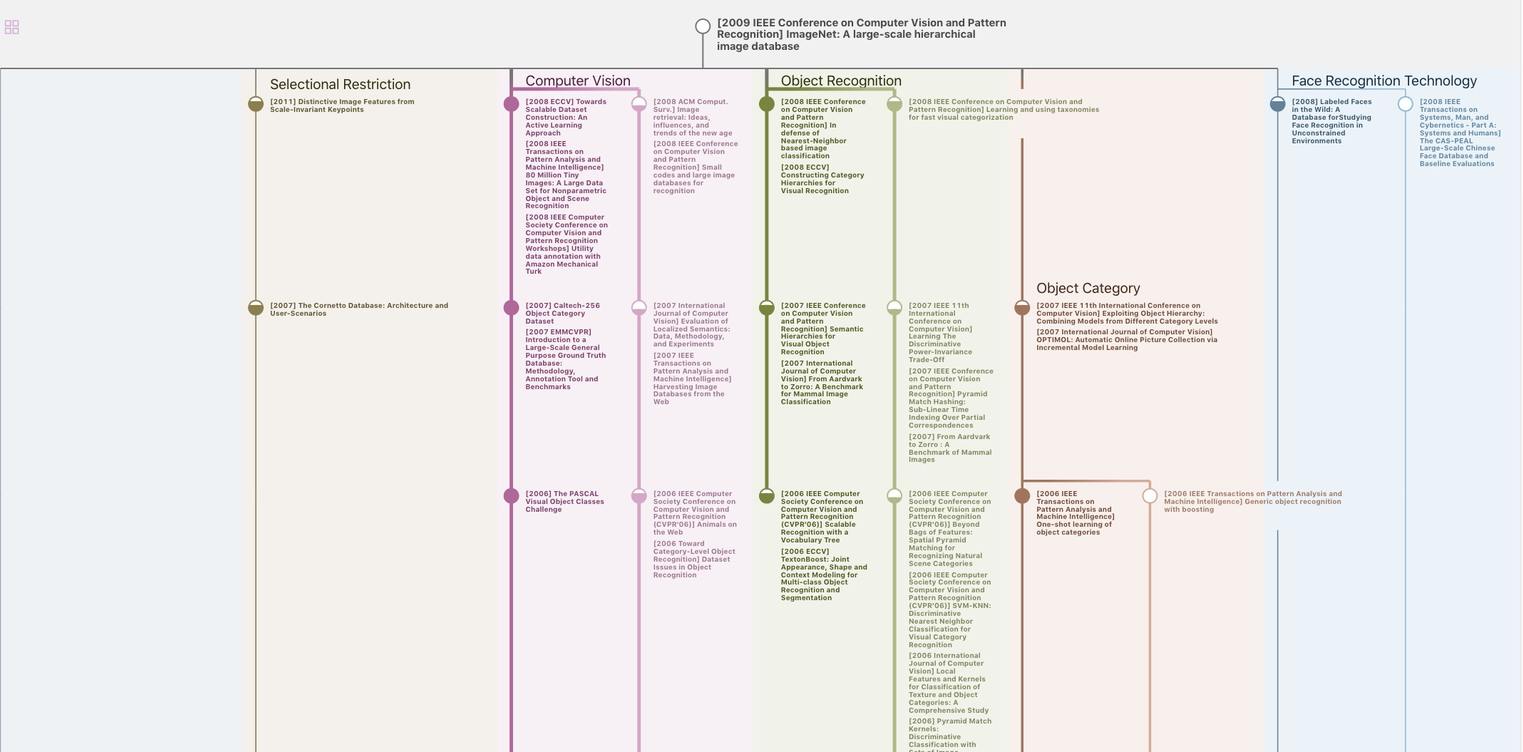
生成溯源树,研究论文发展脉络
Chat Paper
正在生成论文摘要