Leveraging Continuous Time to Understand Momentum When Training Diagonal Linear Networks
arxiv(2024)
摘要
In this work, we investigate the effect of momentum on the optimisation
trajectory of gradient descent. We leverage a continuous-time approach in the
analysis of momentum gradient descent with step size γ and momentum
parameter β that allows us to identify an intrinsic quantity λ =
γ/ (1 - β)^2 which uniquely defines the optimisation path
and provides a simple acceleration rule. When training a 2-layer diagonal
linear network in an overparametrised regression setting, we characterise the
recovered solution through an implicit regularisation problem. We then prove
that small values of λ help to recover sparse solutions. Finally, we
give similar but weaker results for stochastic momentum gradient descent. We
provide numerical experiments which support our claims.
更多查看译文
AI 理解论文
溯源树
样例
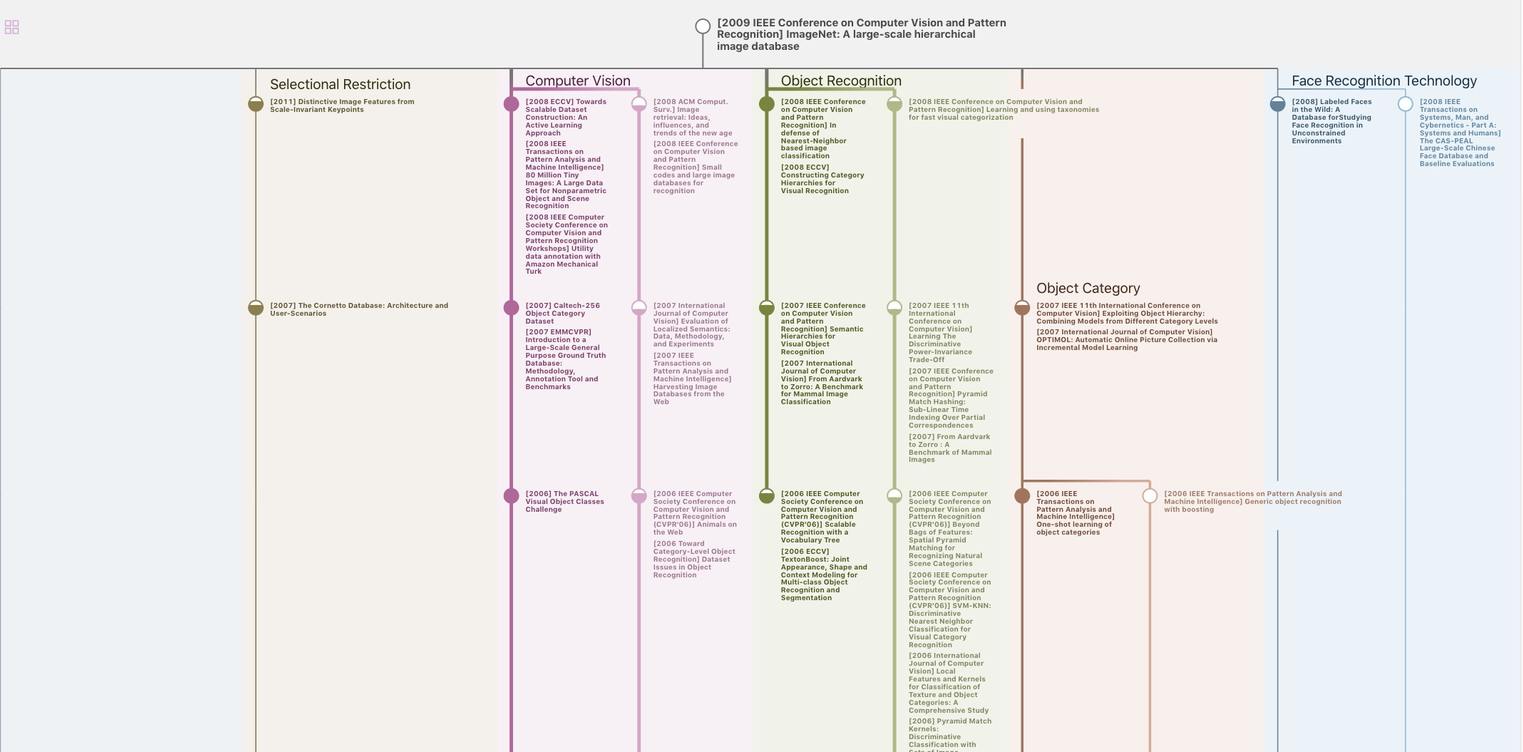
生成溯源树,研究论文发展脉络
Chat Paper
正在生成论文摘要