Improved Lower Bound for Differentially Private Facility Location
arxiv(2024)
摘要
We consider the differentially private (DP) facility location problem in the
so called super-set output setting proposed by Gupta et al. [SODA 2010]. The
current best known expected approximation ratio for an ϵ-DP algorithm
is O(log n/√(ϵ)) due to Cohen-Addad et al.
[AISTATS 2022] where n denote the size of the metric space, meanwhile the
best known lower bound is Ω(1/√(ϵ)) [NeurIPS 2019].
In this short note, we give a lower bound of
Ω̃(min{log n, √(log
n/ϵ)}) on the expected approximation ratio of any
ϵ-DP algorithm, which is the first evidence that the approximation
ratio has to grow with the size of the metric space.
更多查看译文
AI 理解论文
溯源树
样例
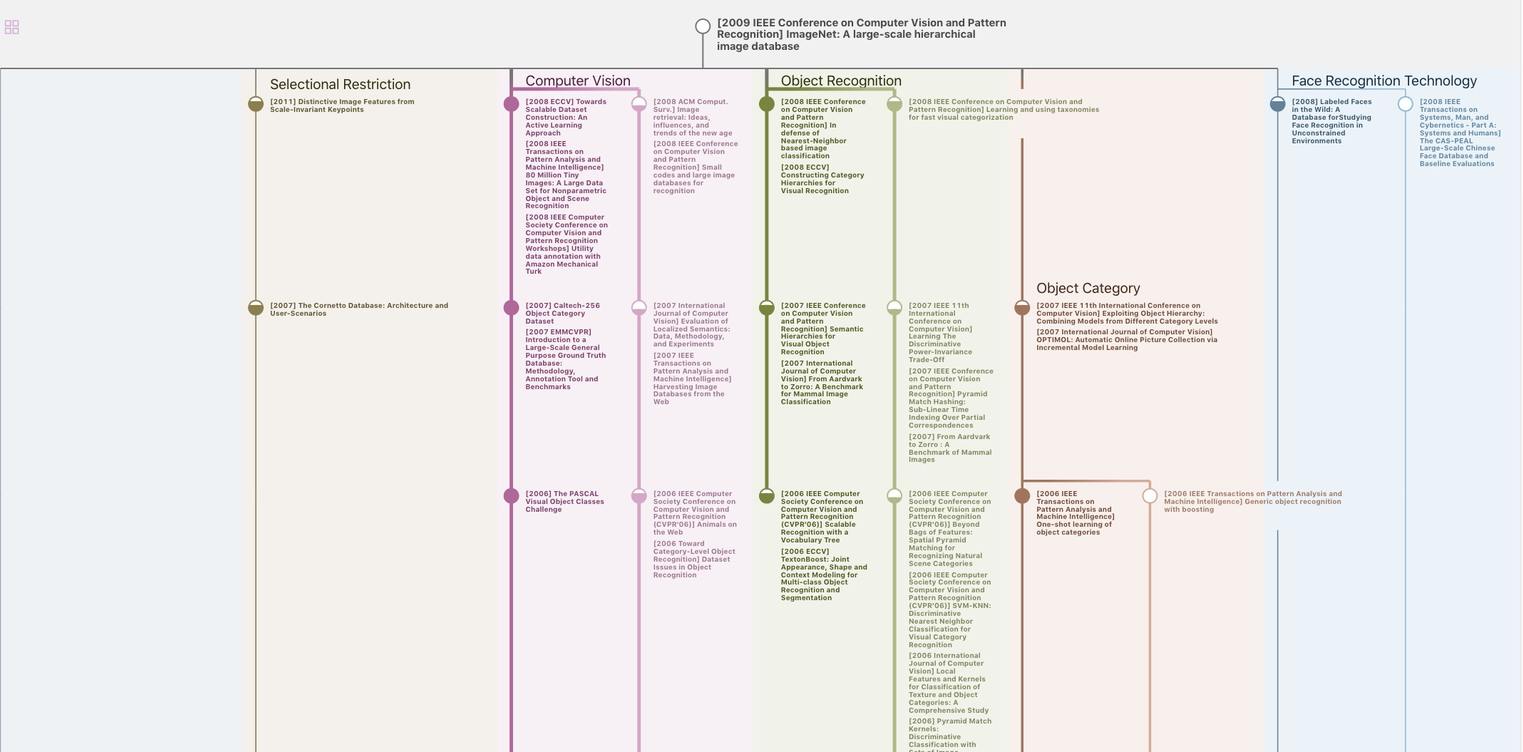
生成溯源树,研究论文发展脉络
Chat Paper
正在生成论文摘要