Group Privacy Amplification and Unified Amplification by Subsampling for Rényi Differential Privacy
arxiv(2024)
摘要
Differential privacy (DP) has various desirable properties, such as
robustness to post-processing, group privacy, and amplification by subsampling,
which can be derived independently of each other. Our goal is to determine
whether stronger privacy guarantees can be obtained by considering multiple of
these properties jointly. To this end, we focus on the combination of group
privacy and amplification by subsampling. To provide guarantees that are
amenable to machine learning algorithms, we conduct our analysis in the
framework of Rényi-DP, which has more favorable composition properties than
(ϵ,δ)-DP. As part of this analysis, we develop a unified
framework for deriving amplification by subsampling guarantees for Rényi-DP,
which represents the first such framework for a privacy accounting method and
is of independent interest. We find that it not only lets us improve upon and
generalize existing amplification results for Rényi-DP, but also derive
provably tight group privacy amplification guarantees stronger than existing
principles. These results establish the joint study of different DP properties
as a promising research direction.
更多查看译文
AI 理解论文
溯源树
样例
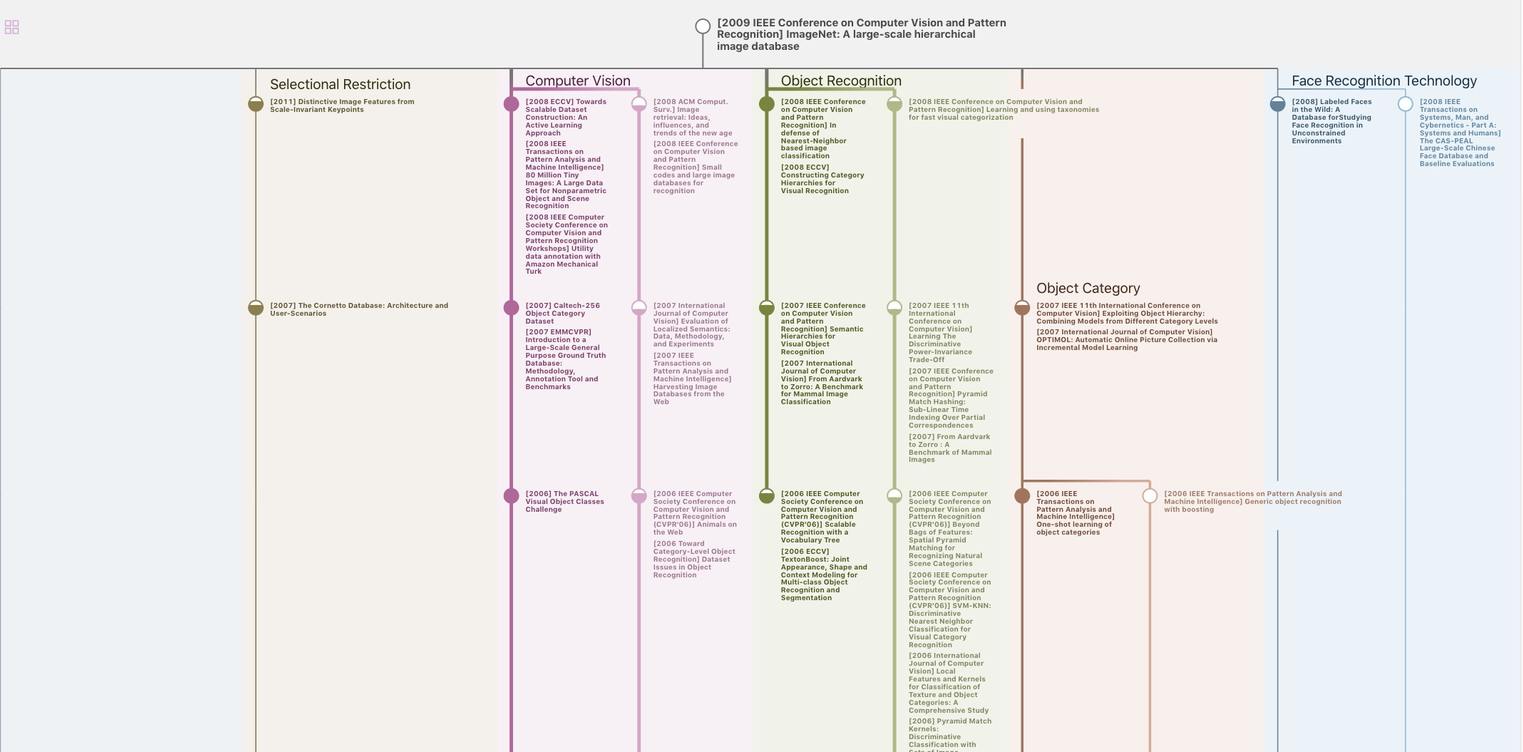
生成溯源树,研究论文发展脉络
Chat Paper
正在生成论文摘要