Storm surge modeling in the AI era: Using LSTM-based machine learning for enhancing forecasting accuracy
Coastal Engineering(2024)
摘要
Physics simulation results of natural processes usually do not fully capture the real world. This is caused for instance by limits in what physical processes are simulated and to what accuracy. In this work we propose and analyze the use of an LSTM-based deep learning network machine learning (ML) architecture for capturing and predicting the behavior of the systemic error for storm tide forecast models with respect to real-world water elevation observations from gauge stations during hurricane events. The overall goal of this work is to predict the systemic error of the physics model and use it to improve the accuracy of the simulation results post factum (i.e., to correct the model bias). We trained our proposed ML model on a dataset of 61 historical storms in the coastal regions of the south and southeastern U.S. and we tested its performance in bias correcting modeled water level data predictions from Hurricane Ian (2022). We show that our model can consistently improve the forecasting accuracy for Hurricane Ian — unknown to the ML model — at the majority of gauge station coordinates. Moreover, by examining the impact of using different subsets of the initial training dataset, containing a number of relatively similar or different hurricanes in terms of hurricane track, we found that we can obtain similar quality of bias correction by only using a subset of six hurricanes. This is an important result that implies the possibility to apply a pre-trained ML model to real-time hurricane forecasting results with the goal of bias correcting and improving the forecast accuracy. The presented work is an important first step in creating a bias correction system for real-time storm tide forecasting applicable to the full simulation area. It also presents a highly transferable and operationally applicable methodology for improving the accuracy in a wide range of physics simulation scenarios beyond storm tide forecasting.
更多查看译文
关键词
Long-Short Term Memory (LSTM) networks,Machine learning,Storm surge modeling,Bias correction
AI 理解论文
溯源树
样例
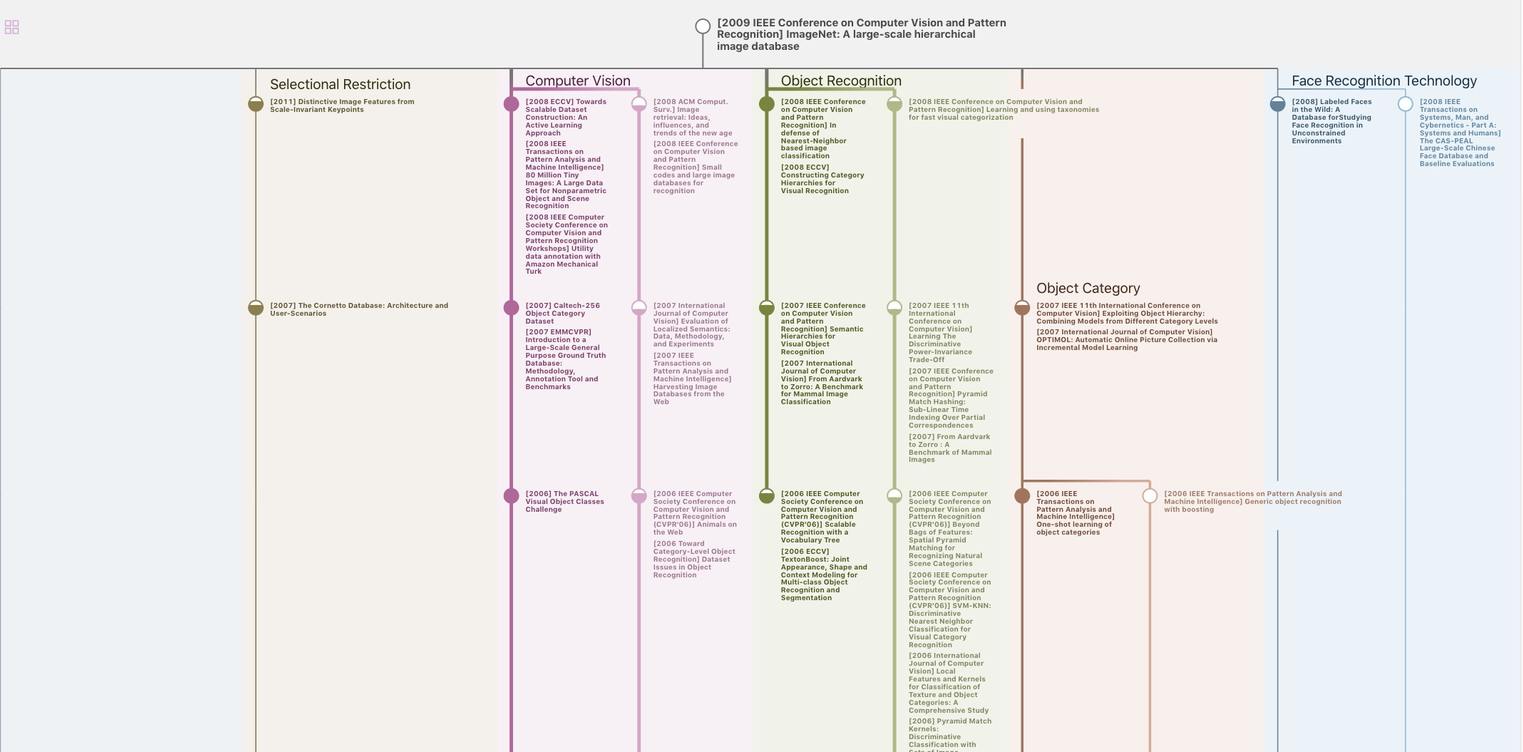
生成溯源树,研究论文发展脉络
Chat Paper
正在生成论文摘要