Integrating machine learning technique with SWAT model to optimize the prediction of peak flow in the Beas River basin, India
crossref(2024)
摘要
Accurately predicting and simulating runoff is more significant in Beas River Basin, due to the uncertainty that posed by the climate change. This uncertainty brought challenges and difficulties to efficiently manage the water resources. Beas river basin is prone to flash flood area, hence estimating the peak flow is critical in this basin. Due to the presence of dams and reservoirs in the basin, they pose difficulties in predicting peak flow and daily streamflow. In this study, for modelling streamflow physics-based machine learning (ML) model in combination with semi-distributed process-based SWAT hydrological model have been evaluated. In this study performance of Elastic Net (EN) ML technique have been compared with calibrated-SWAT (cSWAT) model. The root mean square error (RMSE), coefficient of determination (R2) and Nash–Sutcliffe efficiency (NSE) were used to evaluate the performance of all the models. The findings of the study revealed that the in comparison to cSWAT model, ML model performed better. However, when ML regression models are paired with uncalibrated SWAT (uSWAT-ML) few models outperformed cSWAT model. The R2, RMSE and NSE value of cSWAT model is 0.73, 276.92 m3/s, and 0.72 which shows good performance. EN obtained better performance with R2, RMSE and NSE value of 0.89, 0.87 and 158.31 m3 respectively. Hence the approached uSWAT-ML have been used to predict the peak flow values, with models EN got better results with R2 value of 0.71. The results indicates that the integrating ML model with uncalibrated SWAT model yields reliable results for predicting daily streamflow and peak flow values.
更多查看译文
AI 理解论文
溯源树
样例
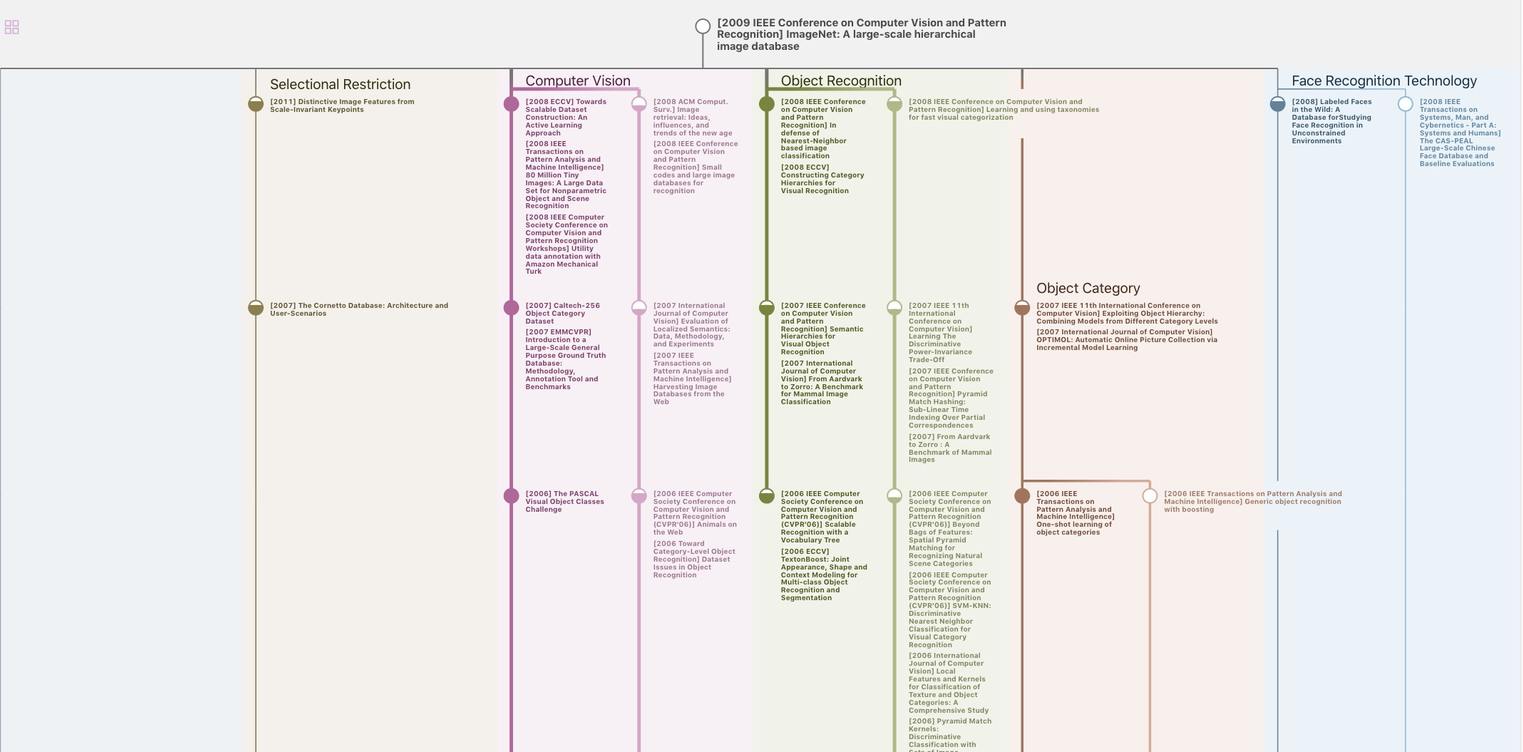
生成溯源树,研究论文发展脉络
Chat Paper
正在生成论文摘要