Filtering and regret network for spacecraft component segmentation based on gray images and depth maps
Chinese Journal of Aeronautics(2024)
摘要
Identifying and segmenting spacecraft components is vital in many on-orbit space missions, such as on-orbit maintenance and component recovery. Integrating depth maps with visual images has been proven effective in improving segmentation accuracy. However, existing methods ignore the noise and fallacy in collected depth maps, which interfere with the network to extract representative features, decreasing the final segmentation accuracy. To this end, this paper proposes a Filtering and Regret Network (FRNet) for spacecraft component segmentation. The FRNet incorporates filtering and regret mechanisms to suppress the abnormal depth response in shallow layers and selectively reuses the filtered cues in deep layers, avoiding the detrimental effects of low-quality depth information while preserving the semantic context inherent in depth maps. Furthermore, a two-stage feature fusion module is proposed, which involves information interaction and aggregation. This module effectively explores the feature correlation and unifies the multi-modal features into a comprehensive representation. Finally, a large-scale spacecraft component recognition dataset is constructed for training and evaluating spacecraft component segmentation algorithms. Experimental results demonstrate that the FRNet achieves a state-of-the-art performance with a mean Intersection Over Union (mIOU) of 84.13% and an average inference time of 133.2 ms when tested on an NVIDIA RTX 2080 SUPER GPU.
更多查看译文
关键词
spacecraft component recognition,multi-modal feature fusion,satellite dataset,intelligent systems,deep learning
AI 理解论文
溯源树
样例
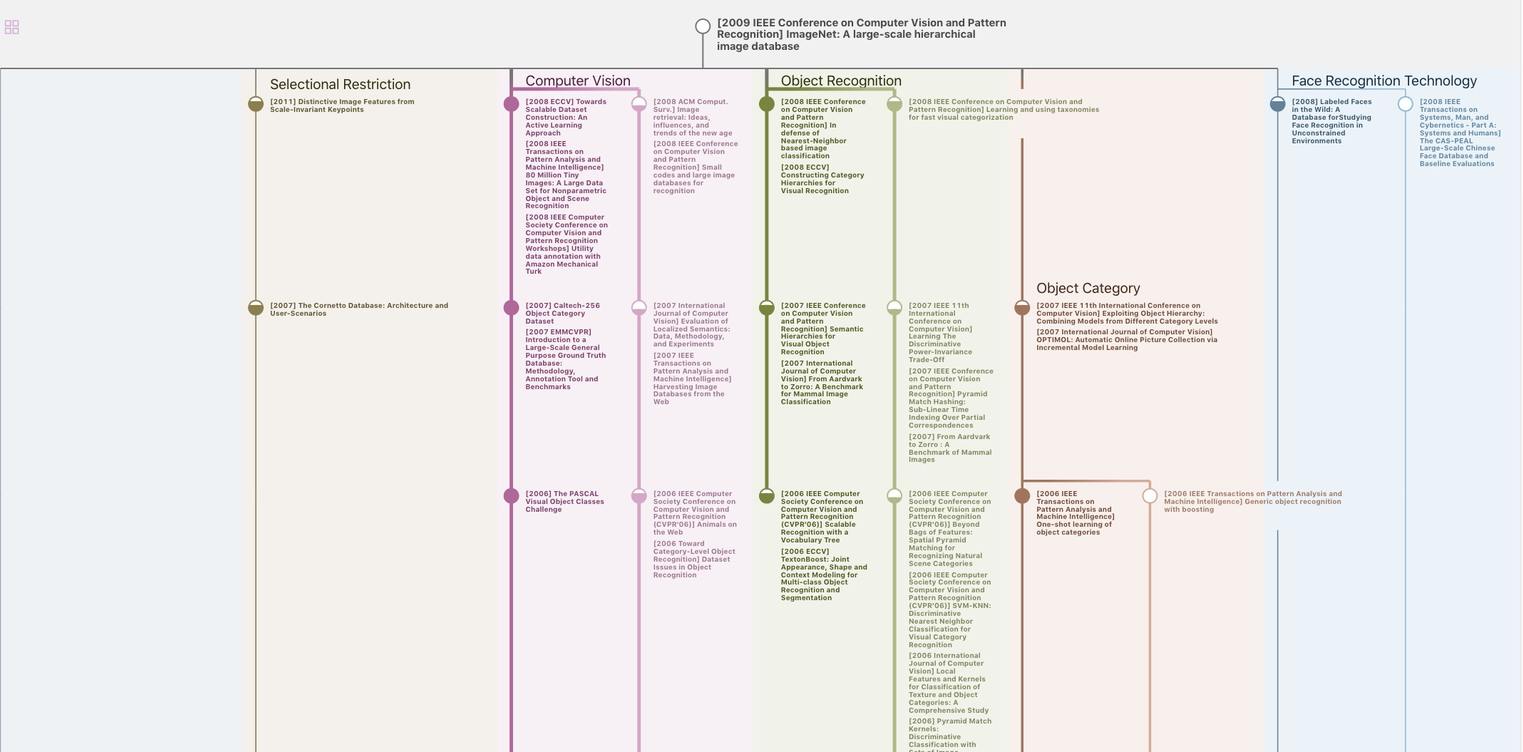
生成溯源树,研究论文发展脉络
Chat Paper
正在生成论文摘要