An efficient deep recurrent neural network for detection of cyberattacks in realistic IoT environment
The Journal of Supercomputing(2024)
摘要
The rapid growth of Internet of Things (IoT) devices has changed human interactions with the environment. IoT networks require specialized defense strategies distinct from traditional corporate contexts. Security measures such as anti-malware software, firewalls, authentication protocols, and encryption techniques are established but face limitations against evolving attack strategies. Therefore, this study proposes an intrusion detection approach for a realistic IoT environment, employing various variants of deep learning models such as deep neural networks (DNNs), convolutional neural networks (CNNs), and recurrent neural networks (RNNs). The research tested three variants for each model: DNN1, DNN2, DNN3, CNN1, CNN2, CNN3, RNN1, RNN2, RNN3. All these variants are customized and tuned differently to analyze the efficacy of the suggested methodology. Likewise, notable observation highlights the significance of aligning training and validation accuracy curves that indicate controlled overfitting, validating the model’s reliability in accurately predicting intrusion and benign network traffic in IoT settings. Results reveal that RNN1 achieves the best results: accuracy of 98.61
更多查看译文
关键词
Internet of Things (IoT),Cybersecurity,IoT security,Security attacks,Deep learning,Deep learning variants
AI 理解论文
溯源树
样例
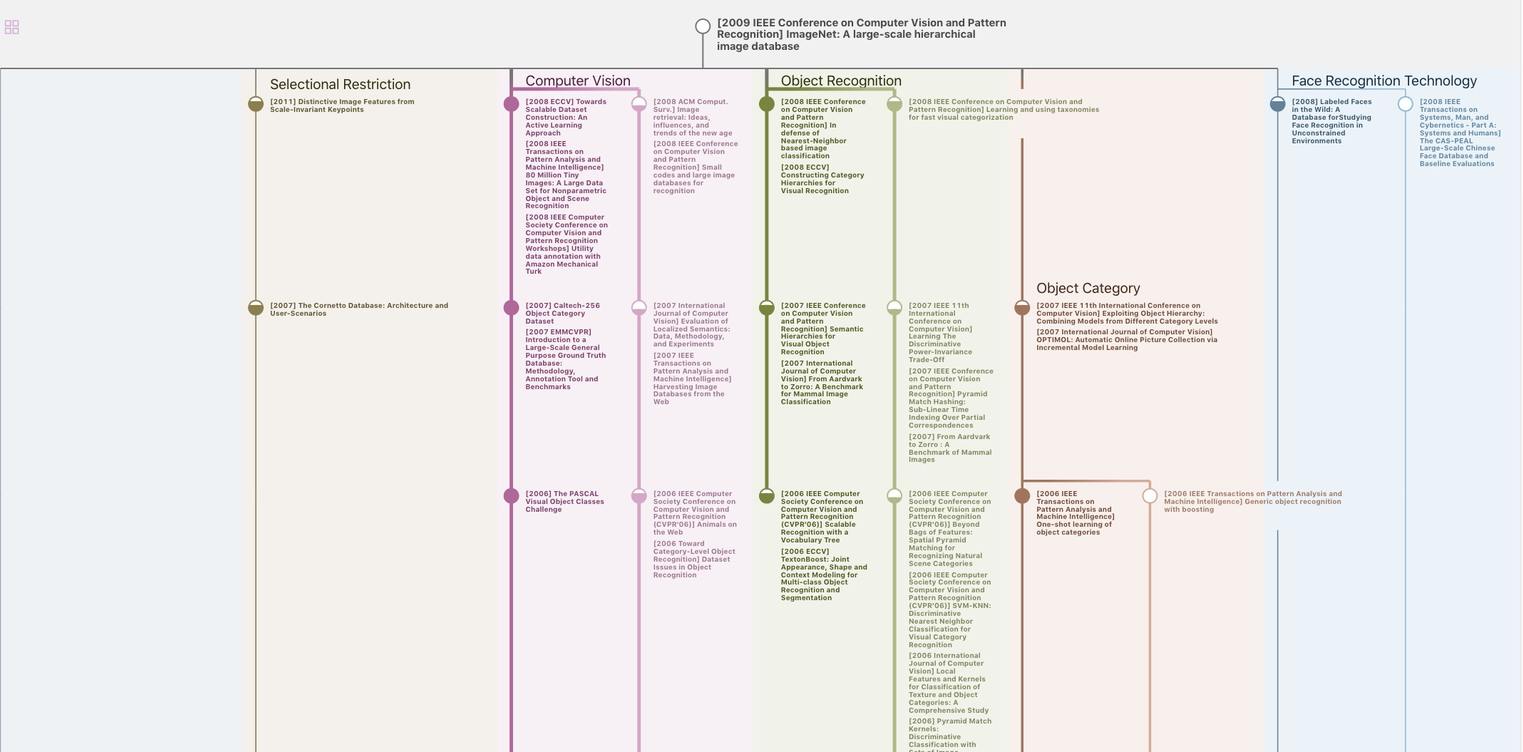
生成溯源树,研究论文发展脉络
Chat Paper
正在生成论文摘要