Tunnel resistivity deep learning inversion method based on physics-driven and signal interpretability
NEAR SURFACE GEOPHYSICS(2024)
摘要
Data-driven deep learning technology has a strong non-linear mapping ability and has good development potential in geophysical inversion problems. Traditional inversion techniques offer broad generality, but they can remain trapped in local minima, particularly for three-dimensional tunnelling resistivity inversion. In this work, we present an inversion methodology that combines traditional physics-driven and deep learning data-driven inversion approaches. To further support deep neural networks' dependability on unseen data, the interpretability of their working mechanism is explored. We execute migration learning based on small sample data after identifying the critical parameters that restrict the effectiveness of inversion by analysing the feature maps of various model data. We demonstrate, using both synthetic examples and field data, that the proposed method can improve the accuracy in detecting water-bearing anomalies (caves and faults), which are typically encountered during tunnel excavation.
更多查看译文
关键词
electrical resistivity,tomography,inversion,tunnel
AI 理解论文
溯源树
样例
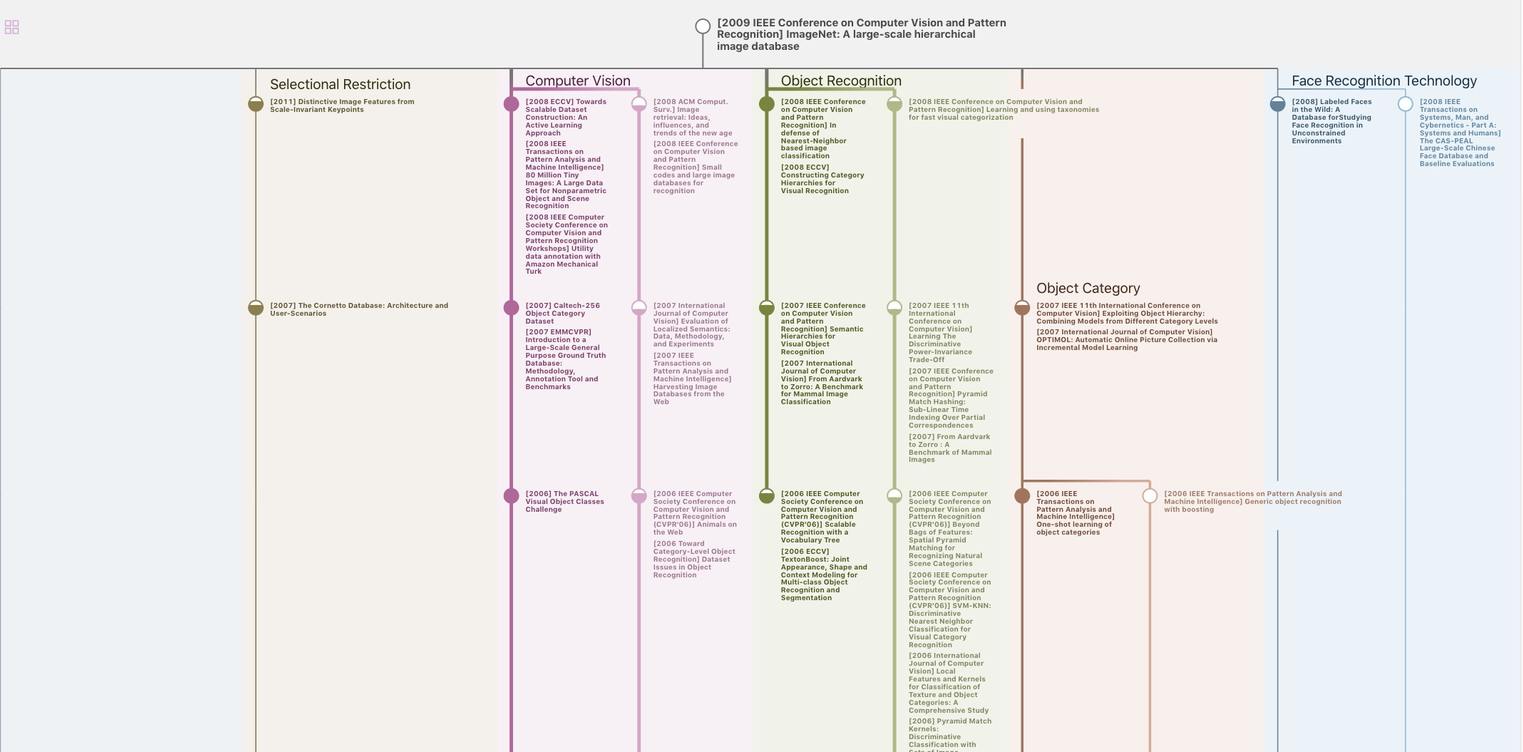
生成溯源树,研究论文发展脉络
Chat Paper
正在生成论文摘要