Online learning for streaming data classification in nonstationary environments
STATISTICAL ANALYSIS AND DATA MINING(2024)
摘要
In this article, we implement the classification of nonstationary streaming data. Due to the inability to obtain full data in the context of streaming data, we adopt a strategy based on clustering structure for data classification. Specifically, this strategy involves dynamically maintaining clustering structures to update the model, thereby updating the objective function for classification. Simultaneously, incoming samples are monitored in real-time to identify the emergence of new classes or the presence of outliers. Moreover, this strategy can also deal with the concept drift problem, where the distribution of data changes with the inflow of data. Regarding the handling of novel instances, we introduce a buffer analysis mechanism to delay their processing, which in turn improves the prediction performance of the model. In the process of model updating, we also introduce a novel renewable strategy for the covariance matrix. Numerical simulations and experiments on datasets show that our method has significant advantages.
更多查看译文
关键词
classification,concept drift,novel classes,renewable strategy,streaming data
AI 理解论文
溯源树
样例
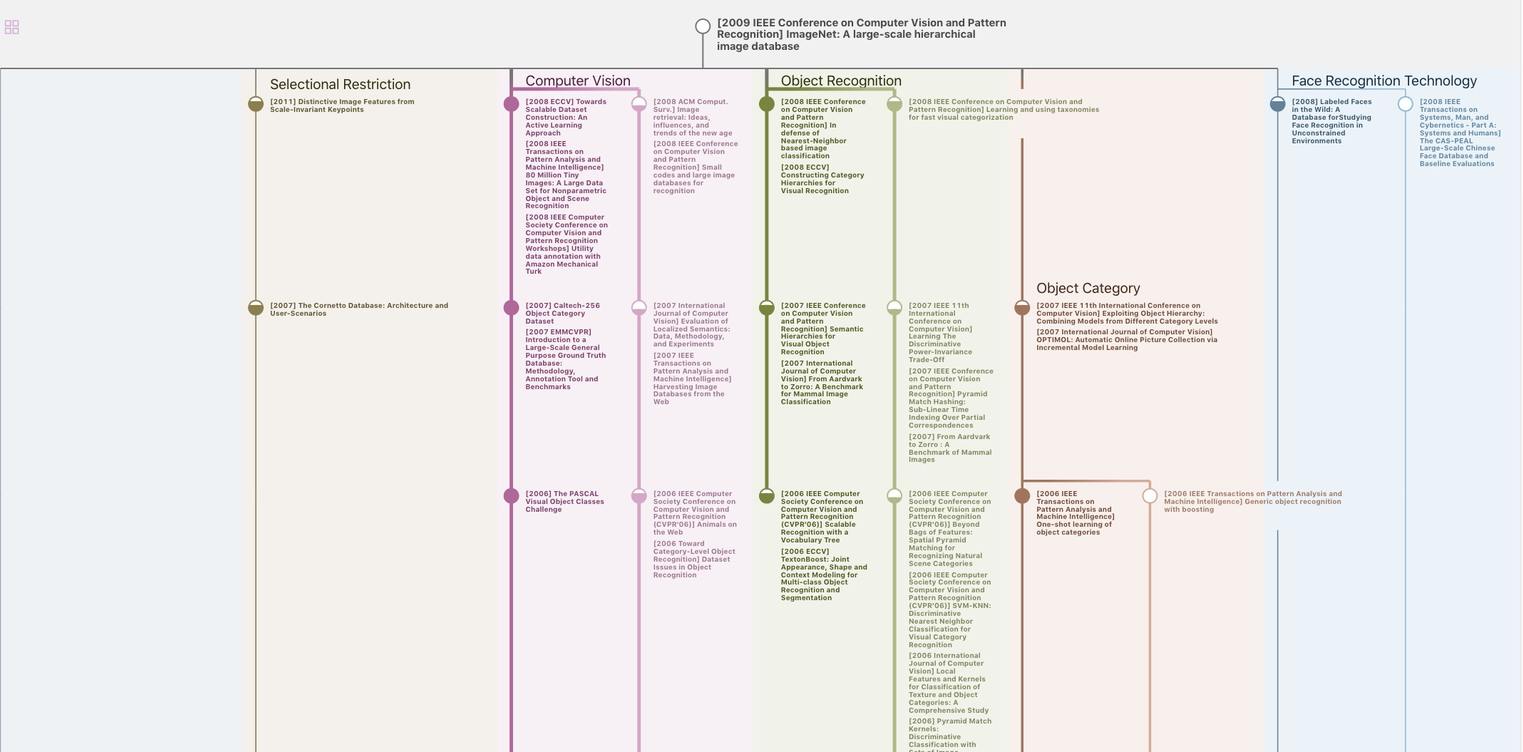
生成溯源树,研究论文发展脉络
Chat Paper
正在生成论文摘要