The Multi-Channel Maximum-Likelihood (MCML) method: towards a cost-effective multisource estimation algorithm
crossref(2024)
摘要
We present an improvement of the Multi-Channel Maximum-Likelihood (MCML) method [1]. This approach is based on the likelihood function derived from a multi-sensor stochastic model expressed in different frequency channels. Using the likelihood function, we determine, for the detection problem, the Generalized Likelihood Ratio (GLR) with a p-value threshold to discriminate signal of interest and noise. For the estimation of the slowness vector, we determine the Maximum Likelihood Estimation (MLE). Comparisons with synthetic and real datasets show that MCML, when implemented in the time-frequency domain, outperforms state-of-the-art detection algorithms in terms of detection probability and false alarm rate in poor signal-to-noise ratio scenarios. Mathematical extension of MCML is implemented in order to detect overlapping coherent signals in the same time frequency domain. This extension is based on the spectral matrix content. Taking into account the last detected source, the array response in the spectral matrix is iteratively subtracted to estimate a subsequent source of weaker energy. We show that the implemented approach allows detecting overlapping signals in the same time frequency window under various scenarios with varying signal-to-noise ratio (SNR), frequency bands and array geometry. Compared with the original method based on multiple maxima selection on the monosource likelihood function, the new approach yields improved wave parameter estimates. Results are illustrated by synthetics and real data recorded by stations part of the International Monitoring System (IMS). [1] B. Poste et al. (2022), The Multi-Channel Maximum-Likelihood (MCML) method: a new approach for infrasound detection and wave parameter estimation, Geophysical Journal International, https://doi.org/10.1093/gji/ggac377
更多查看译文
AI 理解论文
溯源树
样例
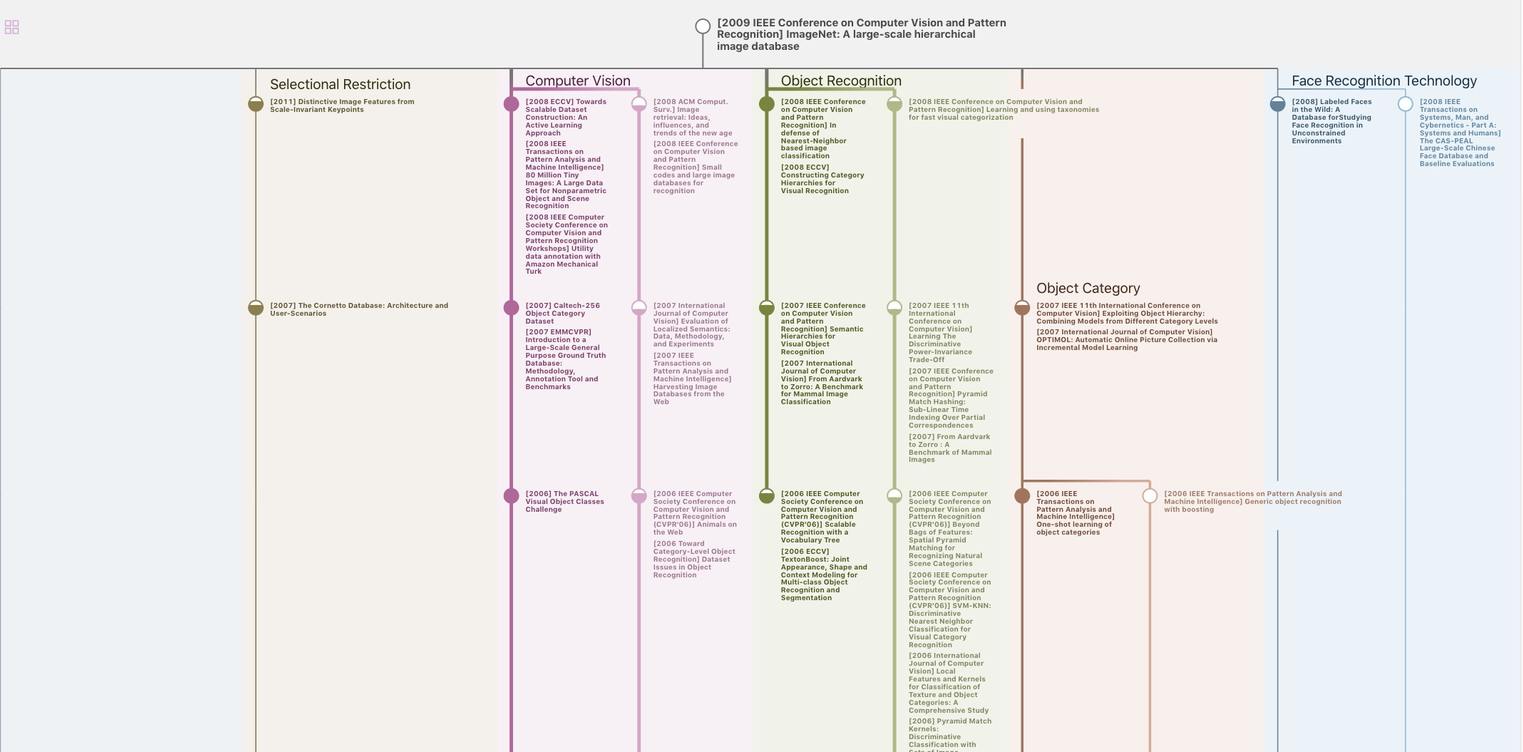
生成溯源树,研究论文发展脉络
Chat Paper
正在生成论文摘要