Preliminary Study on Minutely Sample Labeling Algorithm with Prior Knowledge Model 
crossref(2024)
摘要
Keywords: Dual-Polarization, Minutely Dynamic Tracking, Prior Knowledge Physical Constraints, Deep Learning Considering the suddenness and locality of short duration heavy rainfall (SDHR) and the motion of the associated convection precipitation echo, we propose an automatic sample labeling algorithm using minutely automatic weather station data. Firstly, we filtered out the stations with a sliding cumulative hourly rainfall exceeding 20 millimeters, and then conducted dynamic tracking for several hours. The tracking window remains consistent during an event. Then we extract corresponding volume scan data and obtained 21,706 samples. Compared to grid data, these samples retain the raw radial reflectivity and velocity, which can reveal the dynamic characteristics within precipitation cloud. Moreover, dual-polarization parameters can offer precise advantage in estimating SDHR. Based on the novel spatiotemporal prior-informed deep network (STPiDN) that incorporates seismic spatiotemporal prior knowledge into deep neural networks using limited historical samples[1]. We propose an end-to-end SDHR model that considers fundamental physical prior knowledge and spatiotemporal development of heavy rainfall events. We develop a gated convolutional encoder-decoder network that effectively characterizes the spatiotemporal patterns of SDHR. This network is built upon fluid-individualized variation-guided gating convolutional units, and a comprehensive loss function. In particular, a spatiotemporal consistency loss is designed to facilitate the generation of predictive results that conform to the actual spatiotemporal patterns of SDHR formation, development, and dissipation . The model is trained based on gridded data, and the results show that the predictive model outperforms multiple existing models[2]. More efforts will involve training the proposed model based on radial samples, considering the basic physical prior knowledge and spatio-temporal development patterns of precipitation. This work was supported in part by the Joint Research Project for Meteorological Capacity Improvement under Grant 22NLTSY015, Hubei Province Key R&D Plan 2023BCB119 and Hubei Province Meteorological Bureau Project 2022Y04. [1] Liu, J., Zhang, T.*, Gao, C., and Wang, P. (2023). Forecasting Earthquake Magnitude and Epicenter by Incorporating Spatio-temporal Priors into Deep Neural Networks. IEEE Transactions on Geoscience and Remote Sensing 61:5911413. DOI:10.1109/TGRS.2023.3281784. [2] Zhang, T., Liu, J.*, Gao, C., Wang, P., Leng, L. Xiao, Y. (2023). Prior-Guided Gated Convolutional Networks for Rainstorm Forecasting. Journal of Hydrology. (Under review)
更多查看译文
AI 理解论文
溯源树
样例
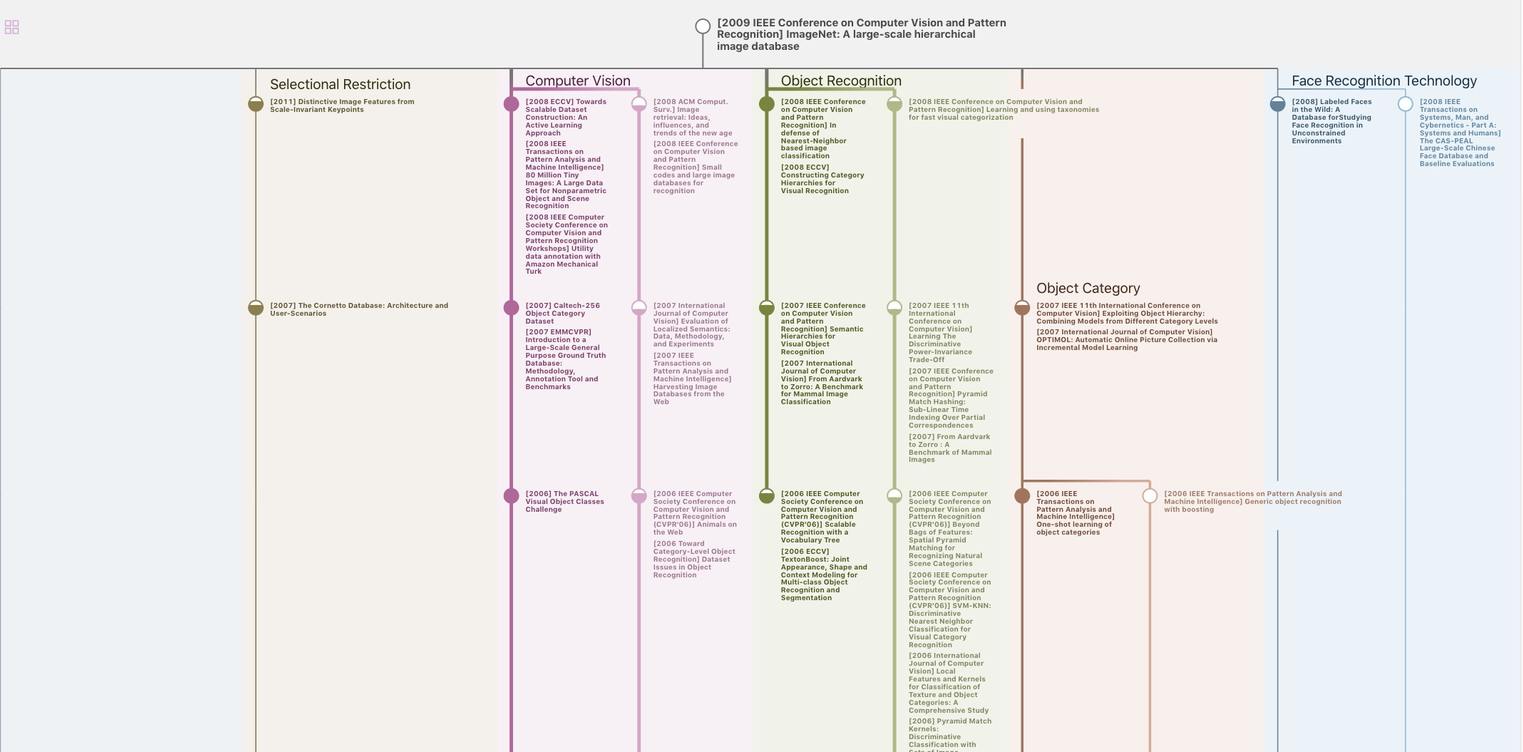
生成溯源树,研究论文发展脉络
Chat Paper
正在生成论文摘要