Identifying uncataloged low-frequency earthquake sources with deep learning
crossref(2024)
摘要
Tectonic faults exhibit a wide spectrum of processes releasing stress: from fast earthquakes to slow deformation. Mapping smaller-scale slow deformation directly is challenging because of the limited signal-to-noise ratio of geodetic recordings. Instead, we need to rely on other telltale signs of slow deformation. Low-frequency earthquakes (LFEs) are such signs: a class of seismically observable signals that coincide with slow deformation. However, detecting LFEs is challenging due to their emergent nature and generally low magnitude. The most common method for LFE detection is template matching. Due to the need for waveform templates, this method is specific to a set of LFE sources and seismic stations. This limitation renders the template matching unable to discover uncataloged sources or detect LFEs in regions without prior known LFE activity. Here, we present a novel, deep learning method for detecting LFEs. Our method detects phase arrivals from LFEs, allowing to detect them with a workflow closely modeled after a standard earthquake detection workflow. In contrast to template matching, the deep learning method is substantially more flexible and can generalise to unknown LFEs and even across world regions. We apply our method to a diverse set of regions, including regions with previously known LFE activity, such as Cascadia and Nankai, and without known LFE activity, such as Northern Chile.
更多查看译文
AI 理解论文
溯源树
样例
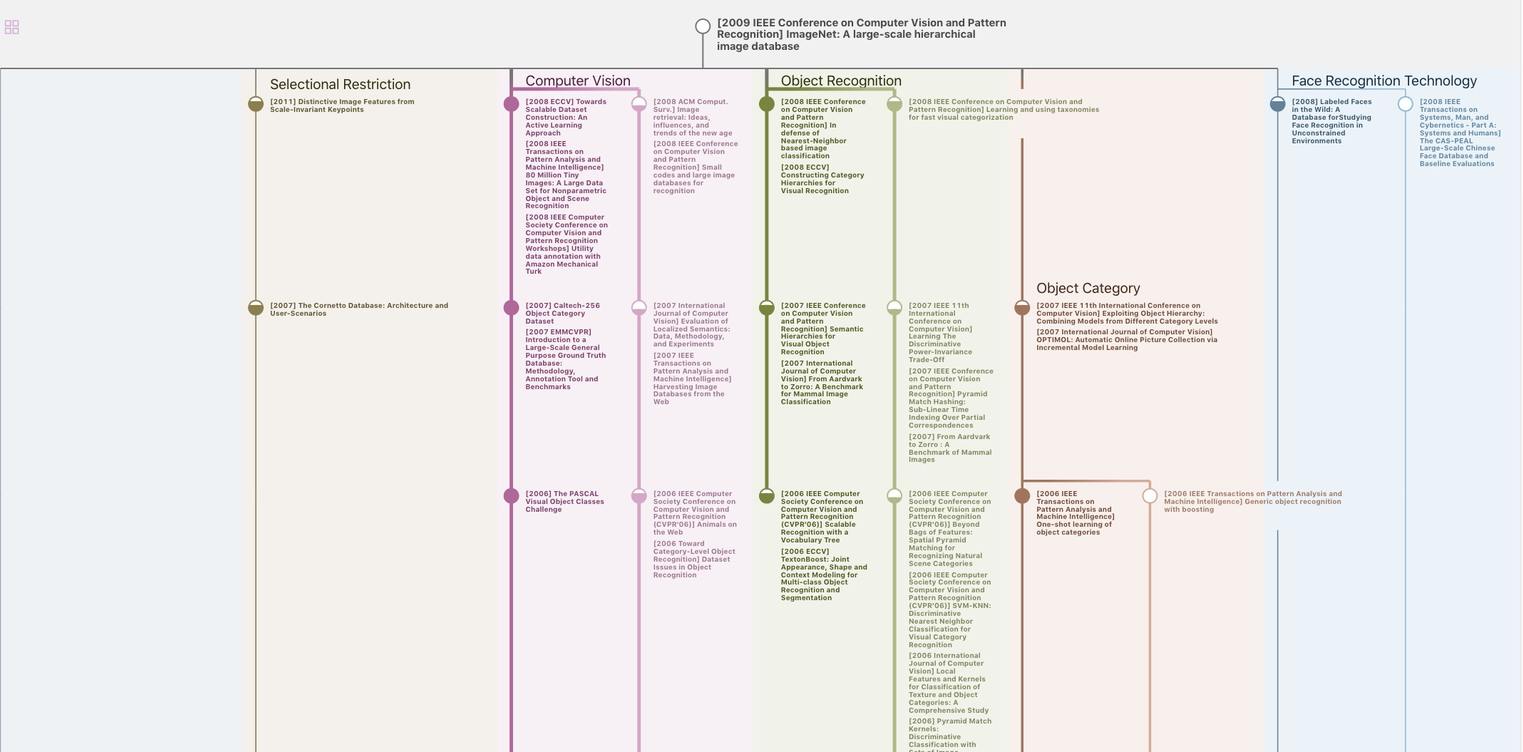
生成溯源树,研究论文发展脉络
Chat Paper
正在生成论文摘要