A severity estimation method for lightweight cucumber leaf disease based on DM-BiSeNet
Information Processing in Agriculture(2024)
摘要
Accurately estimating the severity of cucumber diseases is crucial for improving cucumber quality and minimizing economic losses. Deep learning techniques have shown promising results in automatically extracting disease image features for severity estimation. However, existing methods still face challenges in accurately estimating disease severity under complex backgrounds and achieving real-time performance.This paper presents a lightweight severity estimation method called DM-BiSeNet to address these challenges. The proposed method utilizes BiSeNet V2 as the base network and incorporates depthwise separable convolutional blocks to optimize the detail branch. A simplified MobileNet V3 network is also constructed to optimize the semantic branch. The model training process is accelerated using the AdamW optimizer. To evaluate the performance of DM-BiSeNet, a dataset consisting of cucumber powdery mildew and downy mildew disease images collected in natural scenes is utilized. Experimental results demonstrate that DM-BiSeNet achieves higher accuracy in severity estimation, with an R2 value of 0.9407 and an RMSE of 1.0680, outperforming the comparison methods. Moreover, DM-BiSeNet exhibits a complexity of 1.54 GFLOPs and is capable of reasoning 94 disease images per second.The proposed DM-BiSeNet model offers a lightweight and effective solution for accurate and rapid severity estimation of cucumber diseases under complex backgrounds. It provides a valuable technical tool for quantitative disease estimation, offering significant potential for practical applications.
更多查看译文
关键词
Cucumber disease,Severity estimation,Depthwise separable convolution,Real-time semantic segmentation
AI 理解论文
溯源树
样例
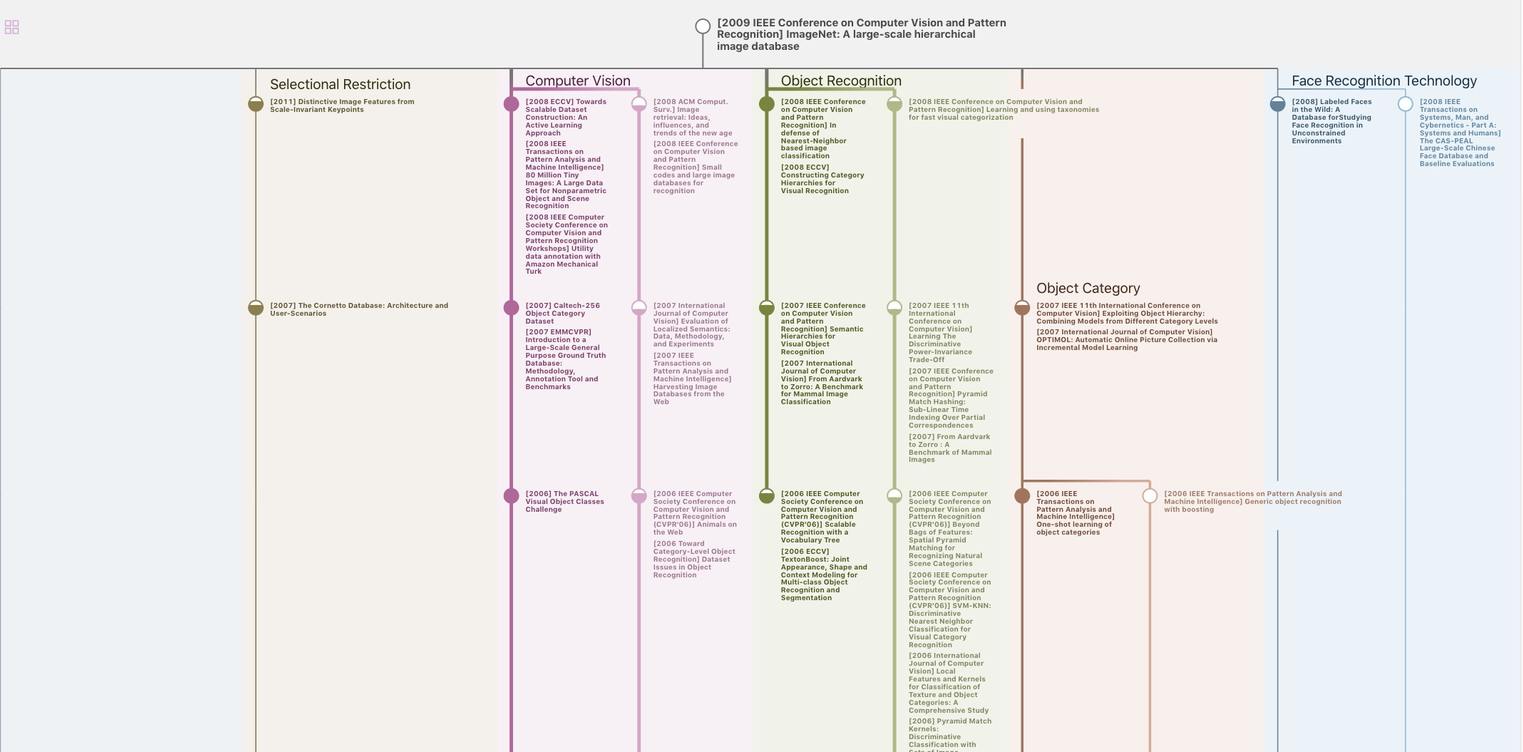
生成溯源树,研究论文发展脉络
Chat Paper
正在生成论文摘要