Quantifying the influence of cloud controlling factors with causal inference
crossref(2024)
摘要
As a key component of the hydrological cycle and the Earth’s radiation budget, clouds play an important role in both weather and climate. Our incomplete understanding of clouds and their role in cloud-climate feedbacks leads to large uncertainties in climate simulations. Using causal discovery as an unsupervised machine learning method we aim to systematically analyse and quantify causal interdependencies and dynamical links between cloud properties and their controlling factors. This approach goes beyond correlation-based measures by systematically excluding common drivers and indirect links. By estimating the causal effect of each of the cloud controlling factors for different cloud regimes we expect to be able to better understand the dominant processes which determine the micro- and macro-physical properties of clouds. Specifically, causal inference is used to investigate the links between cloud properties such as cloud cover, cloud water path, cloud top height and cloud radiative effects and so-called cloud controlling factors, i.e., quantities that impact cloud formation and temporal evolution of the cloud (e.g., sea surface temperature, water vapour path and lower tropospheric stability). For this, causal networks are calculated from time series of these variables from satellite and reanalysis datasets averaged over different geographical regions and cloud regimes in order to quantify the strength of the individual links in the resulting causal graph by applying causal effect estimation.
更多查看译文
AI 理解论文
溯源树
样例
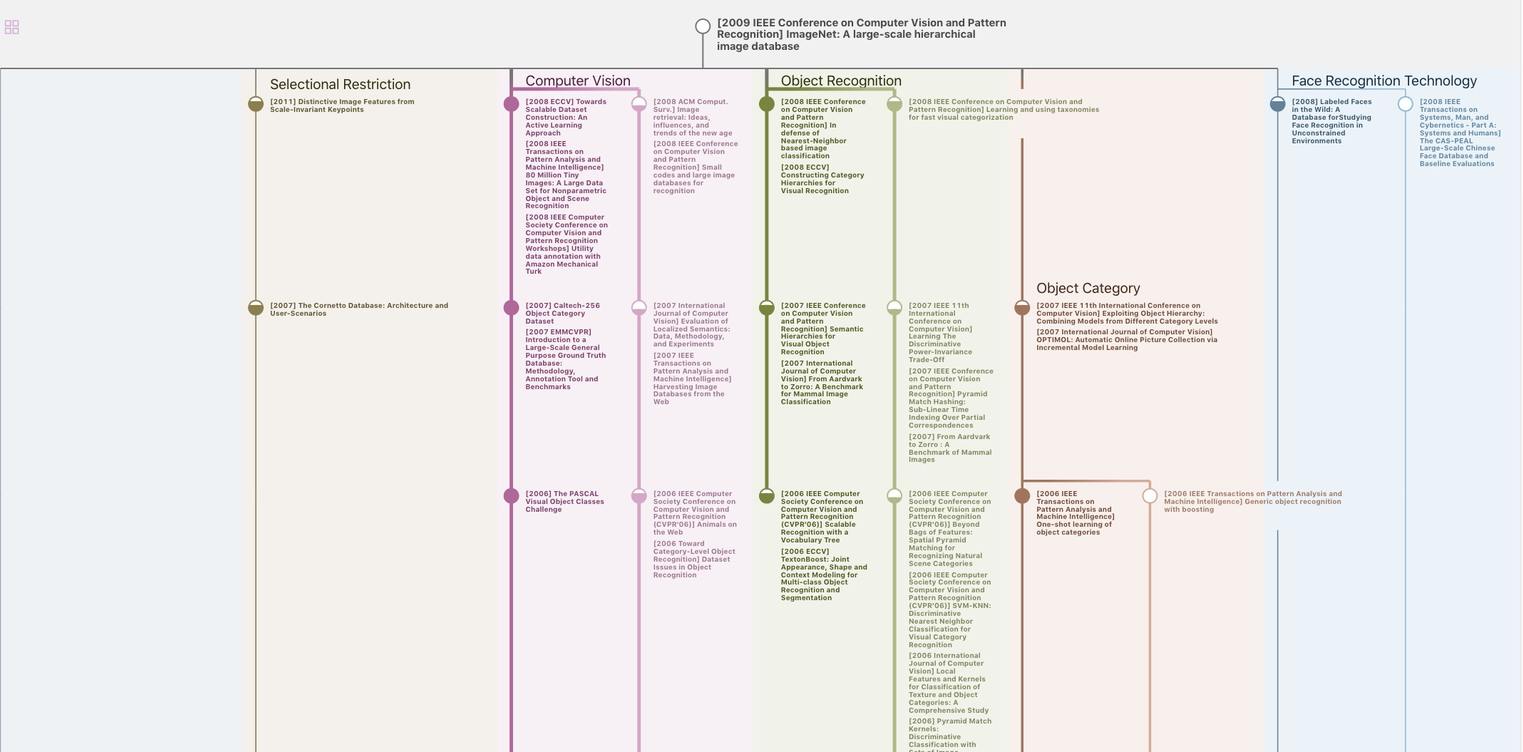
生成溯源树,研究论文发展脉络
Chat Paper
正在生成论文摘要