Semantic-Driven Autonomous Visual Navigation for Unmanned Aerial Vehicles
IEEE TRANSACTIONS ON INDUSTRIAL ELECTRONICS(2024)
摘要
Aiming at the autonomous navigation of unmanned aerial vehicles (UAVs) in complex and unknown environments, this article combines transfer reinforcement learning theory with an end-to-end mapless visual navigation method and proposes a semantic-driven autonomous navigation method for UAVs. First, we propose a reality reconstruction motion decision model, which consists of two parts: reality reconstruction and motion decision. The reality reconstruction part encodes the environment state into a unified feature space by combining semantic information and raw visual perception to generate a more efficient state description. In the motion decision part, we propose a motion decision network for navigation based on temporal attention and spatial attention mechanisms, which plans its own spatial perception according to key features and important memories to generate highly universal strong temporal navigation motion decisions. Subsequently, the action module generates and executes the optimal actions that the UAV can perform based on the decision results. The simulation results show that the UAV can autonomously explore and actively acquire navigation target position and complete collision-free autonomous navigation from any position to the navigation target in the absence of global information and unknown target positions. The real-world experimental testing results further demonstrate that this method improves the utilization efficiency of perception resources for UAVs, solves the reality gap problem, and has strong transferability. It can be directly transferred to challenging scenarios such as dynamic targets, multiple obstacles, and changes in target and obstacle types without the need for retraining. Overall, it is a highly autonomous and adaptive navigation method.
更多查看译文
关键词
Mapless visual navigation,reality gap problem,reality reconstruction motion decision (RRMD) model,transfer reinforcement learning (RL),unmanned aerial vehicle (UAV)
AI 理解论文
溯源树
样例
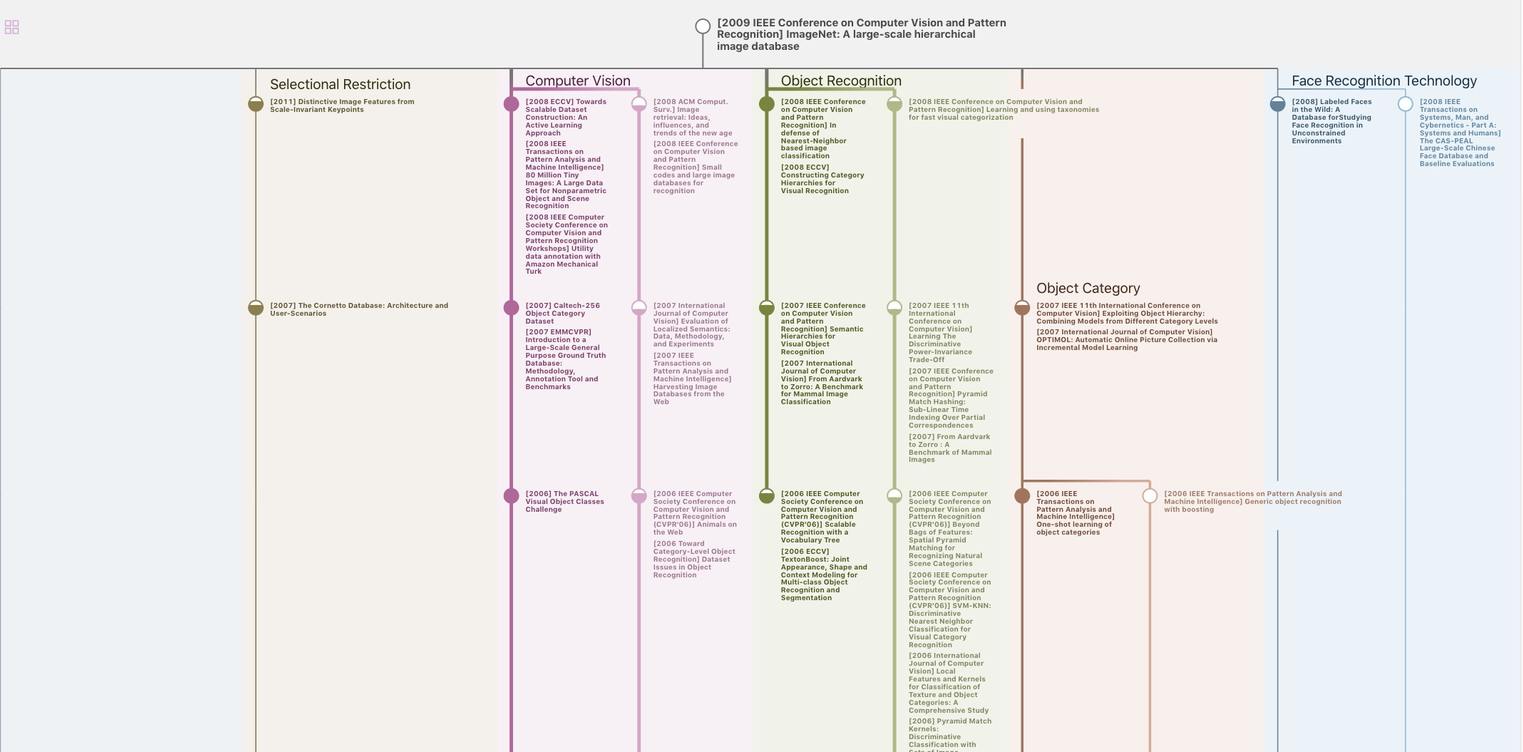
生成溯源树,研究论文发展脉络
Chat Paper
正在生成论文摘要