KNMI’23 climate scenarios for the Netherlands: storyline scenarios of regional climate change
crossref(2024)
摘要
We will present the methodology for the construction, and the outcomes, of the KNMI’23 national climate scenarios for the Netherlands. The KNMI’23 national climate scenarios consist of six scenarios that cover a substantial part of the uncertainty in CMIP6 projections of future climate change in the region. They form a detailed storyline of plausible future climates in the Netherlands. The resulting data is used for impact calculations and assessments by stakeholders, and will be used to inform policy making in different sectors of Dutch society. We have disentangled different sources of uncertainty as much as possible, partly by means of a storyline approach. Uncertainty in future emissions is covered by making scenarios conditional on different SSP scenarios (SSP1-2.6, SSP2-4.5, and SSP5-8.5). For each SSP scenario and time horizon (2050, 2100, 2150), we determine a global warming level based on the median of the constrained estimates of climate sensitivity from IPCC AR6. The remaining climate model uncertainty of the regional climate response at these warming levels is covered by two storylines, which are designed with a focus on the annual and seasonal mean precipitation response (a dry-trending and wet-trending variant for each SSP). This choice was motivated by the importance of future water management to society. For users with specific interests we provide means how to account for the impact of the uncertainty in climate sensitivity. Since CMIP6 GCM data do not provide the required spatial detail for impact modelling, we reconstruct the CMIP6 responses by resampling internal variability in a GCM-RCM initial-condition ensemble. More information on: https://www.knmi.nl/klimaatscenarios (see ‘toolkit’ for material in English).
更多查看译文
AI 理解论文
溯源树
样例
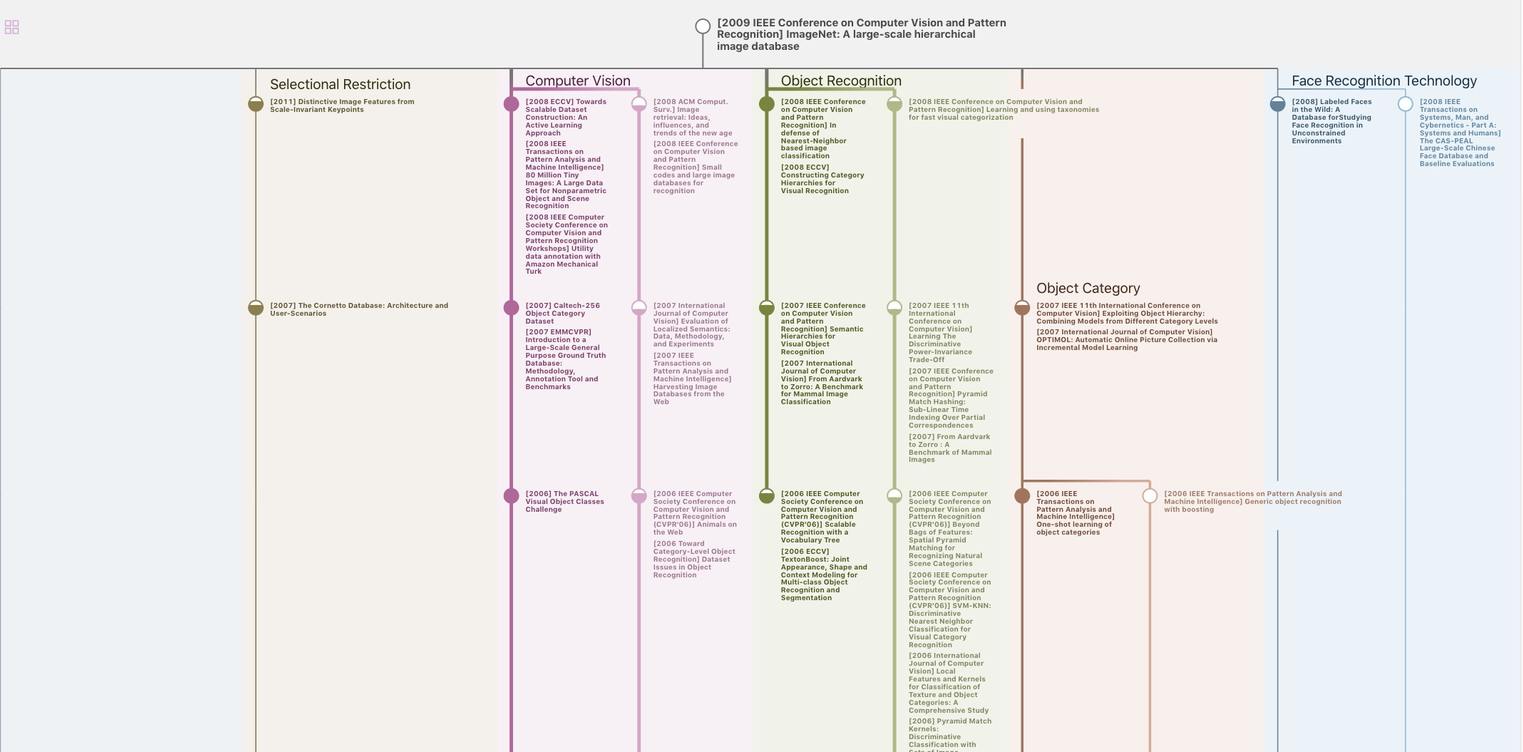
生成溯源树,研究论文发展脉络
Chat Paper
正在生成论文摘要