Information Filtering Unit-based Long Short-Term Memory Network for Industrial Soft Sensor Modeling
IEEE Sensors Journal(2024)
摘要
The use of soft sensors is an alternative solution to physical sensors for real-time acquisition of key quality variables that cannot be measured online in real-time. LSTM-based methods have been improving the state-of-the-art in soft sensors. In order to mine the correlation between historical information and current input, and utilize the relatively important historical information, we propose an underlying recurrent neural network unit called information filtering unit (IFU) and an IFU-based LSTM soft sensor (IF-LSTM). In IFU, a feed forward neural network is firstly constructed to predict the hidden state of the previous time step based on the current input and subsequently a scaling factor is obtained, and the hidden state vector is then scaled to highlight those important components that contain non-redundant information with the current input. Finally, an IF-LSTM model is constructed by combining IFU and LSTM units for predicting hard-to-obtain key quality variables from easily-measured process variables, and its performance is validated on two benchmark industrial datasets (SRU and DC datasets). Experimental results show that the performance of the IF-LSTM outperforms popular RNN variants such as LSTM, GRU, Bi-LSTM and Bi-GRU in all metrics (RMSE, MAE, MAPE and R
2
), and achieves an R
2
of 0.9929 on the DC dataset, indicating that the predicted values are well-fitted to the true values. Furthermore, comparative experiments with state-of-the-art methods in the literature show that the IF-LSTM model achieves the best RMSE of 0.0069 on the DC dataset, and the second best RMSE of 0.0124 on the SRU dataset.
更多查看译文
关键词
Soft sensor,quality variables,long short-term memory (LSTM),information filtering unit (IFU),information filtering long short-term memory (IF-LSTM)
AI 理解论文
溯源树
样例
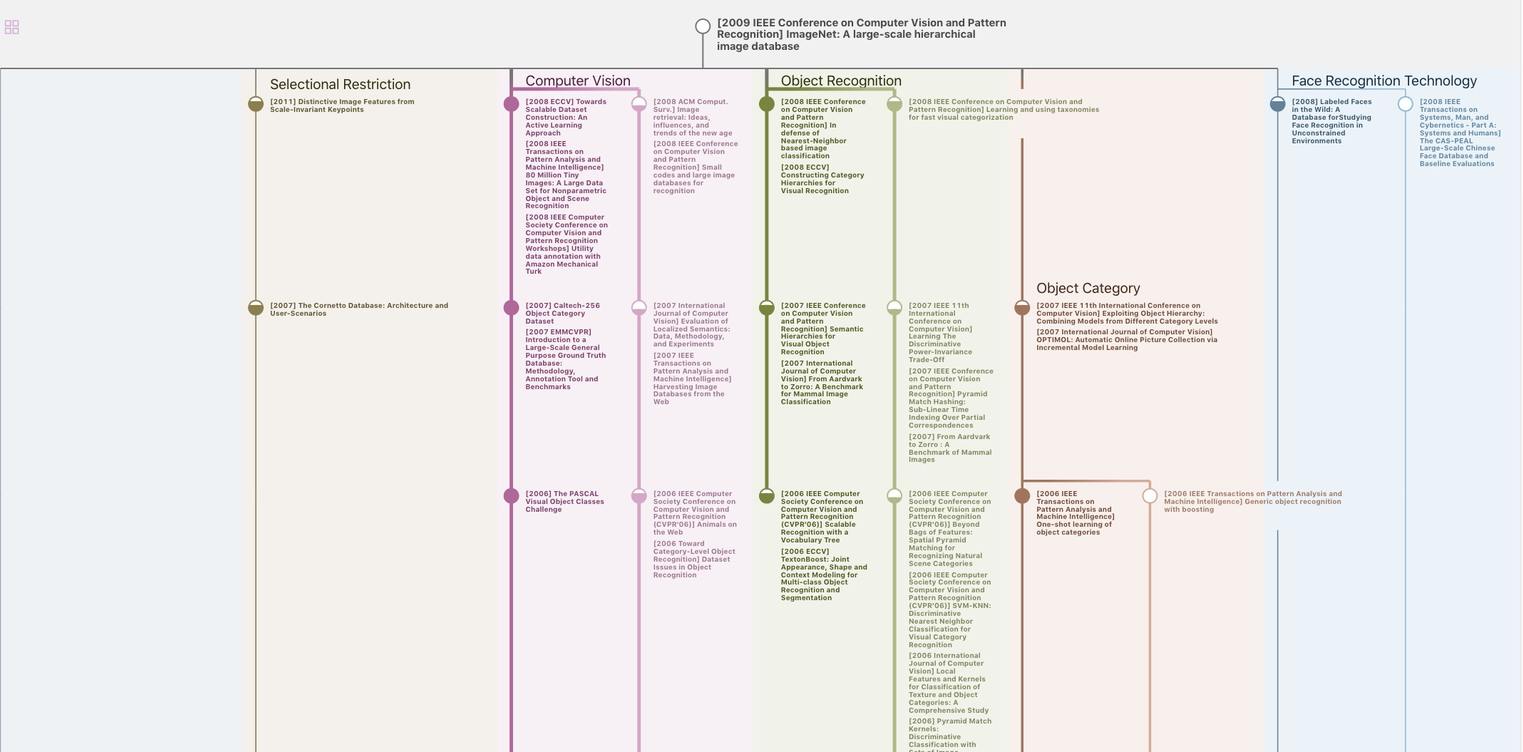
生成溯源树,研究论文发展脉络
Chat Paper
正在生成论文摘要