Hierarchical Graph Learning-Based Floorplanning With Dirichlet Boundary Conditions
IEEE TRANSACTIONS ON VERY LARGE SCALE INTEGRATION (VLSI) SYSTEMS(2024)
摘要
Floorplanning is a complex physical design problem that produces initial locations of movable objects, the quality of which has a great impact on downstream tasks such as placement and routing. To improve the efficacy of floorplanning, machine learning techniques have recently been recruited for help. However, the application-specific location constraints (IOs and cells with fixed locations) pose a huge challenge for machine learning. This article presents a novel uniformization approach by Dirichlet boundary conditions, which decomposes floorplanning into two easier-to-solve subproblems, namely a convex quadratic wirelength optimization problem with location constraints and an NP-hard combinatorial problem with homogeneous Dirichlet boundary conditions. The former problem is efficiently solved using quadratic optimization, and the latter is addressed by efficient graph inference using the proposed hierarchical GNN-based model. The proposed floorplanner called DPlanner has been integrated with state-of-the-art mixed-size placers to generate high-quality placement solutions with up to 56% and 41% improvement in placement iterations and runtime. In addition, compared to the state-of-the-art integrated floorplanning-placement flow, DPlanner achieves over a 20% improvement in placement iteration and more than a 21% reduction in total runtime, along with a 2% average reduction in wirelength.
更多查看译文
关键词
Deep learning,floorplanning,graph neural network,physical design,placement
AI 理解论文
溯源树
样例
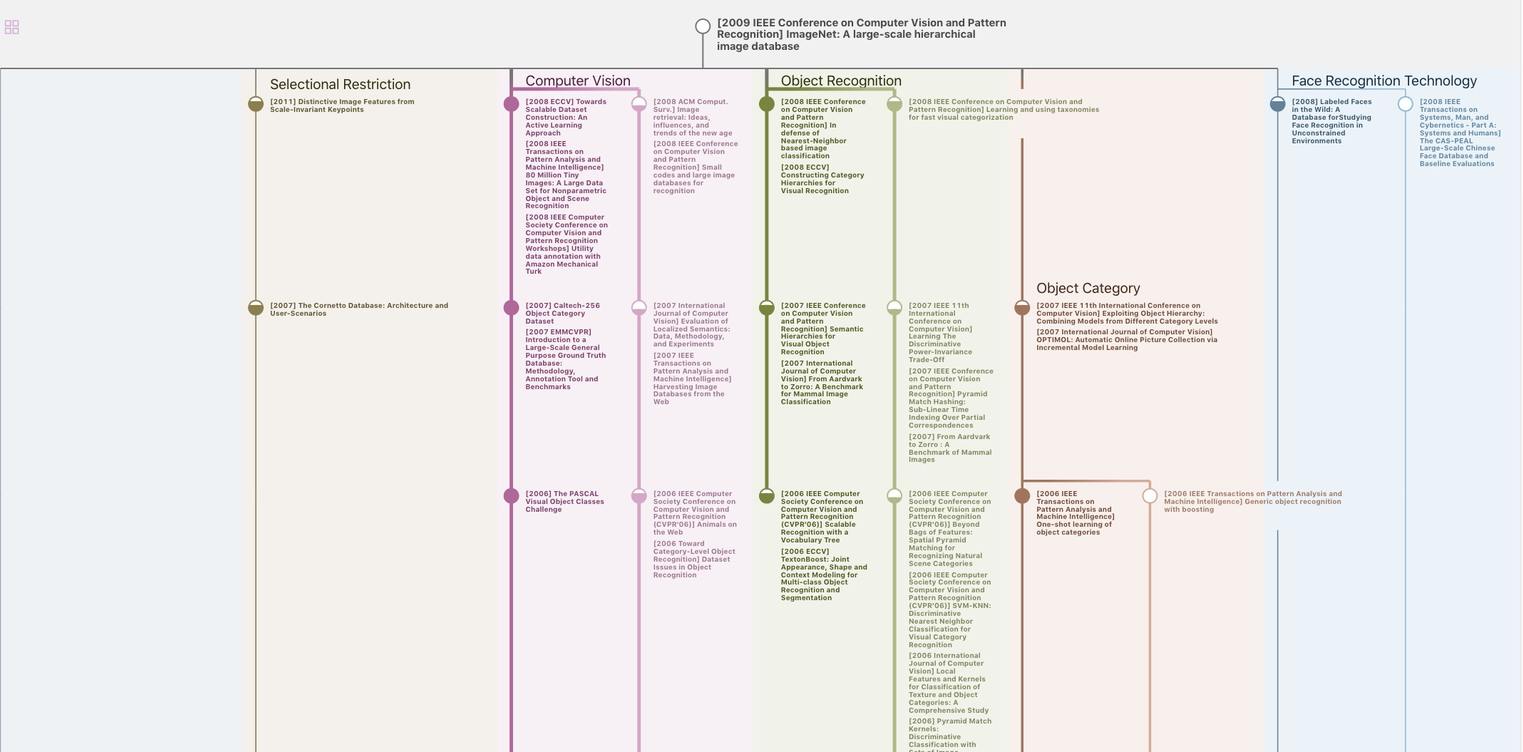
生成溯源树,研究论文发展脉络
Chat Paper
正在生成论文摘要