Hourly Long-Term Traffic Volume Prediction with Meteorological Information Using Graph Convolutional Networks
APPLIED SCIENCES-BASEL(2024)
摘要
Hourly traffic volume prediction is now emerging to mitigate and respond to hourly-level traffic congestion augmented by deep learning techniques. Incorporating meteorological data into the forecasting of hourly traffic volumes substantively improves the precision of long-term traffic forecasts. Nonetheless, integrating weather data into traffic prediction models is challenging due to the complex interplay between traffic flow, time-based patterns, and meteorological conditions. This paper proposes a graph convolutional network to predict long-term traffic volume with meteorological information. This study utilized a four-year traffic volume and meteorological information dataset in Chung-ju si to train and validate the models. The proposed model performed better than the other baseline scenarios with conventional and state-of-the-art deep learning techniques. Furthermore, the counterfactual scenarios analysis revealed the potential negative impacts of meteorological conditions on traffic volume. These findings will enable transportation planners predict hourly traffic volumes for different scenarios, such as harsh weather conditions or holidays. Furthermore, predicting the microscopic traffic simulation for different scenarios of weather conditions or holidays is useful.
更多查看译文
关键词
traffic volume prediction,graph convolutional networks,meteorological information
AI 理解论文
溯源树
样例
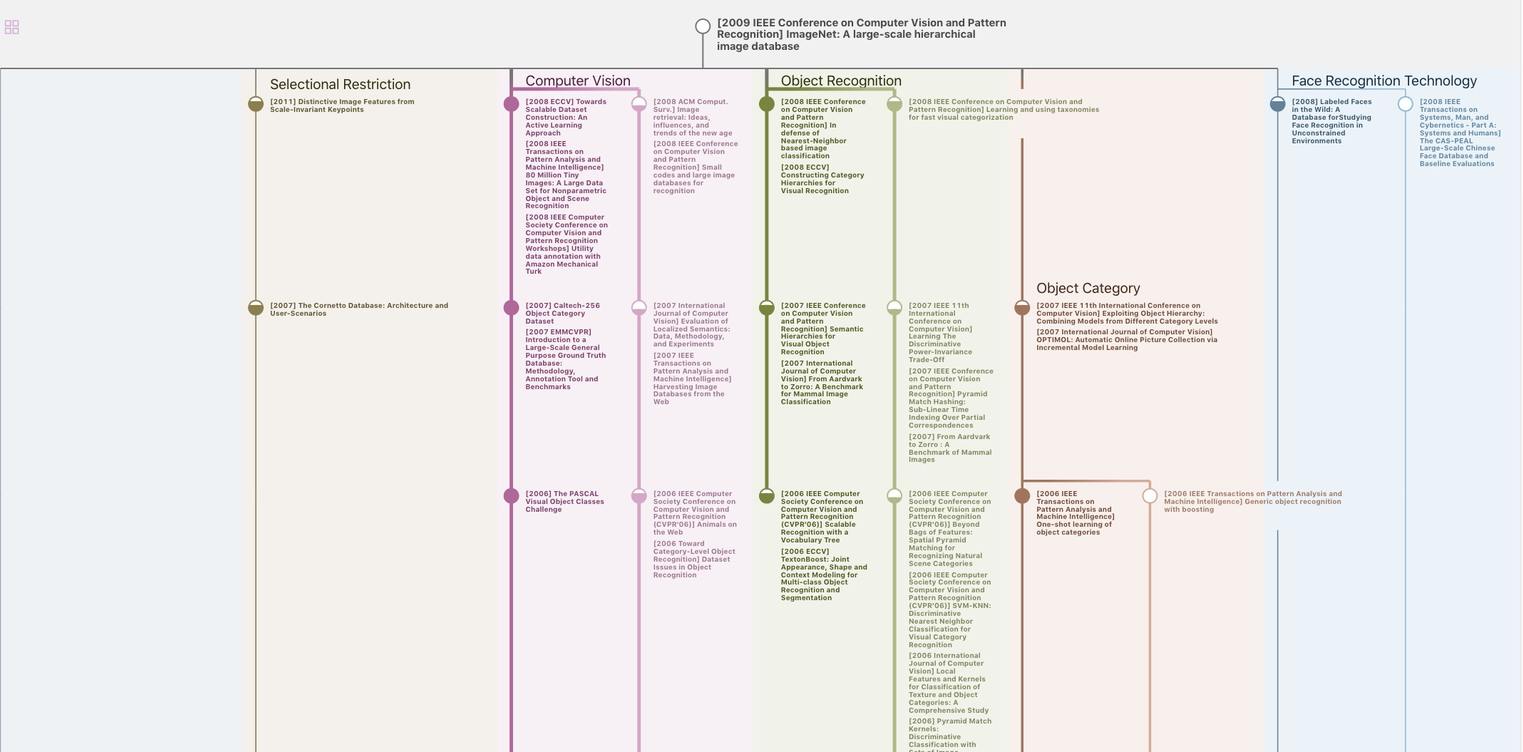
生成溯源树,研究论文发展脉络
Chat Paper
正在生成论文摘要