An Automated Conformalized Causal Learning System for Enhanced Mineral Prospectivity Mapping
crossref(2024)
摘要
Mineral Prospectivity Mapping (MPM) is a crucial process in mineral exploration, traditionally hampered by subjective interpretations and labor-intensive methods leading to unreliable outcomes. Predominantly focused on Independent and Identically Distributed (IID) scenarios, traditional research in MPM often struggles to generalize in Out of Distribution (OOD) scenarios, which are vital for accurate mineral exploration. Addressing these challenges, we introduce an innovative automated conformalized causal learning system for MPM. This system integrates a comprehensive data preprocessing pipeline that includes interpolation, feature filtering, data augmentation, and splitting, effectively managing diverse and imbalanced geological datasets. A central component of the system is Bayesian Optimization, autonomously selecting optimal machine learning models and hyperparameters to significantly enhance performance over non-automated methods. The system's most significant innovation is the incorporation of conformalized causal learning, exceptionally effective in handling OOD data scenarios. This methodology introduces an 'uncertainty region' in predictive models through conformal prediction, substantially reducing misclassification risks, while causal learning elucidates complex cause-and-effect relationships among geological features, essential for precise mineral deposit predictions. We evaluated the performance of our approach on six datasets, where the Area Under the Receiver Operating Characteristic (AUC ROC) of our automated optimized system surpassed the baseline method by an overall 17.84%, and the false positive rate (FPR) was reduced by an overall 84.31%. This development marks a significant advancement in MPM, enhancing accuracy and efficiency in mineral resource exploration and setting a new benchmark in the field. Released as an open-source platform, it offers the geological community a highly efficient, adaptable, and user-friendly tool, poised to revolutionize mineral prospectivity mapping in varied real-world scenarios.
更多查看译文
AI 理解论文
溯源树
样例
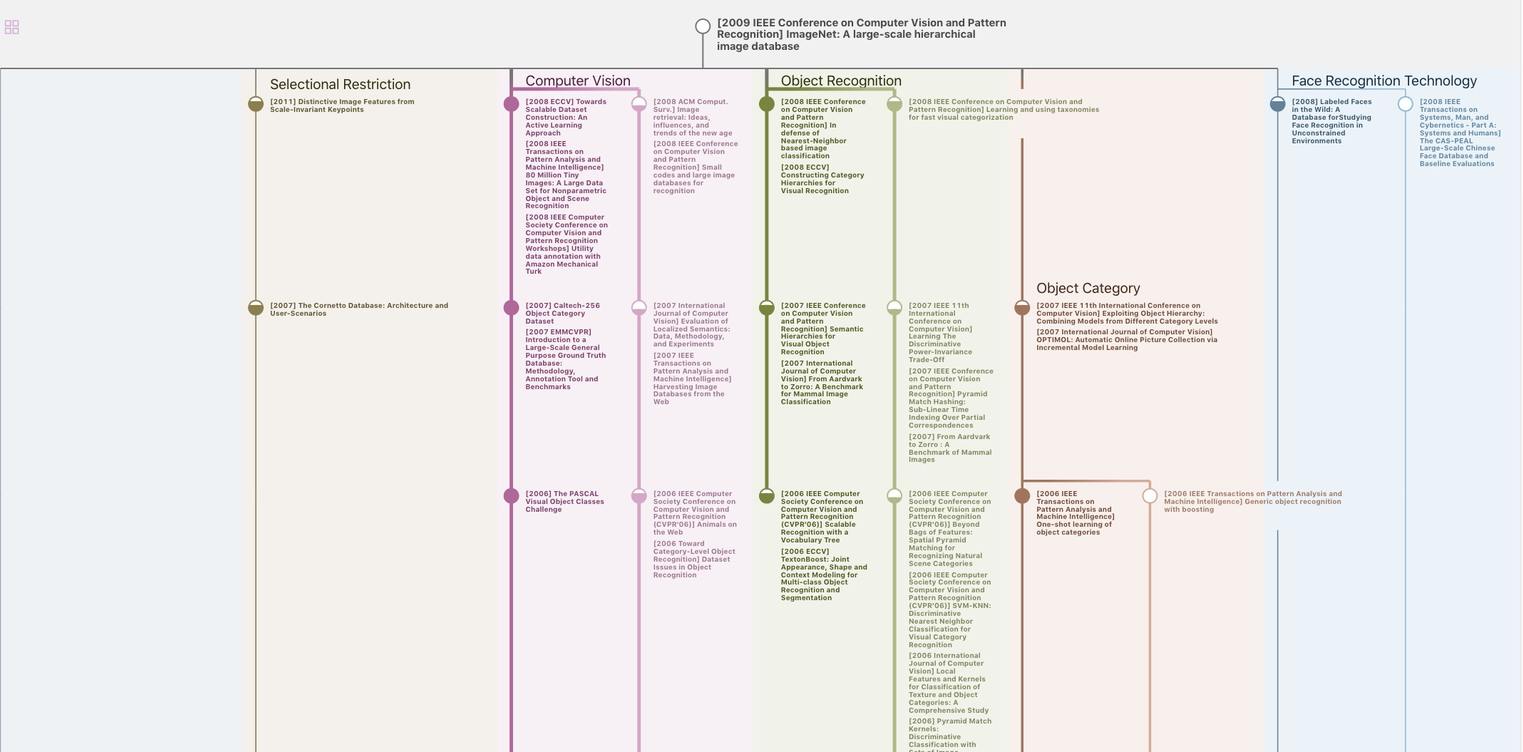
生成溯源树,研究论文发展脉络
Chat Paper
正在生成论文摘要