Hybrid Data-Induced Kalman Filtering Approach and Application in Beam Prediction and Tracking.
IEEE Trans. Signal Process.(2024)
摘要
Beam prediction and tracking (BPT) are key technology for high-frequency communications. Typical techniques include Kalman filtering and Gaussian process regression (GPR). However, Kalman filter requires explicit models of system dynamics, which are challenging to obtain, especially for complicated environments. In contrast, as a data-driven approach, there is no need to derive the system dynamics model for GPR. However, the computational complexity of GPR is often prohibitive, which makes real-time application challenging. To tackle this issue, we propose a novel hybrid model and data driven approach in this paper, which can exploit simultaneously the advantages from the two techniques while overcoming their drawbacks. In particular, the system dynamics required can be obtained in a data-driven manner. Based on a characterization of the system dynamics, we further investigate the long-term behavior of system evolution and propose two more efficient algorithms - long-term prediction and beam width optimization. We demonstrate two advantages of the proposed BPT approach. First, the computational complexity is low due to the inherent Kalman filter. Second, system performance can be significantly improved thanks to the long-term prediction and beam width optimization.
更多查看译文
关键词
Beam prediction,beam tracking,hybrid model and data driven,stochastic differential equation,Kalman filtering,Gaussian process,millimeter wave communications
AI 理解论文
溯源树
样例
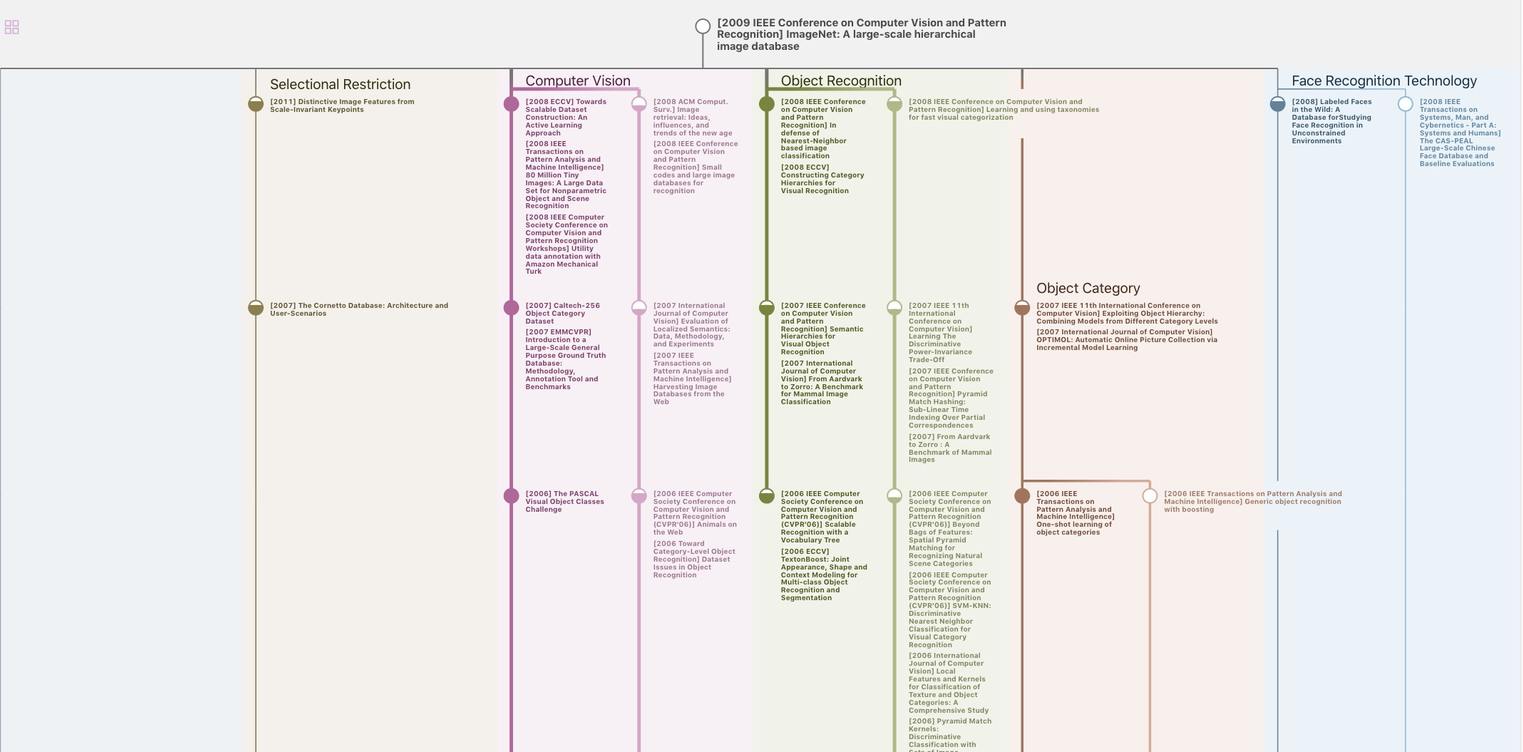
生成溯源树,研究论文发展脉络
Chat Paper
正在生成论文摘要