GraphsformerCPI: Graph Transformer for Compound–Protein Interaction Prediction
Interdisciplinary Sciences: Computational Life Sciences(2024)
摘要
Accurately predicting compound–protein interactions (CPI) is a critical task in computer-aided drug design. In recent years, the exponential growth of compound activity and biomedical data has highlighted the need for efficient and interpretable prediction approaches. In this study, we propose GraphsformerCPI, an end-to-end deep learning framework that improves prediction performance and interpretability. GraphsformerCPI treats compounds and proteins as sequences of nodes with spatial structures, and leverages novel structure-enhanced self-attention mechanisms to integrate semantic and graph structural features within molecules for deep molecule representations. To capture the vital association between compound atoms and protein residues, we devise a dual-attention mechanism to effectively extract relational features through .cross-mapping. By extending the powerful learning capabilities of Transformers to spatial structures and extensively utilizing attention mechanisms, our model offers strong interpretability, a significant advantage over most black-box deep learning methods. To evaluate GraphsformerCPI, extensive experiments were conducted on benchmark datasets including human, C. elegans, Davis and KIBA datasets. We explored the impact of model depth and dropout rate on performance and compared our model against state-of-the-art baseline models. Our results demonstrate that GraphsformerCPI outperforms baseline models in classification datasets and achieves competitive performance in regression datasets. Specifically, on the human dataset, GraphsformerCPI achieves an average improvement of 1.6
更多查看译文
关键词
CPI prediction,Deep learning,Molecular graph,Attention mechanism
AI 理解论文
溯源树
样例
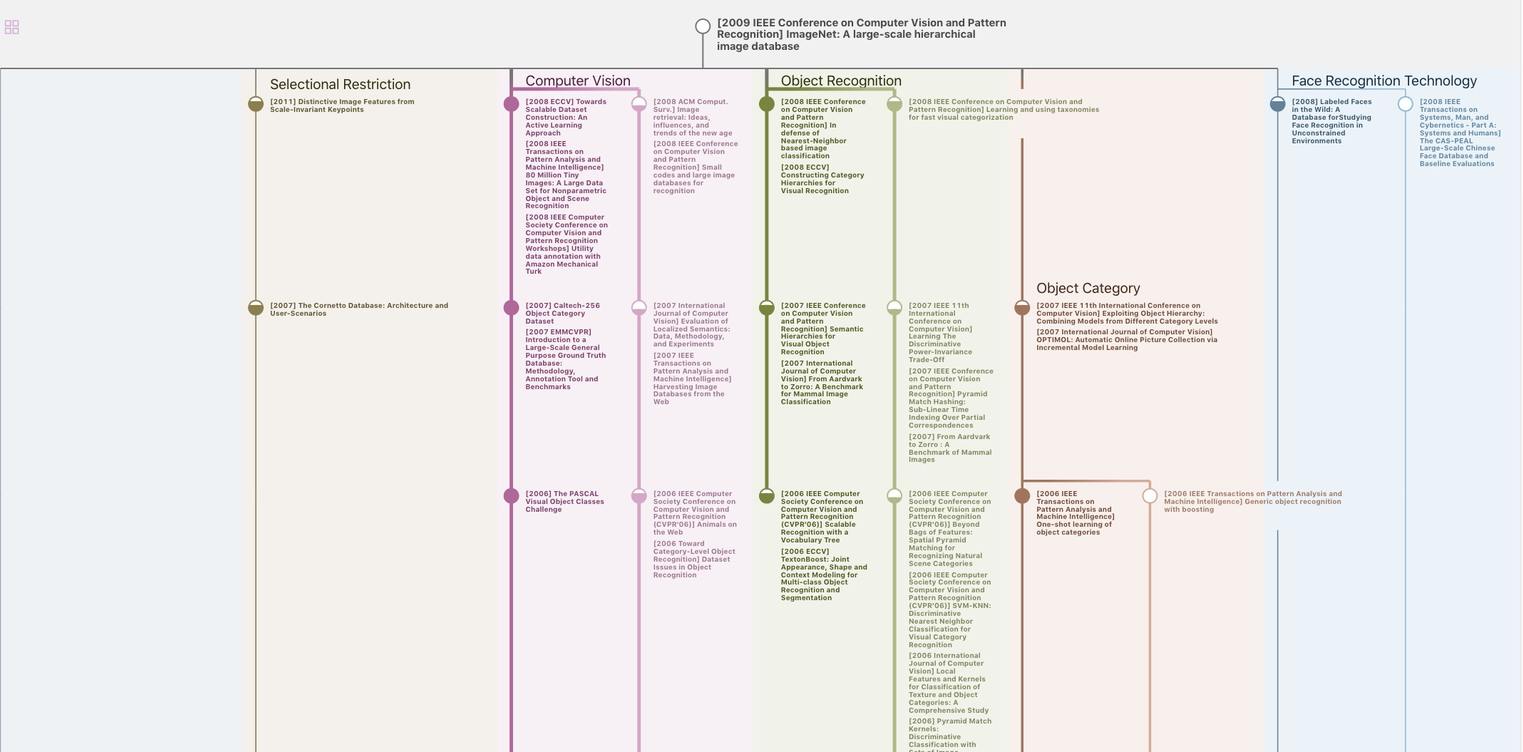
生成溯源树,研究论文发展脉络
Chat Paper
正在生成论文摘要