A first-principles exploration of the conformational space of sodiated di-saccharides assisted by semi-empirical methods and neural network potentials
PHYSICAL CHEMISTRY CHEMICAL PHYSICS(2024)
摘要
Previous exploration of the conformational space of sodiated mono-saccharides using a random search algorithm leads to similar to 103 structurally distinct conformers covering an energy range of similar to 150 kJ mol-1. Thus, it is reasonable to expect that the number of distinct conformers for a given disaccharide would be on the order of 106. Efficient identification of distinct conformers at the first-principles level has been demonstrated with the assistance of neural network potential (NNP) with an accuracy of similar to 1 kJ mol-1 compared to DFT. Leveraging a local minima database of neutral and sodiated glucose (Glc), we develop algorithms to systematically explore the conformation landscape of 19 Glc-based sodiated disaccharides. To accelerate the exploration, the NNP method is implemented. The NNP achieves an accuracy of similar to 2.3 kJ mol-1 compared to DFT, offering a comparable quality to that of DFT. Through a multi-model approach integrating DFTB3, NNP and DFT, we can rapidly locate low-energy disaccharide conformers at the first-principles level. The methodology we show here can be used to efficiently explore the potential energy landscape of any di-saccharides when first-principles accuracy is required. An NNP created by active learning explored conformations of sodiated glucose disaccharides; the resulting database of local minima enables simulations of infrared spectra or collision cross sections for ion mobility spectrometry.
更多查看译文
AI 理解论文
溯源树
样例
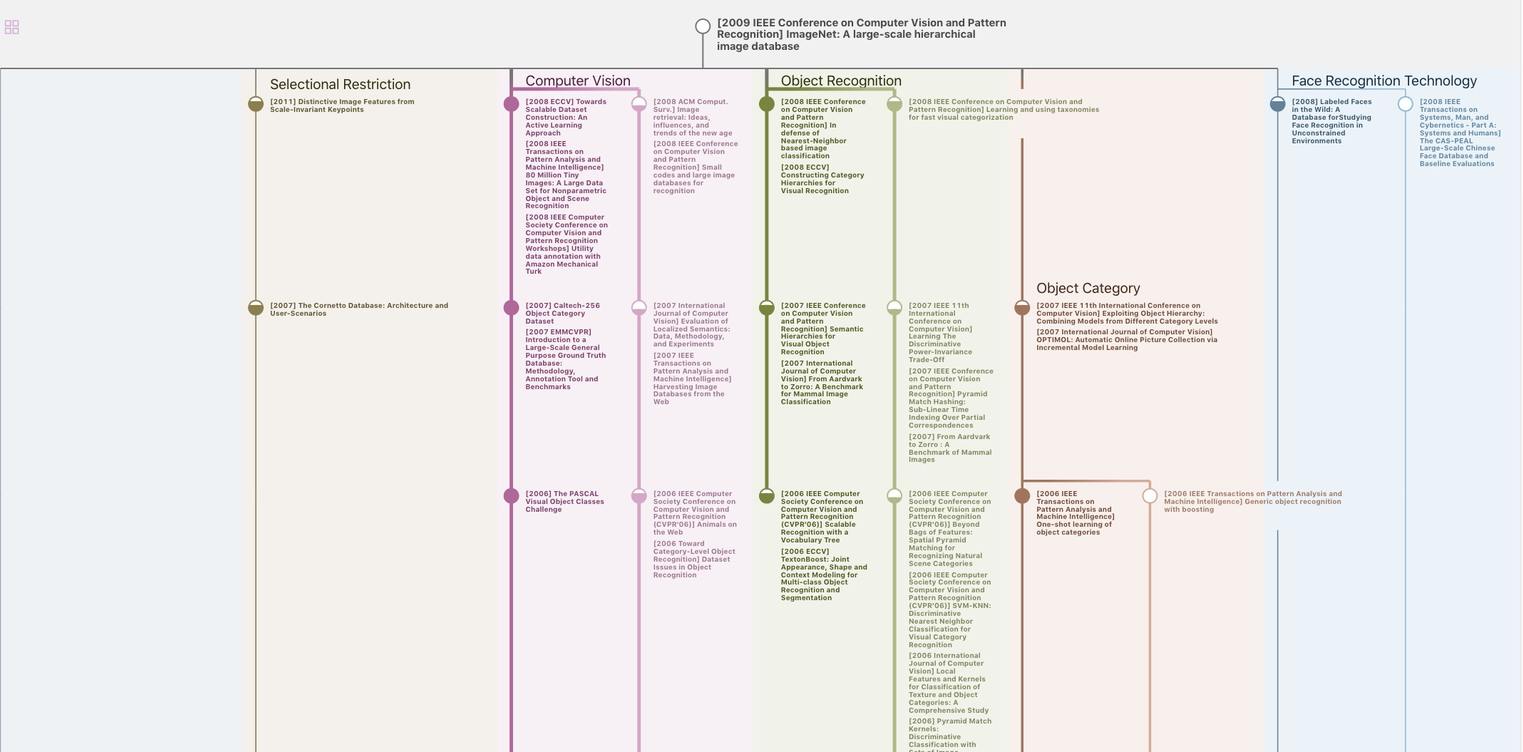
生成溯源树,研究论文发展脉络
Chat Paper
正在生成论文摘要