A scalable crop yield estimation framework based on remote sensing of solar-induced chlorophyll fluorescence (SIF)
Environmental Research Letters(2024)
摘要
Projected increases in food demand driven by population growth coupled with heightened agricultural vulnerability to climate change jointly pose severe threats to global food security in the coming decades, especially for developing nations. By providing real-time and low-cost observations, satellite remote sensing has been widely employed to estimate crop yield across various scales. Most such efforts are based on statistical approaches that require large amounts of ground measurements for model training/calibration, which may be challenging to obtain on a large scale in developing countries that are most food-insecure and climate-vulnerable. In this paper, we develop a generalizable framework that is mechanism-guided and practically parsimonious for crop yield estimation. We then apply this framework to estimate crop yield for two crops (corn and wheat) in two contrasting regions, the US Corn Belt US-CB, and India’s Indo–Gangetic plain Wheat Belt IGP-WB, respectively. This framework is based on the mechanistic light reactions (MLR) model utilizing remotely sensed solar-induced chlorophyll fluorescence (SIF) as a major input. We compared the performance of MLR to two commonly used machine learning (ML) algorithms: artificial neural network and random forest. We found that MLR-SIF has comparable performance to ML algorithms in US-CB, where abundant and high-quality ground measurements of crop yield are routinely available (for model calibration). In IGP-WB, MLR-SIF significantly outperforms ML algorithms. These results demonstrate the potential advantage of MLR-SIF for yield estimation in developing countries where ground truth data is limited in quantity and quality. In addition, high-resolution and crop-specific satellite SIF is crucial for accurate yield estimation. Therefore, harnessing the mechanism-guided MLR-SIF and rapidly growing satellite SIF measurements (with high resolution and crop-specificity) hold promise to enhance food security in developing countries towards more effective responses to food crises, agricultural policies, and more efficient commodity pricing.
更多查看译文
关键词
solar-induced chlorophyll fluorescence (SIF),crop yield,mechanistic light reactions,agricultural monitoring,satellite remote sensing,machine learning
AI 理解论文
溯源树
样例
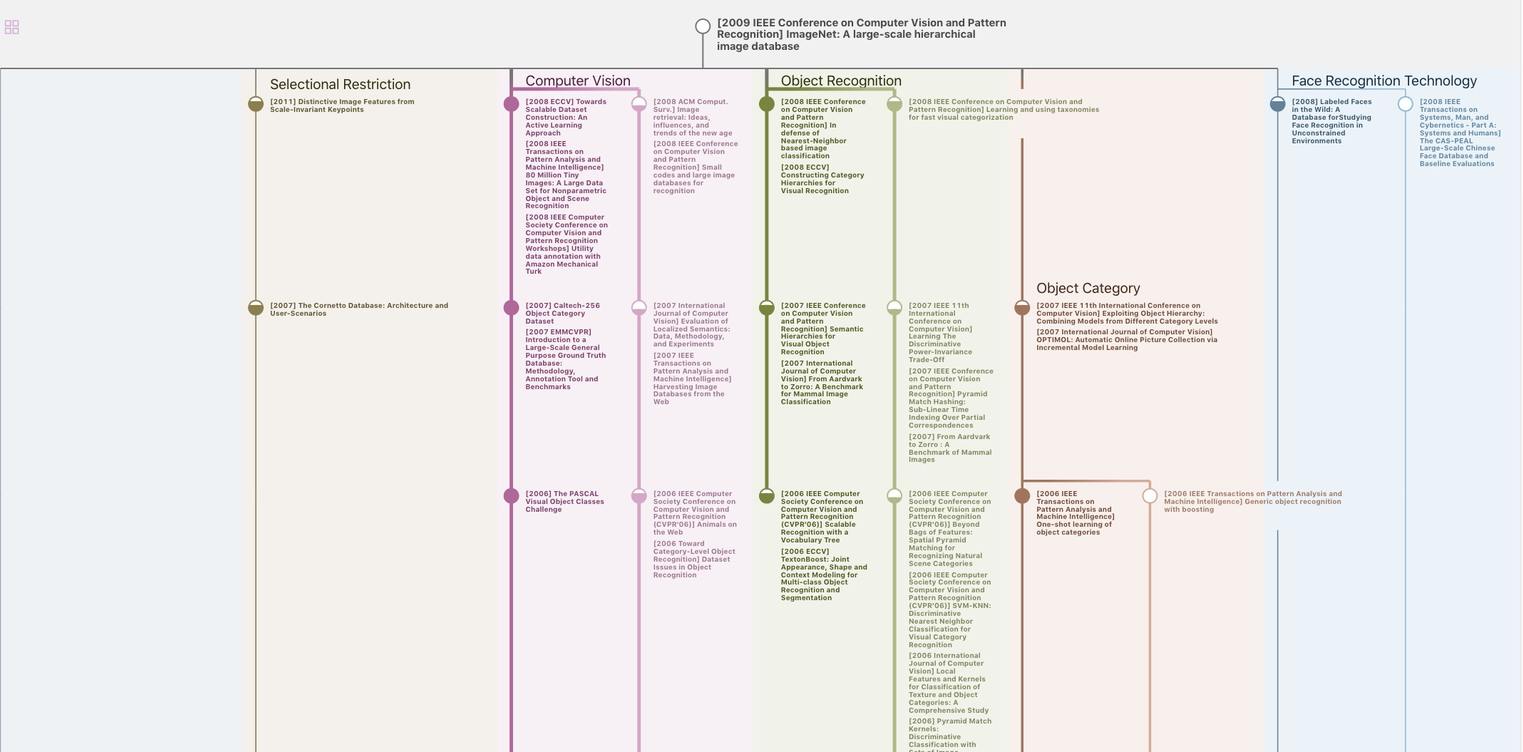
生成溯源树,研究论文发展脉络
Chat Paper
正在生成论文摘要