Stylized Offline Reinforcement Learning: Extracting Diverse High-Quality Behaviors from Heterogeneous Datasets
ICLR 2024(2024)
摘要
Previous literature on policy diversity in reinforcement learning (RL) either focuses on the online setting or ignores the policy performance. In contrast, offline RL, which aims to learn high-quality policies from batched data, has yet to fully leverage the intrinsic diversity of the offline dataset. Addressing this dichotomy and aiming to balance quality and diversity poses a significant challenge to extant methodologies. This paper introduces a novel approach, termed Stylized Offline RL (SORL), which is designed to extract high-performing, stylistically diverse policies from a dataset characterized by distinct behavioral patterns. Drawing inspiration from the venerable Expectation-Maximization (EM) algorithm, SORL innovatively alternates between policy learning and trajectory clustering, a mechanism that promotes policy diversification. To further augment policy performance, we introduce advantage-weighted style learning into the SORL framework. Experimental evaluations across multiple environments demonstrate the significant superiority of SORL over previous methods in extracting high-quality policies with diverse behaviors. A case in point is that SORL successfully learns strong policies with markedly distinct playing patterns from a real-world human dataset of a popular basketball video game "Dunk City Dynasty."
更多查看译文
关键词
Reinforcement Learning,Diversity in RL,Offline RL
AI 理解论文
溯源树
样例
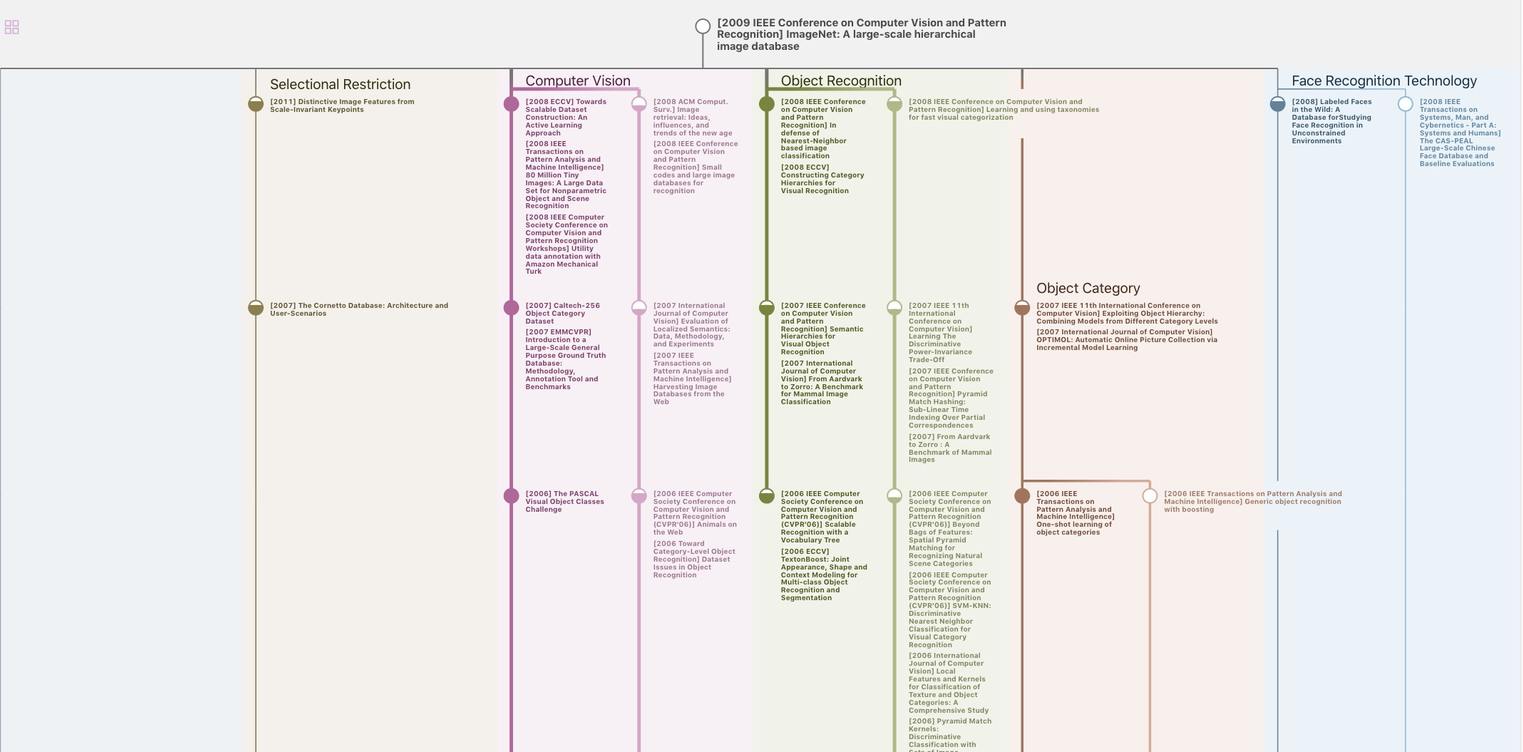
生成溯源树,研究论文发展脉络
Chat Paper
正在生成论文摘要