Adaptive deep spiking neural network with global-local learning via balanced excitatory and inhibitory mechanism
ICLR 2024(2024)
摘要
The training method of Spiking Neural Networks (SNNs) is an essential problem, and how to integrate local and global learning is a worthy research interest. However, the current integration methods do not consider the network conditions suitable for local and global learning, and thus fail to balance their advantages. In this paper, we propose an Excitation-Inhibition Mechanism-assisted Hybrid Learning(EIHL) algorithm that adjusts the network connectivity by using the excitation-inhibition mechanism and then switches between local and global learning according to the network connectivity. The experimental results on CIFAR10/100 and DVS-CIFAR10 demonstrate that the EIHL not only has better accuracy performance than other methods but also has excellent sparsity advantage. Especially, the Spiking VGG11 is trained by EIHL, STBP, and STDP on DVS_CIFAR10, respectively. The accuracy of the Spiking VGG11 model on EIHL is 62.45%, which is 4.35% higher than STBP and 11.40% higher than STDP, and the sparsity is 18.74%, which is 18.74% higher than the other two methods. Moreover, the excitation-inhibition mechanism used in our method also offers a new perspective on the field of SNN learning.
更多查看译文
关键词
Spiking neural networks,excitation inhibition mechanism,Long-Term Potentiation,Long-Term Depression
AI 理解论文
溯源树
样例
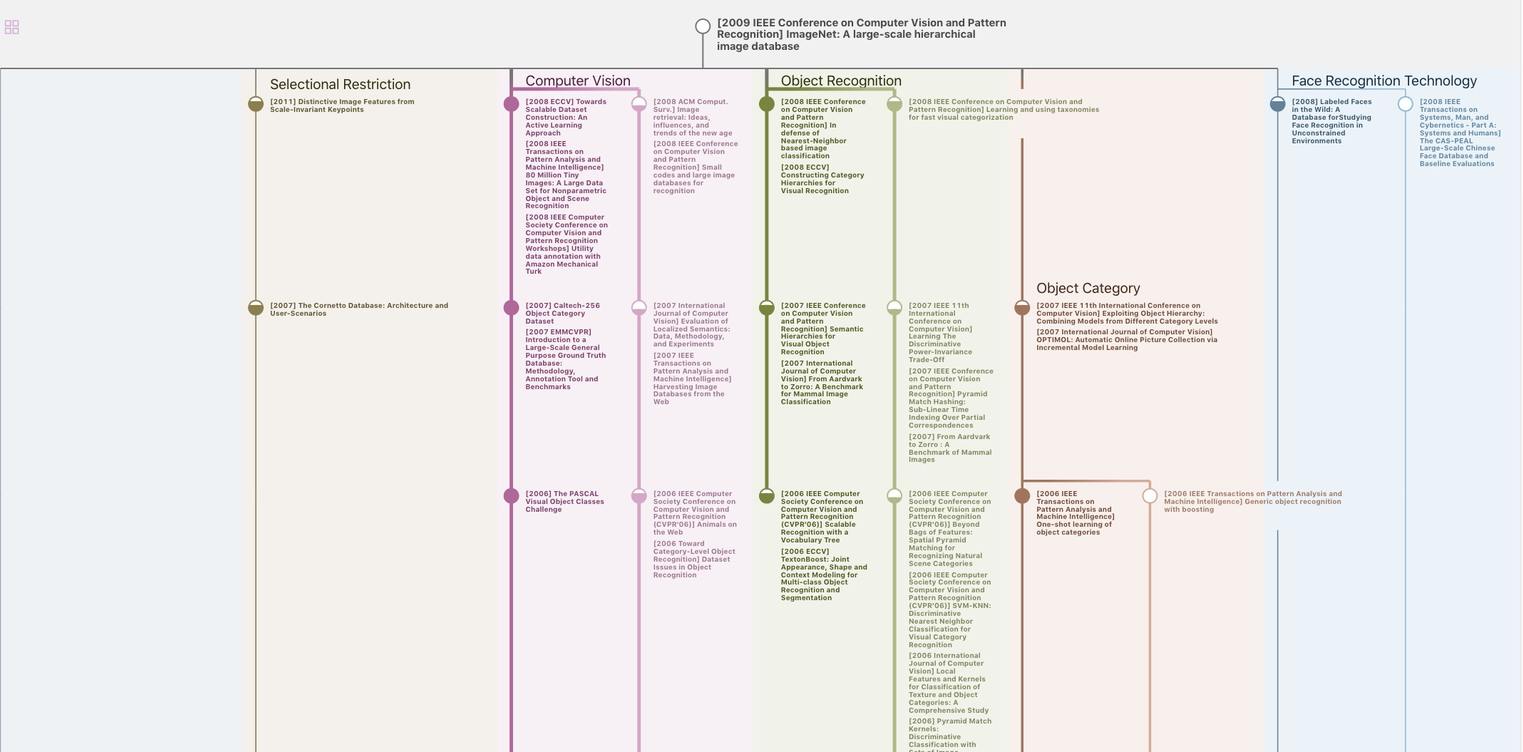
生成溯源树,研究论文发展脉络
Chat Paper
正在生成论文摘要