G$^2$N$^2$ : Weisfeiler and Lehman go grammatical
ICLR 2024(2024)
摘要
This paper introduces a framework for formally establishing a connection between a portion of an algebraic language and a Graph Neural Network (GNN). The framework leverages Context-Free Grammars (CFG) to organize algebraic operations into generative rules that can be translated into a GNN layer model. As CFGs derived directly from a language tend to contain redundancies in their rules and variables, we present a grammar reduction scheme. By applying this strategy, we define a CFG that conforms to the third-order Weisfeiler-Lehman (3-WL) test using the matricial language MATLANG. From this 3-WL CFG, we derive a GNN model, named G$^2$N$^2$, which is provably 3-WL compliant. Through various experiments, we demonstrate the superior efficiency of G$^2$N$^2$ compared to other 3-WL GNNs across numerous downstream tasks. Specifically, one experiment highlights the benefits of grammar reduction within our framework.
更多查看译文
关键词
Machine learning,GNN,Graph Learning
AI 理解论文
溯源树
样例
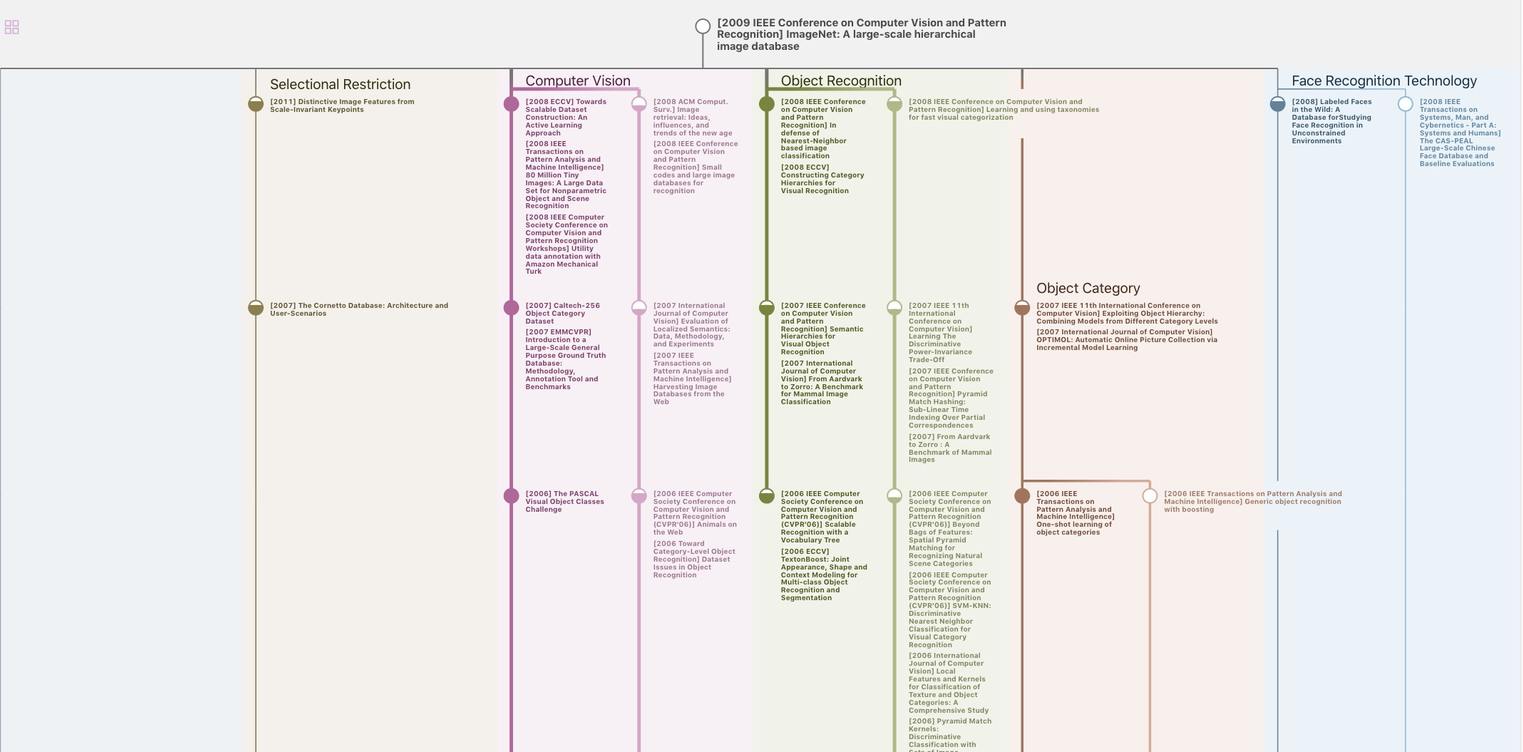
生成溯源树,研究论文发展脉络
Chat Paper
正在生成论文摘要