NeRM: Learning Neural Representations for High-Framerate Human Motion Synthesis
ICLR 2024(2024)
摘要
Generating realistic human motions with high framerate is an underexplored task, due to the varied framerates of training data, huge memory burden brought by high framerates and slow sampling speed of generative models. Recent advances make a compromise for training by downsampling high-framerate details away and discarding low-framerate samples, which suffer from severe information loss and restricted-framerate generation. In this paper, we found that the recent emerging paradigm of Implicit Neural Representations (INRs) that encode a signal into a continuous function can effectively tackle this challenging problem. To this end, we introduce NeRM, a generative model capable of taking advantage of varied-size data and capturing variational distribution of motions for high-framerate motion synthesis. By optimizing latent representation and a auto-decoder conditioned on temporal coordinates, NeRM learns continuous motion fields of sampled motion clips that ingeniously avoid explicit modeling of raw varied-size motions. This expressive latent representation is then used to learn a diffusion model that enables both unconditional and conditional generation of human motions. We demonstrate that our approach achieves competitive results with state-of-the-art methods, and can generate arbitrary framerate motions. Additionally, we show that NeRM is not only memory-friendly, but also highly efficient even when generating high-framerate motions.
更多查看译文
关键词
Human motion synthesis,implicit neural representation,diffusion model,any-framerate training
AI 理解论文
溯源树
样例
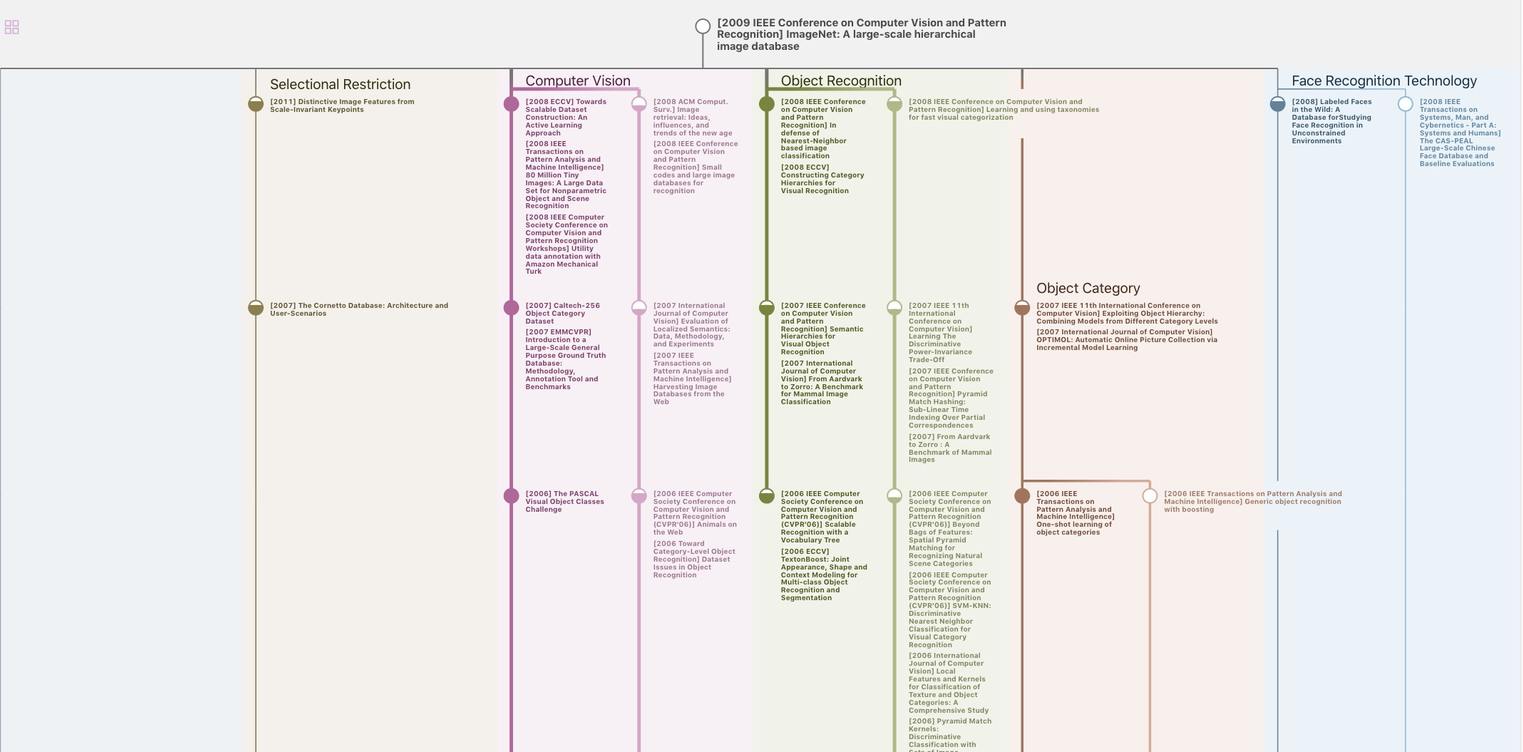
生成溯源树,研究论文发展脉络
Chat Paper
正在生成论文摘要