Plug-and-Play: An Efficient Post-training Pruning Method for Large Language Models
ICLR 2024(2024)
摘要
With the rapid growth of large language models (LLMs), there is increasing demand for memory and computation for LLMs. Recent efforts on post-training pruning of LLMs aim to reduce the model size and computation, yet the performance is still sub-optimal.
In this paper, we present a plug-and-play solution for post-training pruning of LLMs.
The proposed solution has two innovative components: 1) **Relative Importance and Activations** (RIA), a new pruning metric that jointly considers the weight and activations efficiently on LLMs; and 2) **Channel Permutation**, a new approach to maximally preserve important weights under N:M sparsity.
The proposed two components can be readily combined to further enhance the N:M structuredly pruned LLMs.
Our empirical experiments show that RIA alone can already surpass all existing post-training pruning methods on prevalent LLMs, e.g., LLaMA ranging from 7B to 65B. Furthermore, N:M structured pruning with channel permutation can even outperform the original LLaMA2 70B on zero-shot tasks, together with practical speed-up on specific hardware.
更多查看译文
关键词
Post-Training Pruning,Combinatorial Optimization,Large Language Models,Inference Acceleration
AI 理解论文
溯源树
样例
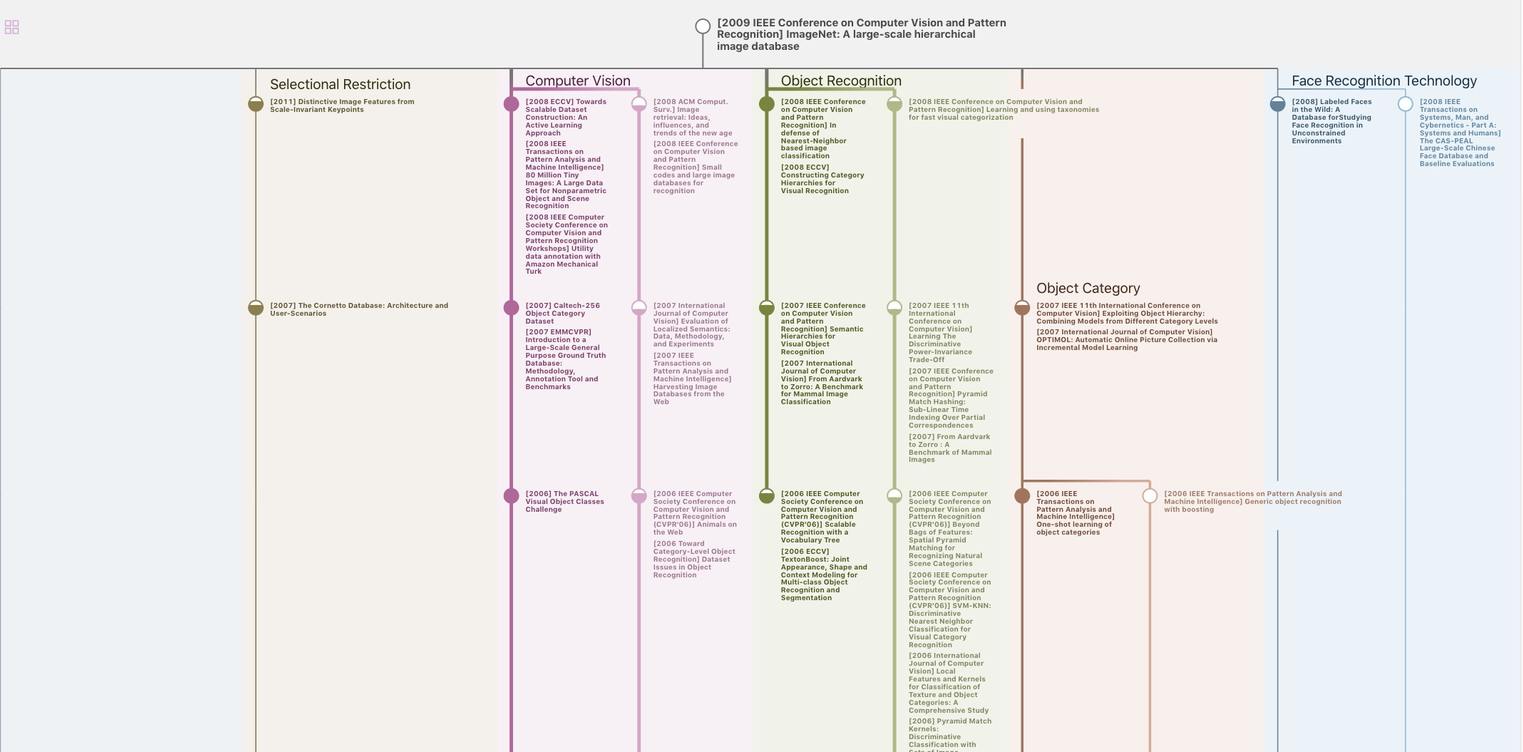
生成溯源树,研究论文发展脉络
Chat Paper
正在生成论文摘要