Unsupervised Classification for Multilook Polarimetric SAR Images via Double Dirichlet Process Mixture Model
IEEE Transactions on Geoscience and Remote Sensing(2024)
摘要
This paper proposes a hierarchical double Dirichlet process mixture model (DDPMM) for multilook polarimetric synthetic aperture radar (PolSAR) data unsupervised classification. Specifically, within the framework of product model (PM), an observed PolSAR data point can be factorized as the multiplication representation of a positive-scalar texture variable and a complex-Wishart-distributed speckle component. Based on this assumption, the polarization DPMM and texture DPMM in the proposed model are hierarchically established to characterize the polarimetric matrix and texture variable, respectively, thus yielding the generation procedure of the observation data to be learned sufficiently. Meanwhile, instead of sharing the same texture vector in many existing PM-based methods, each data point in DDPMM is associated with its own texture vector, which can be characterized as the weighted summation of several densities via texture DPMM rather than following a single distribution, such that the texture information can be fully and flexibly captured. In particular, dual local spatial constraints based on the statistical representations of polarization and texture spaces are also explored on the two DPMMs, allowing the local correlation to be adequately and dynamically incorporated. Moreover, all closed-form updates are derived with the variational Bayesian inference algorithm and the cluster number of the proposed model can be determined automatically. Experimental results on four real PolSAR datasets demonstrate the superiority of the proposed DDPMM to some state-of-the-art methods.
更多查看译文
关键词
Polarimetric synthetic aperture radar,variational Bayesian inference,unsupervised classification,Dirichlet process mixture model,remote sensing technology
AI 理解论文
溯源树
样例
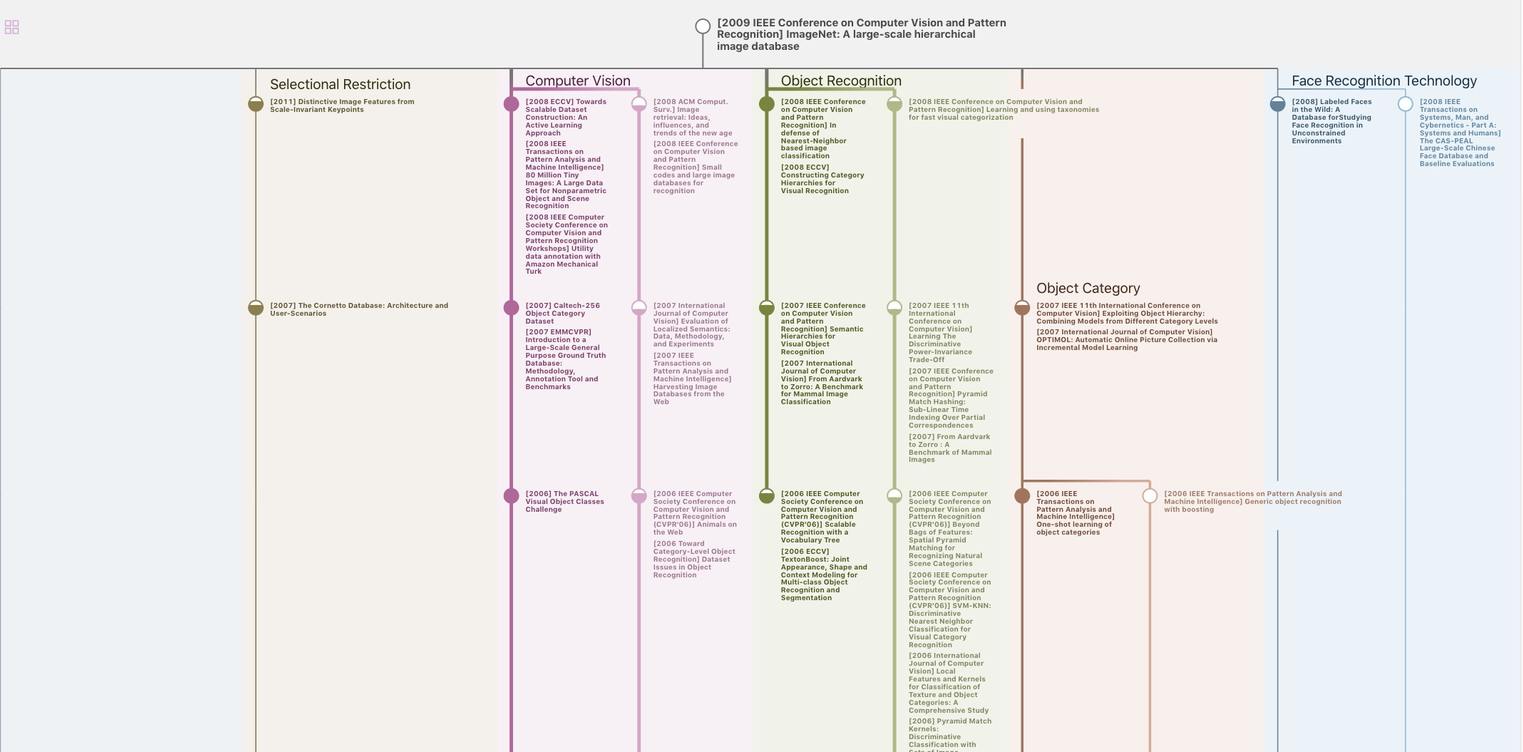
生成溯源树,研究论文发展脉络
Chat Paper
正在生成论文摘要