Training-free Multi-objective Diffusion Model for 3D Molecule Generation
ICLR 2024(2024)
摘要
Searching for novel and diverse molecular candidates is a critical undertaking in drug and material discovery. Existing approaches have successfully adapted the diffusion model, the most effective generative model in image generation, to create 1D SMILES strings, 2D chemical graphs, or 3D molecular conformers. However, these methods are not efficient and flexible enough to generate 3D molecules with multiple desired properties, as they require additional training for the models for each new property or even a new combination of existing properties. Moreover, some properties may potentially conflict, making it impossible to find a molecule that satisfies all of them simultaneously. To address these challenges, we present a training-free conditional 3D molecular generation algorithm based on off-the-shelf unconditional diffusion models and property prediction models. The key techniques include modeling the loss of property prediction models as energy functions, considering the property relation between multiple conditions as a probabilistic graph, and developing a stable posterior estimation for computing the conditional score function. We conducted experiments on both single-objective and multi-objective 3D molecule generation, focusing on quantum properties, and compared our approach with the trained or fine-tuned diffusion models. Our proposed model achieves superior performance in generating molecules that meet the conditions, without any additional training cost.
更多查看译文
关键词
Multi-objective Diffusion Model,3D Molecule
AI 理解论文
溯源树
样例
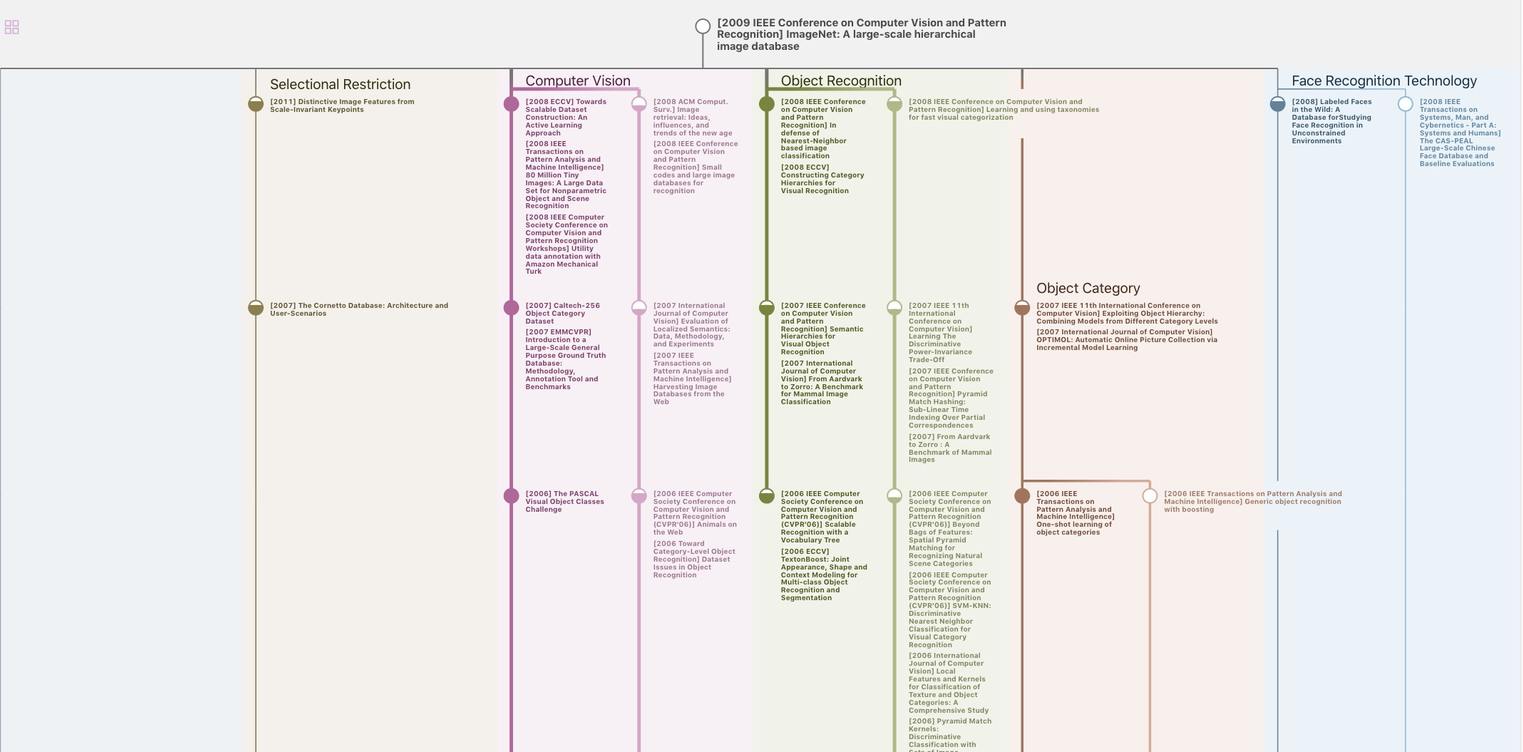
生成溯源树,研究论文发展脉络
Chat Paper
正在生成论文摘要