Improving the Convergence of Dynamic NeRFs via Optimal Transport
ICLR 2024(2024)
摘要
Synthesizing novel views for dynamic scenes from a collection of RGB inputs poses significant challenges due to the inherent under-constrained nature of the problem. To mitigate this ill-posedness, practitioners in the field of neural radiance fields (NeRF) often resort to the adoption of intricate geometric regularization techniques, including scene flow, depth estimation, or learned perceptual similarity. While these geometric cues have demonstrated their effectiveness, their incorporation leads to evaluation of computationally expensive off-the-shelf models, introducing substantial computational overhead into the pipeline. Moreover, seamlessly integrating such modules into diverse dynamic NeRF models can be a non-trivial task, hindering their utilization in an architecture-agnostic manner. In this paper, we propose a theoretically grounded, lightweight regularizer by treating the dynamics of a time-varying scene as a low-frequency change of a probability distribution of the light intensity. We constrain the dynamics of this distribution using optimal transport (OT) and provide error bounds under reasonable assumptions. Our regularization is learning-free, architecture agnostic, and can be implemented with just a few lines of code. Finally, we demonstrate the practical efficacy of our regularizer across state-of-the-art architectures.
更多查看译文
关键词
Dynamic NeRF,Optimal transport
AI 理解论文
溯源树
样例
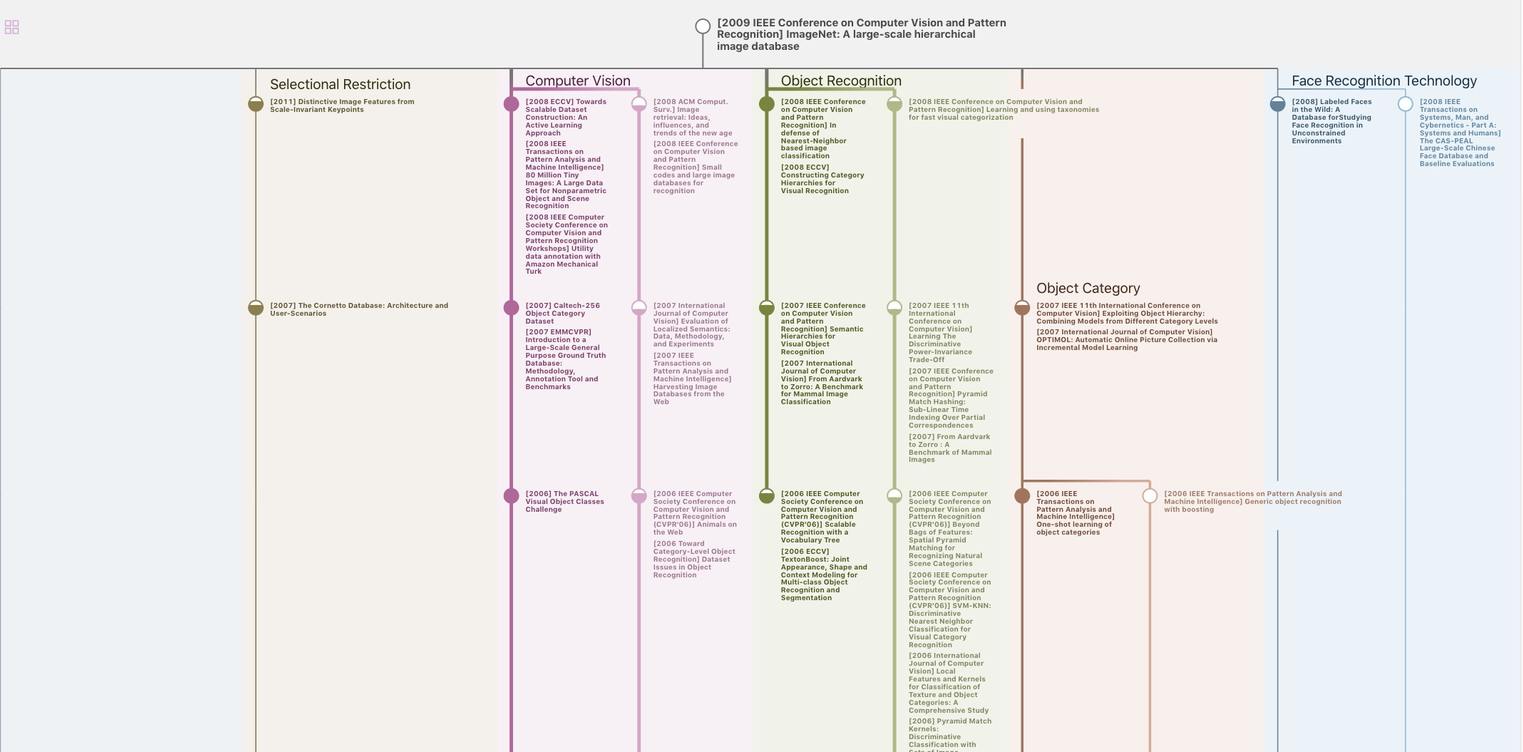
生成溯源树,研究论文发展脉络
Chat Paper
正在生成论文摘要