MAMBA: an Effective World Model Approach for Meta-Reinforcement Learning
ICLR 2024(2024)
摘要
Meta-reinforcement learning (meta-RL) is a promising framework for tackling
challenging domains requiring efficient exploration. Existing meta-RL
algorithms are characterized by low sample efficiency, and mostly focus on
low-dimensional task distributions. In parallel, model-based RL methods have
been successful in solving partially observable MDPs, of which meta-RL is a
special case. In this work, we leverage this success and propose a new
model-based approach to meta-RL, based on elements from existing
state-of-the-art model-based and meta-RL methods. We demonstrate the
effectiveness of our approach on common meta-RL benchmark domains, attaining
greater return with better sample efficiency (up to 15×) while requiring
very little hyperparameter tuning. In addition, we validate our approach on a
slate of more challenging, higher-dimensional domains, taking a step towards
real-world generalizing agents.
更多查看译文
关键词
Meta Reinforcement Learning,World Models,Model Based Reinforcement Learning
AI 理解论文
溯源树
样例
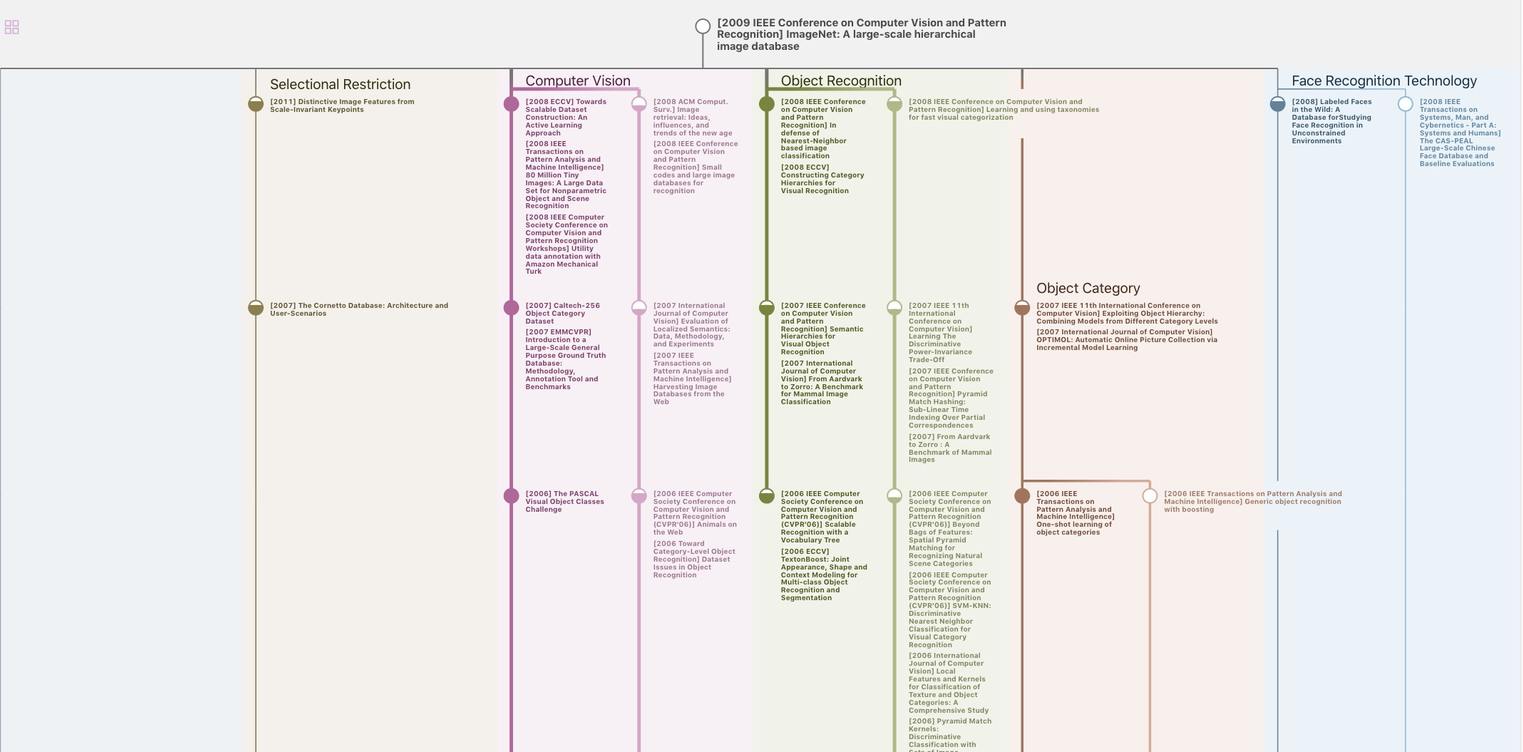
生成溯源树,研究论文发展脉络
Chat Paper
正在生成论文摘要