C-TPT: Calibrated Test-Time Prompt Tuning for Vision-Language Models via Text Feature Dispersion
ICLR 2024(2024)
摘要
In deep learning, test-time adaptation has gained attention as a method for
model fine-tuning without the need for labeled data. A prime exemplification is
the recently proposed test-time prompt tuning for large-scale vision-language
models such as CLIP. Unfortunately, these prompts have been mainly developed to
improve accuracy, overlooking the importance of calibration-a crucial aspect
for quantifying prediction uncertainty. However, traditional calibration
methods rely on substantial amounts of labeled data, making them impractical
for test-time scenarios. To this end, this paper explores calibration during
test-time prompt tuning by leveraging the inherent properties of CLIP. Through
a series of observations, we find that the prompt choice significantly affects
the calibration in CLIP, where the prompts leading to higher text feature
dispersion result in better-calibrated predictions. Introducing the Average
Text Feature Dispersion (ATFD), we establish its relationship with calibration
error and present a novel method, Calibrated Test-time Prompt Tuning (C-TPT),
for optimizing prompts during test-time with enhanced calibration. Through
extensive experiments on different CLIP architectures and datasets, we show
that C-TPT can effectively improve the calibration of test-time prompt tuning
without needing labeled data.
更多查看译文
关键词
Calibration,Test-time adaptation,Foundation model,Prompt tuning
AI 理解论文
溯源树
样例
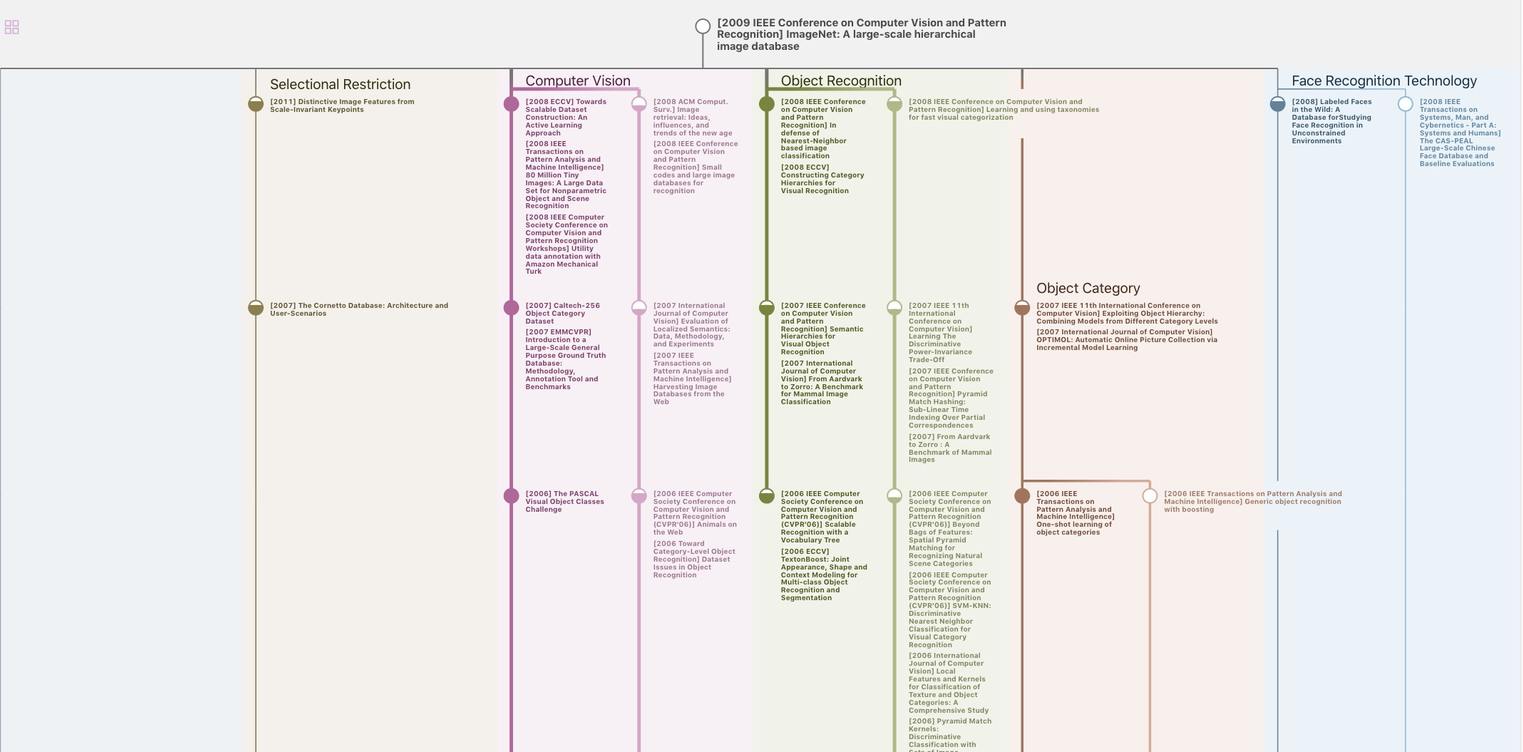
生成溯源树,研究论文发展脉络
Chat Paper
正在生成论文摘要