PROGRAM: PROtotype GRAph Model based Pseudo-Label Learning for Test-Time Adaptation
ICLR 2024(2024)
摘要
Test-time adaptation (TTA) aims to adapt a pre-trained model from a source domain to a target domain only using online unlabeled target data during testing, without accessing to the source data or modifying the original training process. Among the various TTA methods, pseudo-labeling has gained popularity. However, the presence of incorrect pseudo-labels can hinder the effectiveness of target domain adaptation. To overcome this challenge, we propose a novel TTA method, called PROtotype GRAph Model based pseudo-label learning (PROGRAM). PROGRAM consists of two key components: (1) Prototype Graph Model (PGM) for reliable pseudo-label generation; (2) Robust Self-Training (RST) for test-time adaptation with noisy pseudo-labels. PGM constructs the graph using prototypes and test samples, facilitating effective message passing among them to generate more reliable pseudo-labels. RST combines the advantages of consistency regularization and pseudo-labeling to achieve robust target domain adaptation in the presence of noisy pseudo-labels. Our proposed PROGRAM can be easily integrated into existing baselines, resulting in consistent improvement. Extensive experiments show that our PROGRAM outperforms the existing TTA methods on multiple domain generalization and image corruption benchmarks.
更多查看译文
关键词
test-time adaptation,domain adaptation,domain shift
AI 理解论文
溯源树
样例
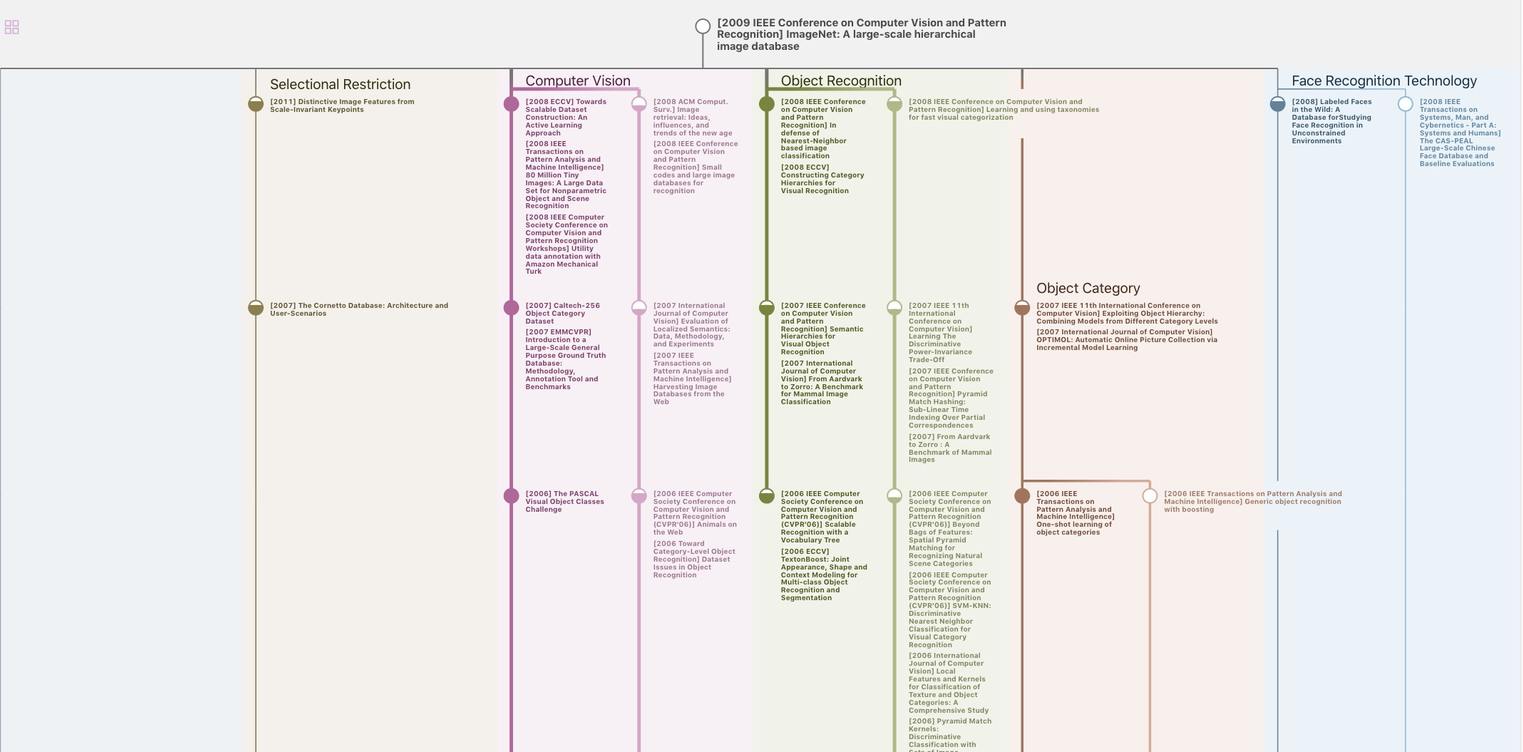
生成溯源树,研究论文发展脉络
Chat Paper
正在生成论文摘要