Latent Intuitive Physics: Learning to Transfer Hidden Physics from A 3D Video
ICLR 2024(2024)
摘要
We introduce latent intuitive physics, a transfer learning framework for physics simulation that can infer hidden properties of fluids from a single 3D video and simulate the observed fluid in novel scenes. Our key insight is to use latent features drawn from a learnable prior distribution conditioned on the underlying particle states to capture the invisible and complex physical properties. To achieve this, we train a parametrized prior learner given visual observations to approximate the visual posterior of inverse graphics, and both the particle states and the visual posterior are obtained from a learned neural renderer. The converged prior learner is embedded in our probabilistic physics engine, allowing us to perform novel simulations on unseen geometries, boundaries, and dynamics without knowledge of the true physical parameters. We validate our model in three ways: (i) novel scene simulation with the learned visual-world physics, (ii) future prediction of the observed fluid dynamics, and (iii) supervised particle simulation. Our model demonstrates strong performance in all three tasks.
更多查看译文
关键词
Intuitive physics,inverse graphics,particle-based fluid simulation,neural rendering
AI 理解论文
溯源树
样例
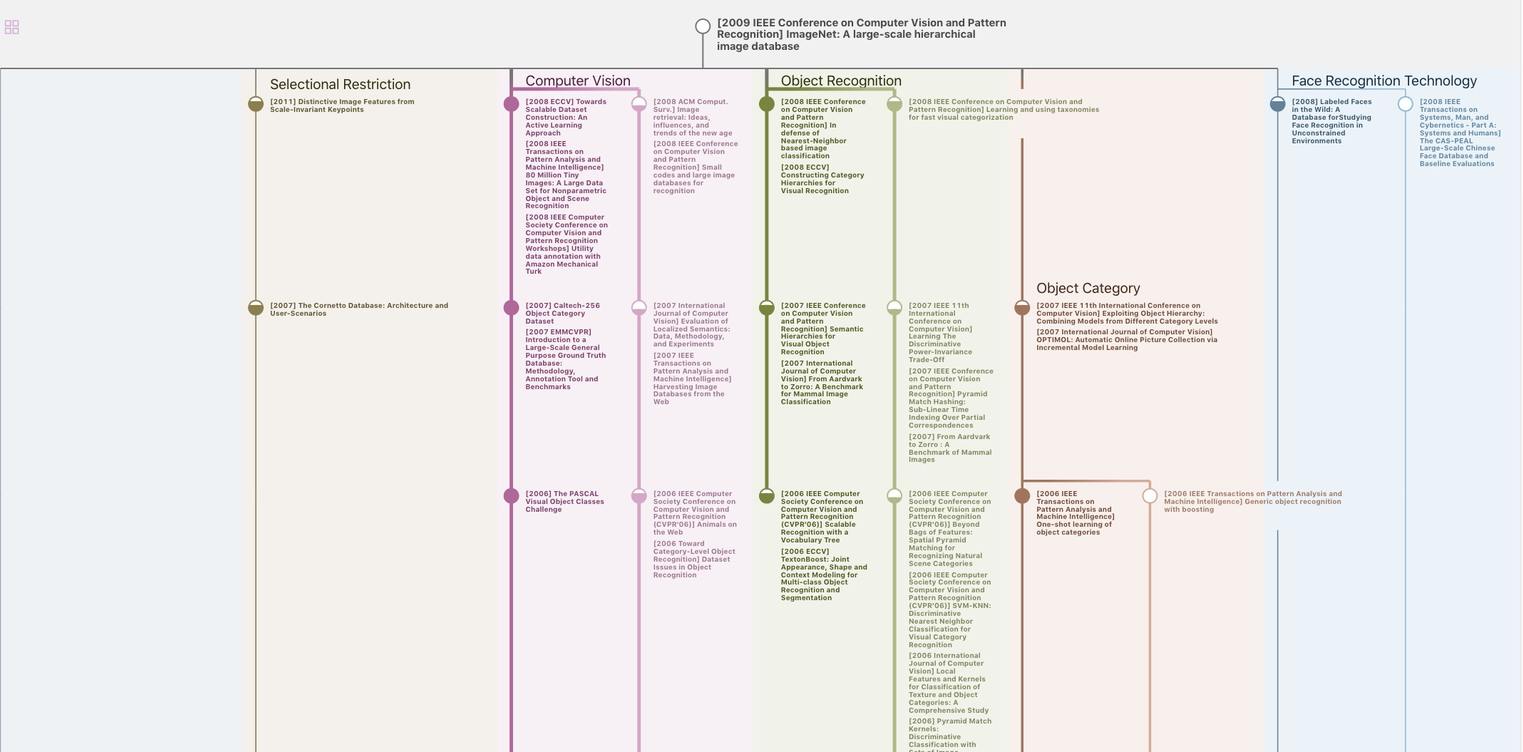
生成溯源树,研究论文发展脉络
Chat Paper
正在生成论文摘要