Cauchy-Schwarz Divergence Information Bottleneck for Regression
ICLR 2024(2024)
摘要
The information bottleneck (IB) approach is popular to improve the generalization, robustness and explainability of deep neural networks. Essentially, it aims to find a minimum sufficient representation $\mathbf{t}$ by striking a trade-off between a compression term, which is usually characterized by mutual information $I(\mathbf{x};\mathbf{t})$ where $\mathbf{x}$ refers to the input, and a prediction term usually characterized by $I(y;\mathbf{t})$ where $y$ is the desired response. Mutual information is for the IB for the most part expressed in terms of the Kullback-Leibler (KL) divergence, which in the regression case corresponds to prediction based on mean squared error (MSE) loss with Gaussian assumption and compression approximated by variational inference.
In this paper, we study the IB principle for the regression problem and develop a new way to parameterize the IB with deep neural networks by exploiting favorable properties of the Cauchy-Schwarz (CS) divergence. By doing so, we move away from MSE-based regression and ease estimation by avoiding variational approximations or distributional assumptions. We investigate the improved generalization ability of our proposed CS-IB and demonstrate strong adversarial robustness guarantees. We demonstrate its superior performance on six real-world regression tasks over other popular deep IB approaches. We additionally observe that the solutions discovered by CS-IB always achieve the best trade-off between prediction accuracy and compression ratio in the information plane.
更多查看译文
关键词
Information Bottleneck,Cauchy-Schwarz Divergence,Regression
AI 理解论文
溯源树
样例
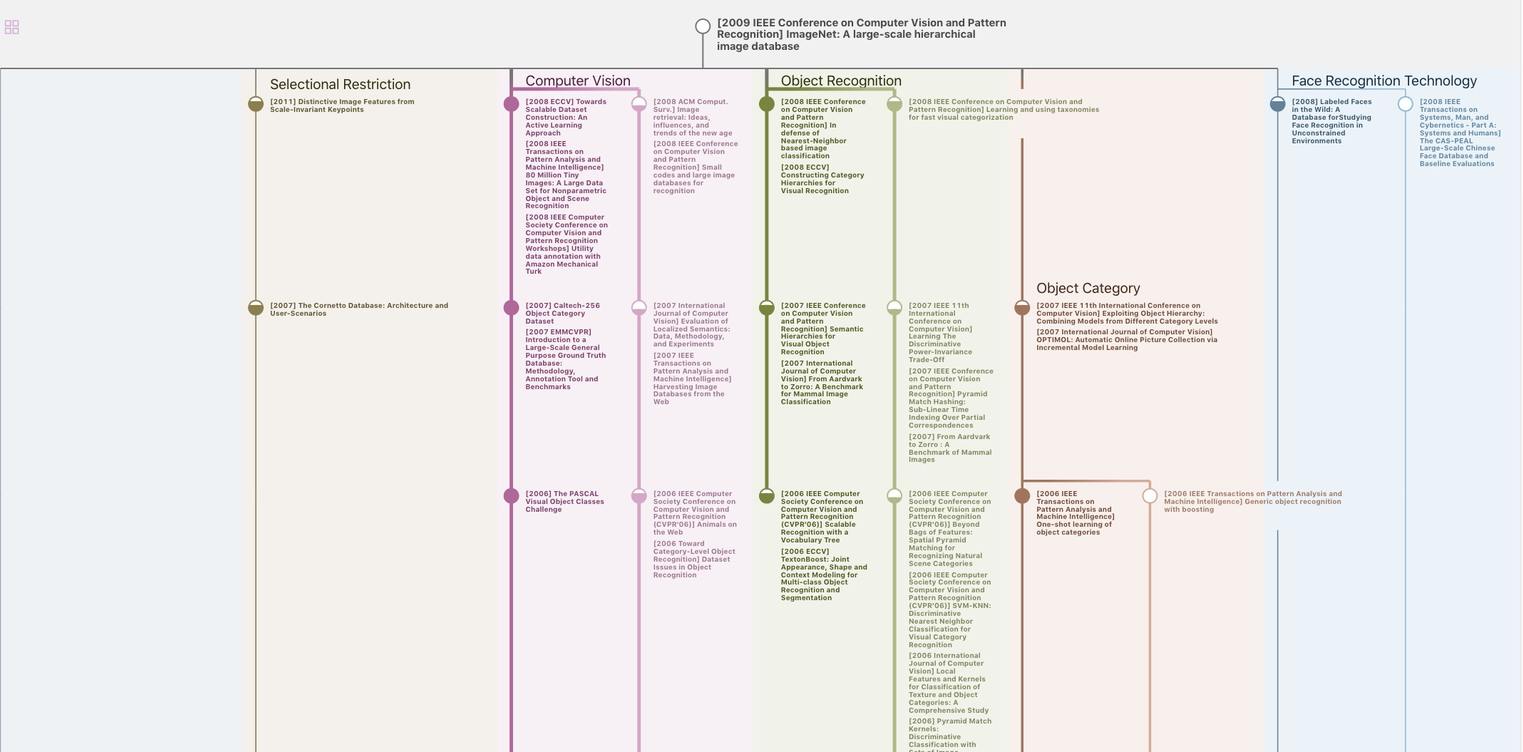
生成溯源树,研究论文发展脉络
Chat Paper
正在生成论文摘要