Bounding the Expected Robustness of Graph Neural Networks Subject to Node Feature Attacks
ICLR 2024(2024)
摘要
Graph Neural Networks (GNNs) have demonstrated state-of-the-art performance in various graph representation learning tasks. Recently, studies revealed their vulnerability to adversarial attacks. In this work, we theoretically define the concept of expected robustness in the context of attributed graphs and relate it to the classical definition of adversarial robustness in the graph representation learning literature. Our definition allows us to derive an upper bound of the expected robustness of Graph Convolutional Networks (GCNs) and Graph Isomorphism Networks subject to node feature attacks. Building on these findings, we connect the expected robustness of GNNs to the orthogonality of their weight matrices and consequently propose an attack-independent, more robust variant of the GCN, called the Graph Convolutional Orthogonal Robust Networks (GCORNs). We further introduce a probabilistic method to estimate the expected robustness, which allows us to evaluate the effectiveness of GCORN on several real-world datasets. Experimental experiments showed that GCORN outperforms available defense methods.
更多查看译文
关键词
Graph Neural Networks,Adversarial Robustness
AI 理解论文
溯源树
样例
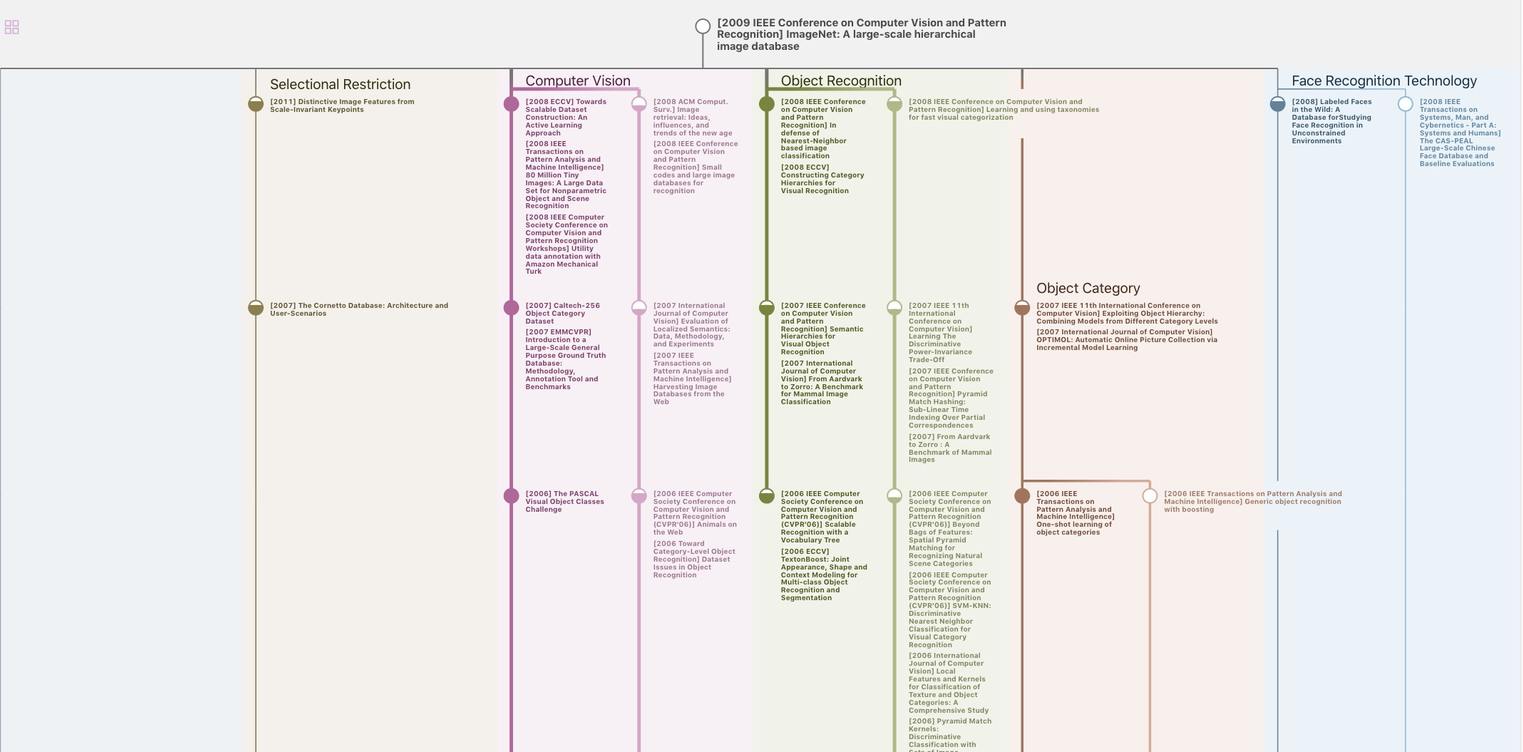
生成溯源树,研究论文发展脉络
Chat Paper
正在生成论文摘要