Causal Structure Recovery with Latent Variables under Milder Distributional and Graphical Assumptions
ICLR 2024(2024)
摘要
Traditional causal discovery approaches typically assume the absence of latent variables, a simplification that often does not align with real-world situations. Recently, there has been a surge of causal discovery methods that explicitly consider latent variables. While some works aim to reveal causal relations between observed variables in the presence of latent variables, others seek to identify latent variables and recover the causal structure over them. The latter typically entail strong distributional and graphical assumptions, such as the non-Gaussianity, purity, and two-pure-children assumption. In this paper, we endeavor to recover the whole causal structure involving both latent and observed variables under milder assumptions. We formulate two cases, one allows entirely arbitrary distribution and requires only one pure child per latent variable, and the other requires no pure child and imposes the non-Gaussianity requirement on only a subset of variables, and they both avoid the purity assumption. We prove the identifiability of linear latent variable models in both cases, and our constructive proof leads to theoretically sound and computationally efficient algorithms.
更多查看译文
关键词
causal discovery,latent variables
AI 理解论文
溯源树
样例
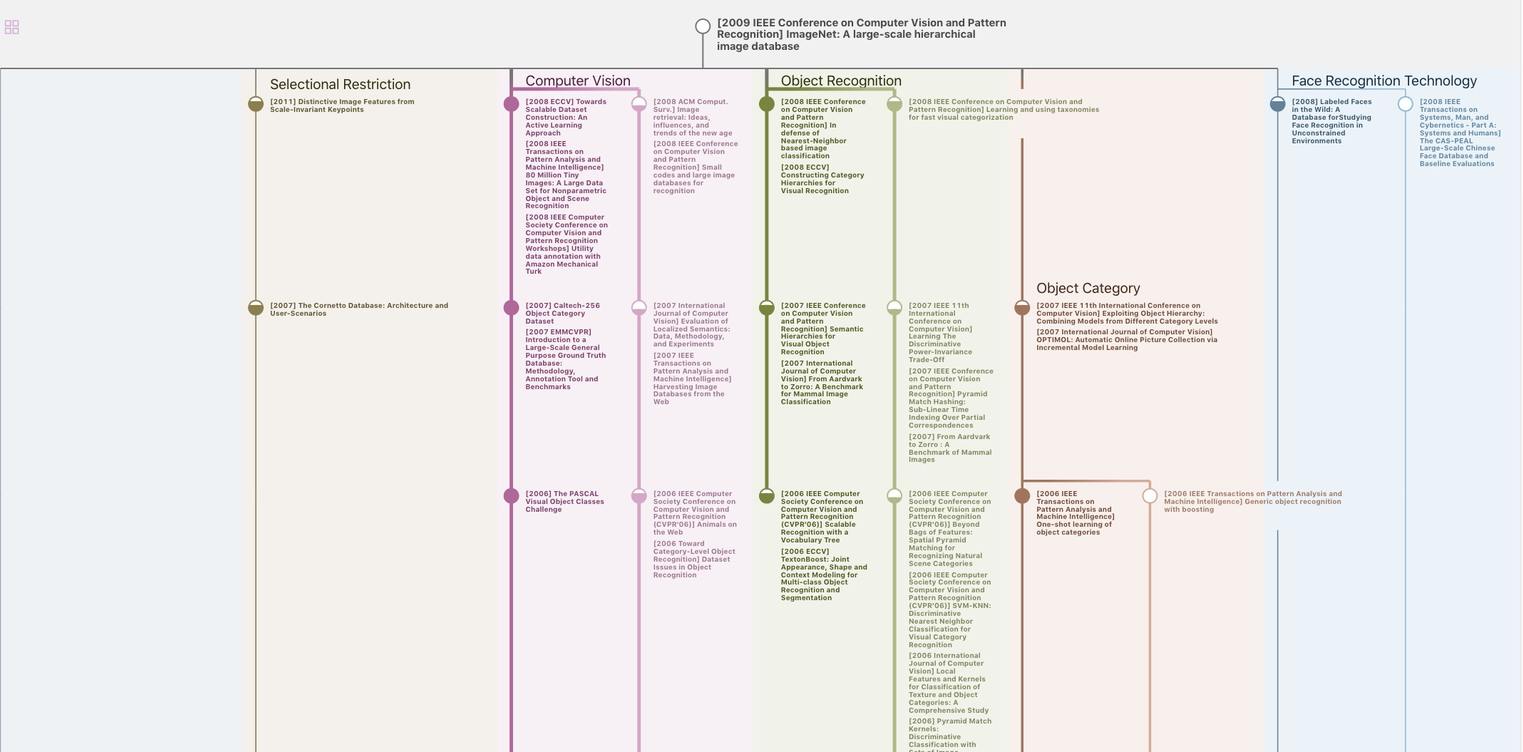
生成溯源树,研究论文发展脉络
Chat Paper
正在生成论文摘要