Random Sparse Lifts: Construction, Analysis and Convergence of finite sparse networks
ICLR 2024(2024)
摘要
We present a framework to define a large class of neural networks for which, by construction, training by gradient flow provably reaches arbitrarily low loss when the number of parameters grows. Distinct from the fixed-space global optimality of non-convex optimization, this new form of convergence, and the techniques introduced to prove such convergence, pave the way for a usable deep learning convergence theory in the near future, without overparameterization assumptions relating the number of parameters and training samples. We define these architectures from a simple computation graph and a mechanism to lift it, thus increasing the number of parameters, generalizing the idea of increasing the widths of multi-layer perceptrons. We show that architectures similar to most common deep learning models are present in this class, obtained by sparsifying the weight tensors of usual architectures at initialization. Leveraging tools of algebraic topology and random graph theory, we use the computation graph’s geometry to propagate properties guaranteeing convergence to any precision for these large sparse models.
更多查看译文
关键词
Neural networks,theory of deep learning,convergence guarantees,random graphs,algebraic topology
AI 理解论文
溯源树
样例
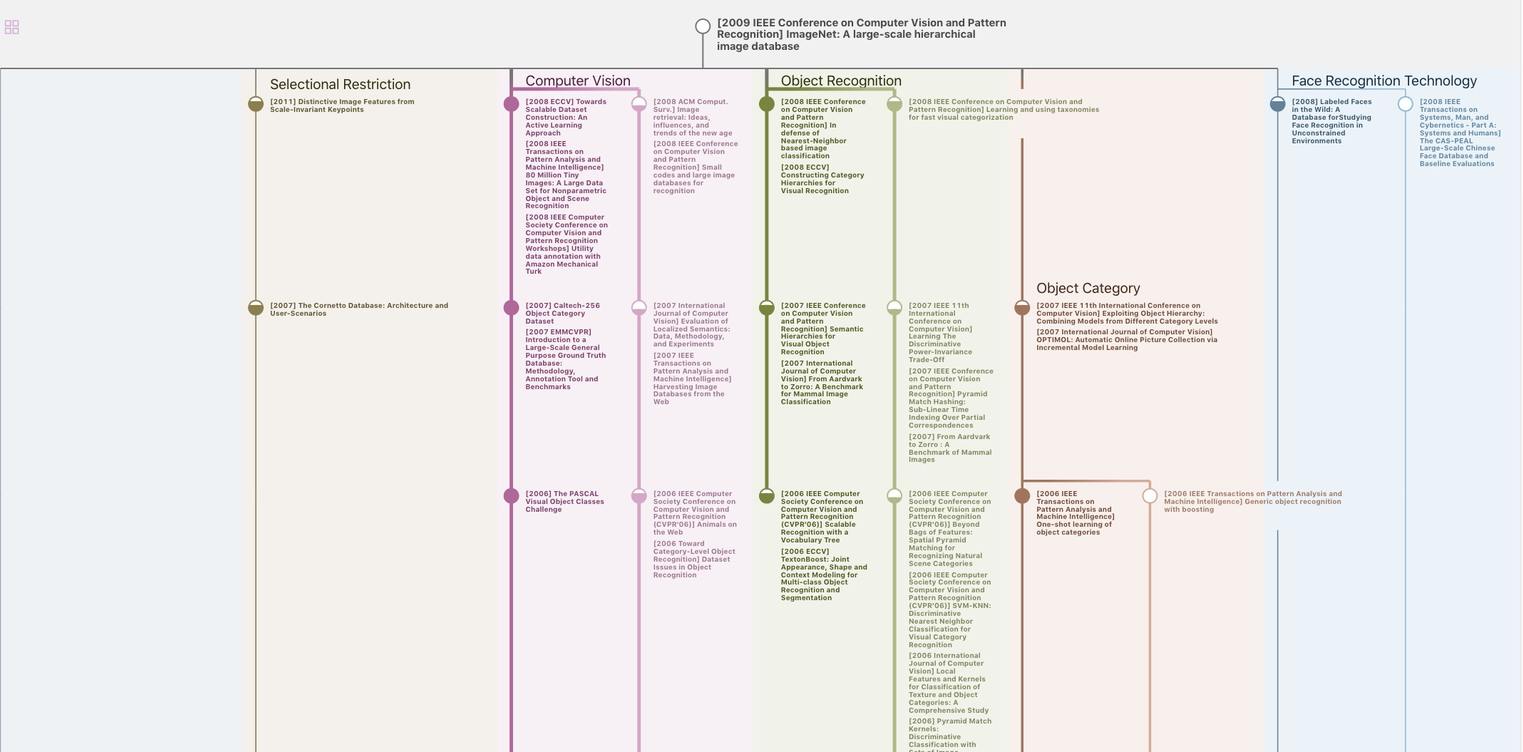
生成溯源树,研究论文发展脉络
Chat Paper
正在生成论文摘要