Evaluating Language Models Through Negotiations
ICLR 2024(2024)
摘要
Commercial interests are racing to exploit language models' remarkable capability to display agent-like behavior.
Indeed, a future where personal LM-based agents are widely adopted to perform complicated tasks involving planning and negotiating appears increasingly plausible. Current, predominantly static evaluation methods are ill-suited to evaluate such dynamic, multi-step applications. In this work, we therefore propose jointly evaluating LM performance and alignment through the lens of negotiation games.
We argue that this common real-world task provides scalable, difficult-to-hack performance metrics while offering non-trivial insights into model decision-making. Crucially, negotiation games allow us to study both competitive and cooperative performance, modulate complexity, and side-step accidental evaluation data leakage. Using our evaluation setup, we report results for publicly accessible LMs from all major providers on a variety of negotiation games. Noteworthy takeaways include: (i) open-source models are currently unable to complete this task, (ii) cooperative bargaining games prove challenging, and (iii) the most powerful models do not always 'win'.
Evaluation through negotiations complements existing evaluation efforts by providing a novel evaluation paradigm to study evolving language model agency. We release an open-source library to accelerate research in this critical direction and lower the technical boundaries for researchers outside of the machine learning field to contribute.
更多查看译文
关键词
language model evaluation,dynamic evaluation,alignment,cooperative AI,agency
AI 理解论文
溯源树
样例
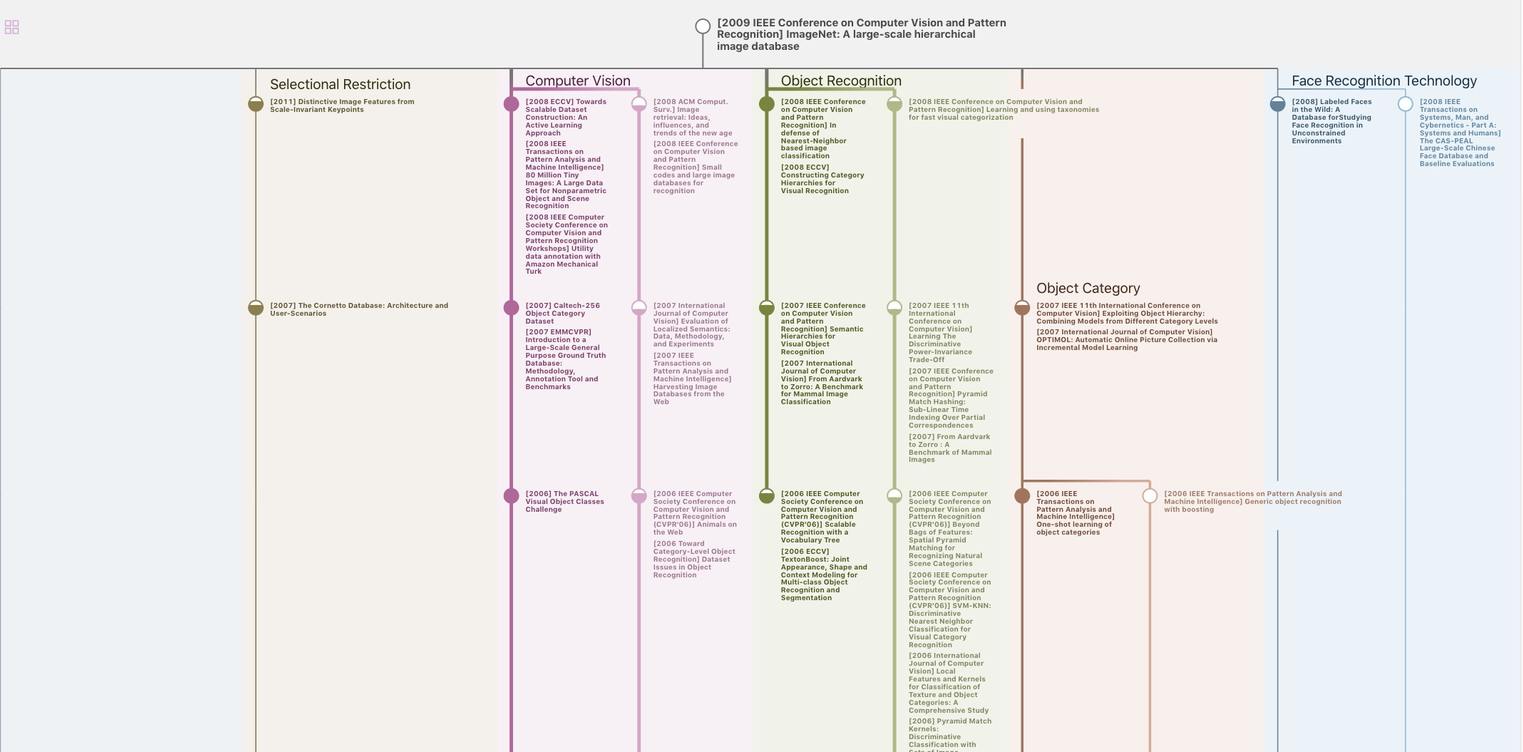
生成溯源树,研究论文发展脉络
Chat Paper
正在生成论文摘要